11 minute read
SAMPLING
Next Article
Because of expense, time, and accessibility, collecting data from the whole target population is almost always unfeasible. Thus, researchers use techniques (sampling techniques) to decide on a subset or a smaller group, a sample, from the target population to collect data from. These sampling techniques are designed so that the data collected from the sample is representative of the whole target population. (Cohen, Manion & Morrison, 2007).
Basic sampling terms:
1. Population: is the entire group of people (or any other elements) who possess specific attributes that a researcher is interested to study. There are two types of population:
A. Target population: population under study to which the researcher wants to generalize the research findings.
B. Accessible population: part of the target population that is available to the researcher. (Phillips & Stawarski, 2016)
2. Element: Is the single member of the population under study. It is also called subjects, respondents, or participants.
3. Sampling: It is the process of selecting a portion of the population to obtain data regarding a research problem.
4. Sample: A portion of the population that has been selected to represent the population of interest, and from which data will be collected.
5. Sampling frame: It is a comprehensive list of all the sampling elements in the accessible population. The sample is selected from the sampling frame.
6. Representativeness: It is how well the sample represents the variables of interest in the target population, as well as other demographic characteristics.
The sample should replicate the population in approximately the same proportions as it occurs in the target or accessible population. This is achieved by randomization.
7. Sampling bias: It occurs when the researcher shows a preference in selecting one participant over another. This bias increases when random selection is not used.
8. Inclusion criteria: The characteristics that each sample element must possess in order to be included in the sample.
9. Exclusion criteria: The characteristics that an element may possess and could confound or contaminate the results of the study. Such elements should not be included in the sample.
10. Sampling plan: It is a description of the strategies that will be used to obtain the sample of the study, It should include the sample size, type, as well as inclusion and exclusion criteria.
11. Randomization: Randomization is a systematic process where the researcher can specify the chance of each element of the population to be selected for the sample. It is used to ensure that selections are made independent of each other and there is no bias in selecting the sample. Randomization is employed to ensure that the variable of interest will be present in the sample to the extent as it would be in the whole population. (Phillips & Stawarski, 2016).
Types of sampling:
There are two main types of sampling techniques: Probability Sampling and Nonprobability Sampling.
Probability (random) Sampling:
It is a sampling technique in which each element or member in the accessible population has an equal chance to be selected and included in the sample. To obtain a probability sample, the researcher has to identify every element in the accessible population. That is to develop a sampling frame.
There are four types of probability sampling: Simple, Stratified, Cluster, and Systematic. (Polit & Hungler, 1978)
Subtypes Simple Random Sampling
It Is the most basic probability sampling method. To achieve a simple random sampling the researcher first identifies the elements of the accessible population. The elements are randomly selected from the sampling frame. This could be achieved through a variety of procedures. In one procedure, names are written on slips of papers, placed in a container, mixed well, and then elements are drawn one at a time until the desired sample size is reached.
Although this procedure is sufficient to achieve simple random sampling, it could be time consuming and tiresome when a large sample size is desired. Thus, the most common way of achieving simple random sampling is using a table of random numbers. This table is usually computer-generated, and a number is assigned to each name in the sampling frame.
Numbers then are selected randomly to obtain a sample. Simple random sampling, however, has a couple of downsides which make it inconvenient to use: a complete list of accessible population is needed, which is impossible in many situations, and It does not guarantee a high representation level with very high population sizes. (Phillips & Stawarski, 2016).
Stratified Random Sampling
This method is used when the researcher knows some of the characteristics of the population (variables) that are critical in achieving representativeness. Here, the researcher divides the accessible population according to mutually exclusive variables.
Mutually exclusive means that each sample element belongs to only one group or stratum. Then, the researcher selects a sub-sample from each group or stratum randomly.
Moreover, Proportional stratified random sampling is a method that increases representativeness of variables in the sample. In this method, the number of elements taken from each stratum is proportional to the number of elements in the accessible population. This sampling technique ensures the representation of each sub-group in the accessible population. However, it requires specific information about the population in order to stratify it accurately, and a complete list of accessible population is required. (Polit & Hungler, 1978).
Cluster Sampling
Here, the population to be studied is too large and/or geographically scattered over a grand space (Polit & Hungler, 1978). Therefore, it is difficult or impossible to obtain a sampling frame for randomization. For example, if a study is to be conducted over all doctors in Egypt.
To select a random sample from this population, multistage/cluster random sampling is appropriate because simple and stratified techniques would be inconvenient. Cluster sampling is carried out through dividing the large populations in clusters using simple or stratified. Then, the researcher obtains sample frames out of these clusters to select the sample from. This process could be repeated until a reasonable cluster is reached where the researcher can obtain a sample frame and select a sample from that frame.
Because selecting a sample from clusters is done in two or more stages, cluster sampling is also referred to as Multi-stage random sampling. These multiple stages also contribute to increased sampling error. (Phillips & Stawarski, 2016).
Systematic Random Sampling
Like both simple and stratified random sampling, this method requires an ordered list of all members of the accessible population. This technique depends on selecting every kth subject from the sample frame (Phillips & Stawarski, 2016). To achieve this, the researcher would divide the population sum by the sample size desired to get the sampling interval (k).
For example, if a researcher wants a sample of 50 from a population of 400 subjects, the sampling interval (k)=400/50=8. This means that the researcher will choose every 8th subject from the population. While this technique is easy and inexpensive, the researcher needs to be careful when listing the population elements in the sample frame. Unless elements on the sample frame are randomized and do not have a certain order whatsoever, bias will occur. (Polit & Hungler, 1978).
Non-probability Sampling:
In non-probability sampling, random selection is not used. Thus, each element or participant in the accessible population does not have equal chance to be included in the sample. Accordingly, the sample may not be representative of the population, and the study results therefore cannot be generalized to the general population. However, this sampling technique is less expensive and less complicated. There are three types of non-probability sampling: Convenience, Quota, and Purposive Sampling. (Phillips & Stawarski, 2016)
Subtypes Convenience Sampling
This technique uses participants who are easily accessible to the researcher and who meet the criteria for inclusion. Thus, subjects/elements are included in the study just because they are available at a certain time in a certain place.
For example, a researcher might select a sample by choosing every fifth patient who enters the clinic on a certain day.
Snowball (Network) Sampling
Quota Sampling
It Is a type of convenience sampling. Snowball sampling is when the researcher gets assistance from subjects to bring more subjects who meet the inclusion criteria into the study. (Phillips & Stawarski, 2016)
Purposive (Judgmental)
This method is similar to the stratified random sampling in that the participants are divided into strata based on specified characteristics to provide representativeness of different groups within the accessible population. Yet, this differs from stratified random sampling in that the elements are not randomly selected from each group/stratum (Polit & Hungler, 1978). The researcher first determines which strata are to be studied. A stratum can be age group, sex, educational level, diagnosis, etc. Then, the researcher will calculate the quota (or number) of participants needed for each stratum. This quota can be computed proportionally to the population. Members are then selected from each quota conveniently and not randomly.
In this technique, the researcher (based on his knowledge and/or experience) selects the elements of the study sample. According to the researcher, the chosen elements are thought to best represent the phenomenon being studied.
Sampling
This sampling technique is usually used in qualitative research. This technique allows the researcher to handpick, so it is easy, simple, and time saving. However, the generalizability of the research findings is very limited. (Polit & Hungler, 1978)
Choosing the sampling technique:
To choose an appropriate sampling technique, the researcher should identify the research goals and select the sampling methods that would achieve these goals.
Next the researcher should test each method for satisfying the research goal and then choose the best method. Also the researcher should consider the inclusiveness of the sample and representability.
Also, the feasibility, resources and access to population play great roles in the final decision for a sampling strategy.
Cross-sectional studies (transverse studies, prevalence studies)
Definition
A cross-sectional study is defined as a type of observational research that analyzes data of variables collected at one given point in time across a sample population or a pre-defined subset like a snapshot.
Characteristics
Researchers can conduct a cross-sectional study with the same set of variables over a set period.
Similar research may look at the same variable of interest, but each study observes a new set of subjects.
The cross-sectional analysis assesses topics during a single instance with a defined start and stopping point, unlike longitudinal studies, where variables can change during extensive research.
Cross-sectional studies allow the researcher to look at one independent variable as the focus of the cross-sectional study and one or more dependent variables.
In which the investigator measures the outcome and the exposures in the study participants at the same time.
Types
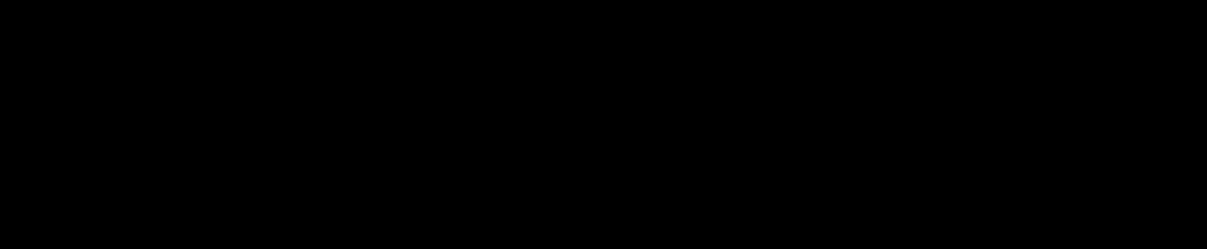
Cross-sectional studies can be classified as descriptive or analytical, depending on whether the outcome variable is assessed for potential associations with exposures or risk factors and there is another type which is repeated (or serial) cross-sectional study.
Descriptive
1. Assesses how frequently, widely, or severely the variable of interest occurs throughout a specific demographic.
2. Simply characterizes the prevalence of one or multiple health outcomes in a specified population.
3. Generates hypotheses.
4. Answers what, who, where, and when questions.
Analytical
1. Investigators collect data for both exposures and outcomes at one specific point in time for the purpose of comparing outcome differences between exposed and unexposed subjects
2. Investigates the association between two related or unrelated parameters
3. Tests hypotheses
4. Answers why and how
Repeated (or serial)
Data collection is conducted on the same target population at different time points.
At each time point, investigators take a different sample (different subjects) of the target population, thus, repeated cross-sectional studies can be used for analyzing population changes over time (also known as aggregate change over time).
They cannot be used to look at individual change (as in a cohort study).
For example, Zito et al.
Using annual cross-sectional surveys, Zito et al. reported that the prevalence of prescription psychotropic drug use among youth (people <20 years old) increased more than threefold between 1987 and 1996 in a mid-Atlantic Medicaid population. This is not a cohort design because it does not follow a single group of people over time; there are changes in the population over time due to births, deaths, aging, migration, and eligibility changes.
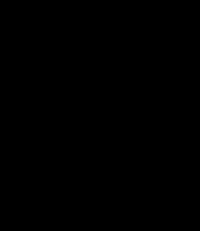
Importance
Learn about characteristics such as knowledge, attitude, and practices of individuals in a population.
Used to collect all variables at one time.
Multiple outcomes can be researched at once.
Prevalence for all factors can be measured which can be assessed at either one point in time (point prevalence) or over a defined period (period prevalence).
Suitable for descriptive analysis.
Researchers can use it as a springboard for further research.
Monitors trends over time with serial cross-sectional studies.
Method
We define a population and determine the presence or absence of exposure and the presence or absence of disease for everyone at the same time. Each subject then can be categorized into one of four possible subgroups.
Design of a hypothetical cross-sectional study: I. Identification of four subgroups based on presence or absence of exposure and presence or absence of disease.
There will be (a) persons who have been exposed and have the disease; (b) persons who have been exposed but do not have the disease; (c) persons who have the disease but have not been exposed; and (d) persons who have neither been exposed nor have the disease. If we use (a) and (b), we can compare the prevalence of exposure in persons with the disease to the prevalence of exposure in persons without the disease.
Design of a hypothetical cross-sectional study II: (top) A 2 × 2 table of the findings from the study; (bottom) two possible approaches to the analysis of results: (A) Calculate the prevalence of disease in exposed persons compared to the prevalence of disease in non-exposed persons, or (B) Calculate the prevalence of exposure in persons with disease compared to the prevalence of exposure in persons without disease.
Strengths of a Cross-sectional Study
Fast and Inexpensive.
No ethical difficulties.
There is no loss to follow-up.
These studies are conducted either before planning a cohort study or a baseline in a cohort study. These types of designs will give us information about the prevalence of outcomes or exposures; this information will be useful for designing the cohort study.
They may be useful for public health planning, monitoring, and evaluation.
Data on all variables is only collected once.
Able to measure prevalence for all factors under investigation.
Multiple outcomes and exposures can be studied.
The prevalence of disease or other health related characteristics are important in public health for assessing the burden of disease in a specified population and in planning and allocating health resources.
Good for descriptive analyses and for generating hypotheses.
Limitations
It is difficult to derive causal relationships from cross-sectional analysis.
As cross-sectional studies measure prevalent rather than incident cases, the data will always reflect determinants of survival as well as etiology.
Unable to measure incidence.
Associations identified may be difficult to interpret.
Susceptible to bias either due to low response and misclassification due to recall bias, temporal bias, survival, or selection bias.
Generalizability limited by sampled population and population definition
Examples
Study sought to determine the prevalence of asthma in children and analyze its association with being a passive smoker, being exposed to vehicular traffic (both risk factors) and the intake of dehydrated fruit (a possible protective factors) the researchers found that the prevalence of asthma increased with the number of smokers with whom they lived, but it was not associated with living near the main avenue or the consumption of dehydrated fruits. Thus, in the cross-sectional study, there are both descriptive (an estimate of prevalence) and analytical components (study of association between variables).
Receiving voluntary family planning services has no relationship with the paradoxical situation of high use of contraceptives and abortion in Vietnam.
Patient-Related Factors Influencing Satisfaction in the Patient-Doctor Encounters at the General Outpatient Clinic of the University of Calabar Teaching Hospital, Calabar, Nigeria.
Cross-sectional pilot study of antibiotic resistance in Propionibacterium acnes strains in Indian acne patients using 16s-RNA polymerase chain reaction: A comparison among treatment modalities including antibiotics, benzoyl peroxide, and isotretinoin.