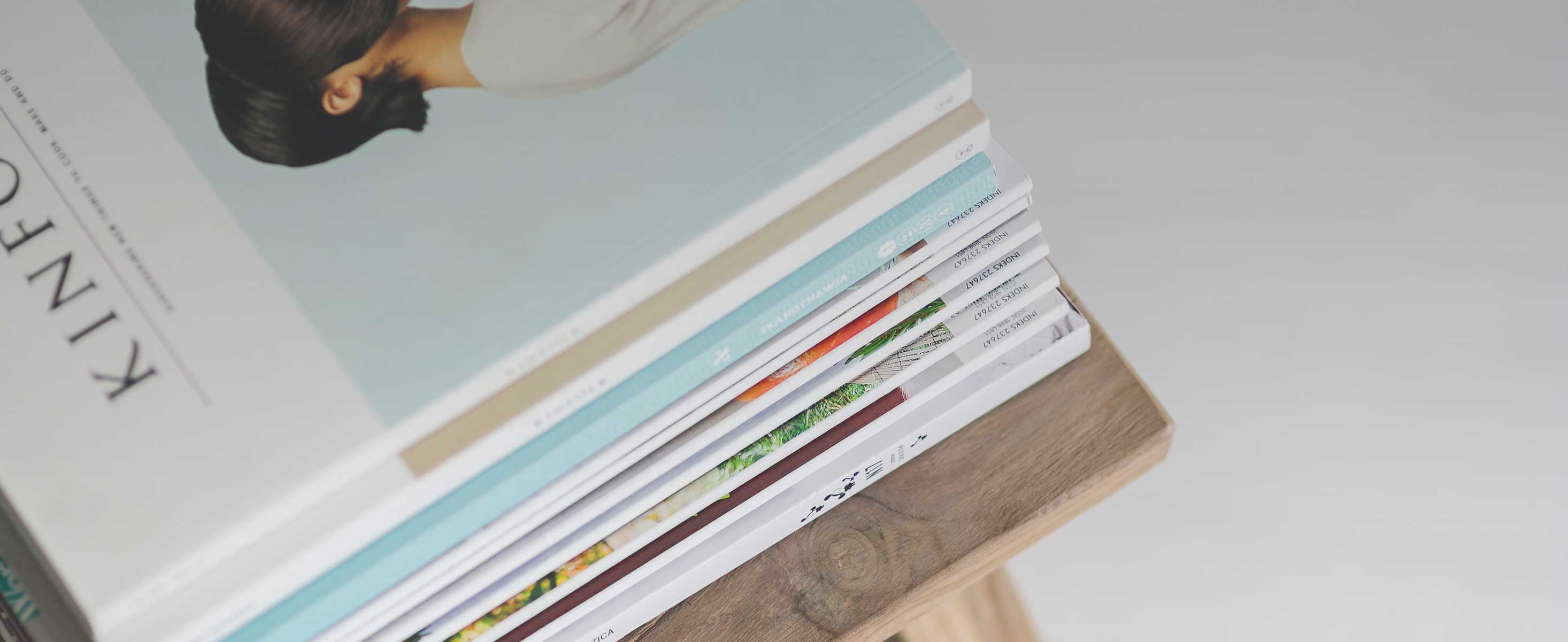
1 minute read
Human Biases in the eyes of AI
We’ve all grown used to algorithms helping us make decisions like what show to binge watch, or what our next online purchase should be. The strength of AI systems and the reason for their wider adoption is their ability to identify patterns in existing data to make accurate risk predictions. In line with recent research, Arul Mishra believes that this strength becomes a weakness when existing data include historic human biases, including race, gender, age, or income-based biases. AI systems, unfortunately, represent these biases as relevant rules that humans utilize in their decision-making processes, which leads to a cycle of discrimination, in which models learn biases from past human decisions, and themselves make inequitable predictions.
Mishra utilizes different methods, causal and computational, to examine how algorithms might influence whether a consumer will receive a line of credit; whether a defendant will be denied a favorable sentence; or whether a consumer will be shown different job or product advertisements all based on their group membership such as race, gender, income, age, etc. Working with several for-profit (e.g., national lenders, retail outlets) and non-profit organizations (e.g., legal organizations working for better access to justice), she has examined whether algorithmic predictions could be biased, the causes of such bias, the individual and social impact of the bias, and importantly, how the algorithms can be debiased. ■
Arul Mishra
Professor, Marketing
Arul Mishra is a Professor of Marketing at the David Eccles School of Business. Her research focuses on understanding different aspects of a person’s decision-making process. She is interested in examining research questions in the domains of consumer decision-making, behavioral promotions, risk perception, and financial decision-making.
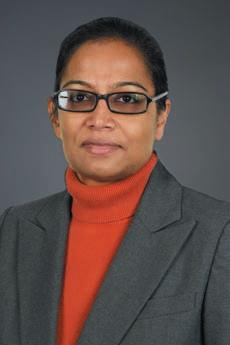