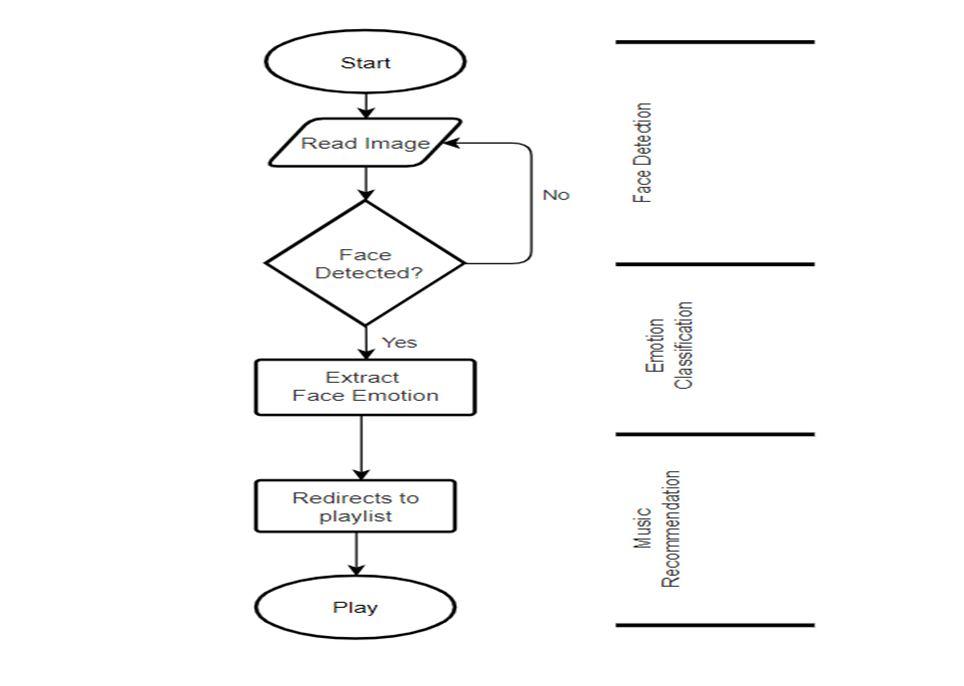
5 minute read
Web Application for Emotion-Based Music Player using Streamlit
Dr. J Naga Padmaja1 , Amula Vijay Kanth2, P Vamshidhar Reddy3, B Abhinay Rao4
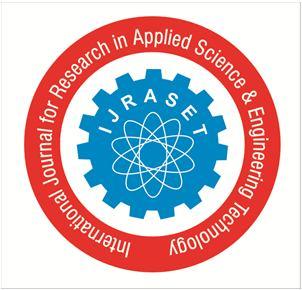
Advertisement
1Assistant Professor,
2, 3, 4
UG Student, Dept of Computer Science and Engineering, Vardhaman College of Engineering, Hyderabad
Abstract: Music is a language that doesn’t speak in particular words, it speaks in emotions. Music has certain qualities or properties that impact our feelings. We've numerous music recommendation systems that recommend music based on the previous search history, previous listening history, or ratings provided by users. Our project is all about developing a recommendation system that recommends music based on the user’s current mood. This approach is more effective than the existing ones and eases user’s work of first searching and creating a specific playlist. A user’s emotion or mood can be identified by his/her facial expressions. These expressions can be deduced from the live feed via the system’s camera. Machine Learning come up with various methods and techniques through which human emotions can be detected. Support Vector Machine (SVM) algorithm is used to develop this system. The SVM is used as a classifier to classify different emotional states such as happy, sad, etc. The facial feature displacements in the live feed are used as input to the SVM classifier. Based on the output of the SVM classifier, the user is redirected to a corresponding playlist. Streamlit Framework is used to build this web application. It helps us to create beautiful web apps for data science and machine learning in less time.
Keywords: Machine Learning; Support Vector Machine; Streamlit Framework; Recommendation System;
I. INTRODUCTION
Numerous studies in recent times reveal that humans respond and reply to music and this music has a high impression on the exertion of the mortal brain. Music is the form of art known to have a major connection with a person's emotion. Music can also be considered as the universal language of humanity. It has the ability to bring positivity and refreshment in the lives of individuals. Experimenters discovered that music plays a major part in relating thrill and mood. Two of the most important functions of music are its unique capability to lift up one's mood and come more tone- apprehensive. Musical preferences have been demonstrated to be largely affiliated to personality traits and moods. Facial expressions are the best way that people may derive or estimate the emotion, sentiment, or reflections that another person is trying to express. People frequently use their facial expressions to communicate their feelings. It has long been recognized that music may alter a person's nature. A user's mood can be gradually calmed down and an overall good effect can be produced by capturing and recognizing the emotion being emitted by the person and playing appropriate songs corresponding to the one's mood. Music is a great way to express emotions and moods. For example, people like to hear to happysongs when theyare feeling good, a comforting song can support us to relax when we are feeling stressed-out and people tend to hear some kind of sad songs when they are feeling down. Hence in this project, we're going to develop a system which will capture the real time emotion of the user and based on that emotion, related songs will be recommended. We're going to classify songs into the groups based on the categories like Happy, Sad, Neutral etc. Then according to the captured emotion, the user will be redirected to a suitable playlist. In this way user can get recommendation of songs based on his current mood and will change dynamically based on current mood.
II. RELATED WORK
A. Literature Survey
An important field of research to enhance human-machine interaction is emotion recognition. The task of acquisition is made more challenging by emotion complexity. It is suggested that Quondam works capture emotion through a unimodal mechanism, such as just facial expressions or just voice input. The concept of multimodal emotion identification has lately gained some traction, which has improved the machine's ability to recognise emotions with greater accuracy.
Below are some research papers used for Literature Survey on Facial Emotion Recognition.
1) Jaiswal, A. Krishnama Raju and S. Deb, "Facial Emotion Detection Using Deep Learning," 2020 International Conference for Emerging Technology (INCET), 2020.
In order to detect emotions, these steps are used: face detection, feature extraction, and emotion classification. The suggested method reduces computing time while improving validation accuracy and reducing loss accuracy.
ISSN: 2321-9653; IC Value: 45.98; SJ Impact Factor: 7.538
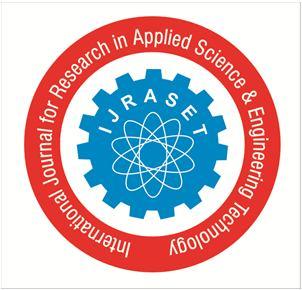
Volume 11 Issue II Feb 2023- Available at www.ijraset.com
It also achieves a further performance evaluation by contrasting this model with an earlier model that was already in use. On the FERC-2013 and JAFFE databases, which comprise seven fundamental emotions like sadness, fear, happiness, anger, neutrality, surprise, and disgust, we tested our neural network architectures. In this research, robust CNN is used to picture identification and use the deep learning (DL) free library "Keras" given by Google for facial emotion detection. This proposed network was trained using two independent datasets, and its validation accuracy and loss accuracy were assessed. Facial expressions are used for seven different emotions in images taken from a specific dataset to detect expressions using a CNN-created emotion model. Keras library provided by Google is used to update a few steps in CNN, and also adjusted the CNN architecture to provide improved accuracy.
2) Kharat, G. U., and S. V. Dudul. "Human emotion recognition system using optimally designed SVM with different facial feature extraction techniques." WSEAS Transactions on Computers 7.6 (2008).
The goal of this research is to create "Humanoid Robots" that can have intelligent conversations with people. The first step in this path is for a computer to use a neural network to recognise human emotions. In this essay, the six primary emotions: anger, disgust, fear, happiness, sadness, and surprise along with the neutral feeling are all acknowledged. The important characteristics for emotion recognition from facial expressions are extracted using a variety of feature extraction approaches, including Discrete Cosine Transform (DCT), Fast Fourier Transform (FFT), and Singular Value Decomposition (SVD). The performance of several feature extraction techniques is examined, and Support Vector Machine (SVM) is utilised to recognise emotions using the extracted face features. On the training dataset, the authors achieved 100% recognition accuracy and 92.29% on the cross-validation dataset.
B. Existing Systems
The majority of currently used recommendation systems are based on user's past ratings, listening history, or search history. Because the system only considers ratings history when making suggestions, it ignores other factors that can affect the forecast, such as the user's reaction, behaviour, feeling, or emotion. As a result, they are only able to have a static user experience. These elements must be included in order to alter and improve the user's present mood. It takes time to create a playlist using these existing recommendation methods. The trick is that the consumer could benefit from a more dynamic, personalised experience by taking such behavioural and emotional characteristics into account. Additionally, playlists are built more quickly and precisely.
III. PROPOSED SYSTEM
Our proposed system creates a mood-based music player that does real-time mood recognition and recommends songs in accordance with identified mood. This adds a new feature to the classic music player apps that are already pre-installed on our mobile devices. Customer satisfaction is a significant advantage of mood detection. The ability to read a person's emotions from their face is crucial. To gather the necessary information from the human face, a camera is employed. This input can be used, among other things, to extract data that can be used to infer a person's mood. Songs are generated using the "emotion" derived from the preceding input. This laborious chore of manually classifying songs into various lists is lessened, which aids in creating a playlist that is suitable for a particular person's emotional characteristics. In this research work, we implemented Machine Learning project Emotion Based Music Recommendation System using the Streamlit framework. The project is split into 3 phases: