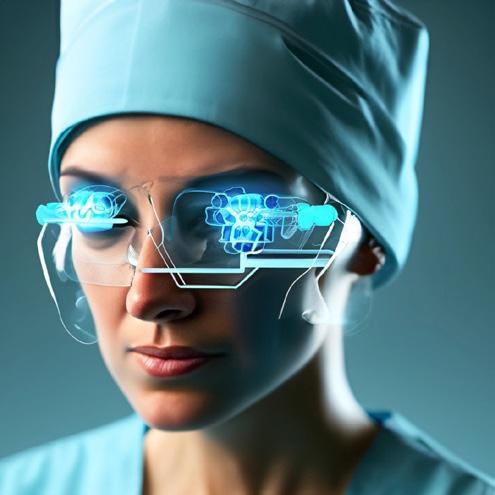
19 minute read
Ready or Not, More AI Tools are Coming to Your Nursing Practice Setting
by Christine Riley, MSN, RN
Introduction
Every nurse remembers the day they took their licensure exam. If a nurse was licensed before 1994, the nurse may have even taken that exam with a pencil and test booklet and waited up to twelve weeks for the results! The National Council of State Boards of Nursing (NCSBN) began conducting studies with computer adaptive testing (CAT) in 1986 and administered the first National Council Licensure Examination (NCLEX) exam via CAT on April 1, 1994 (NCSBN, 2014). With CAT, the computer automatically selects questions from a pool and adapts subsequent questions based on previous responses. For many nurses, this exam may have been one of their first exposures to artificial intelligence (AI) in the field of nursing.
The umbrella term AI describes the tools and techniques which are used to teach computers to mimic human-like cognitive functions, such as learning, reasoning, communicating and decision making (Robert, 2019; von Gerich, et al., 2022). Thoughts of AI may go straight to human-like robots, but AI is much broader than that. Think about when a cell phone application for driving directions automatically reroutes the driver based on traffic patterns, or asking a digital assistant such as “Alexa” or “Siri” what the latest news headlines are. The examples are endless and continue into the healthcare and nursing realms, such as smart insulin pens, or automated alerts that pop up after analyzing data in electronic health records.
The purpose of this article is to give a glimpse into the state of AI as it relates to healthcare and safe nursing practice. This snapshot is not a comprehensive review as AI in healthcare is accelerating at a rapid pace and contains numerous types of technology, not all of which are discussed here. However, nurses should be aware of the general terms related to AI, and how to incorporate these tools in nursing practice while followingTexas Board of Nursing (BON or Board) rules and regulations.
The research process for this article started with a review of NCSBN Environmental Scans over the last five years and a search in PubMed of the following terms in “title/ abstract”: artificial intelligence, AI, healthcare, health, AND care, and the following terms in any field: nurse AND nursing. Results were limited to those written within the last five years and with free access so readers could refer to resources as well. It should also be mentioned that an AI tool, ChatGPT, was used to create an initial outline for this article, which was then modified by this author. ChatGPT is an example of a large language model (LLM). This type of model is designed to process enormous amounts of text data to learn language patterns and mimic human intelligence. See Diagram 1 on page 10 for a visual representation of the AI terms used in this article.
Current Applications of AI in Nursing
A term that comes up frequently in relation to AI is machine learning (ML). Rong et al.(2022) defined machine learning (ML) as the computerized modeling of various components of the process of acquisition of knowledge. This means ML is a computer’s way of simulating human learning by processing data and algorithms, learning from the data processed to evolve and improve performance over time. The ability of algorithms to self-learn and develop via practice is one of the most important aims of machine learning (Rong, et al., 2022). In one study, utilizing data from electronic health records, this ML approach was applied to find and rank predictive factors of hospitalized patient falls. With the ability to forecast factors that influence patient outcomes, prediction models have the potential to support healthcare provider decision making and ultimately improve quality of care (Lindberg, et al., 2020).
Another application of ML for healthcare improvement came via a study that used nursing flowsheet data to identify characteristics of pressure injuries and develop a predictive model for pressure injury events. The authors cited the previous limitations of applying traditional assessment tools, like the Braden Scale, and saw a need for an additional tool, such as a predictive model. While this study was still pending external validation, the initial results concluded that the model accurately predicted pressure injury development and may be helpful in pressure injury prevention (Song et al., 2021).
ML often overlaps with predictive analytics to develop these predictive models, like the ones mentioned above for predicting fall risk and pressure injuries. Predictive analytics is the computational analysis of data and statistics to find patterns and predict future outcomes (Wakefield, 2023). When combined, ML and predictive analytics utilize data analysis, statistics, and machine learning algorithms. This approach enables the predictive model to respond to new data over time, providing a tool for enhanced decision-making. Researchers also incorporated this process in the construction of a predictive model to optimize nurse-patient allocation. Through data collection, analysis of that data and nursing processes, this model demonstrated the high variability of patient care requirements and proposed a method to make more balanced nurse to patient ratio assignments (Moreno-Fergusson et al., 2021).
Remote patient monitoring can also be applied with AI in nursing. This term encompasses devices that allow a health care team to monitor a patient’s vital signs and changes in condition from a distance (NCSBN, 2019; NCSBN 2023). Examples of remote monitoring tools might include blood pressure monitors, pulse oximeters, cardiac event recorders, positive airway pressure machines, and physical activity trackers. One of the studies reviewed focused on remote monitoring of patients in their homes with the use of sensors. The continuous sensor-based monitoring of patients in their “smart homes” provided clinical insight into symptoms experienced by older adults between office visits. Patient data collected from these sensors and ML algorithms were used to provide automated detection of health events. The study found that nurses could use this data to initiate preventive strategies and provide timely intervention to avoid adverse health events (Fritz, et al., 2022).
AI-assisted diagnosis also came up in the review of AI in healthcare. Research from the National Library of Medicine defined medical diagnostics as the “process of evaluating medical conditions or diseases by analyzing symptoms, medical history and test results” (Al-Antari, 2023). When AI is used to assist in medical diagnostics, providers incorporate mechanisms to interpret massive amounts of patient data, such as algorithms to analyze medical images (e.g., X-rays, MRIs, ultrasounds, CT scans, and DXAs), vital signs, medical history, lab test results and demographic information. Findings can allow healthcare providers to identify and diagnose diseases more accurately and quickly (Al-Antari, 2023). There has been concern for the potential of misdiagnosis, leading to incorrect or potentially unsafe treatment options. However, some ML methods associated with AI assisted diagnosis may be more accurate at diagnosing a health condition, such as detecting a cancer via image analysis, where a human might have missed the finding (NCSBN, 2019; Fakoor et al., 2013; Vial et al., 2018 as cited in NCSBN, 2020). AI researchers and clinicians have emphasized that the final word,
decision, diagnosis, and treatment choices should not be made by AI. Rather, AI can be a tool for practitioners, or offer a complementary role in the diagnostic process.
It would be hard to give a full picture of AI in healthcare without talking about robotics. On its own, robotics is defined as the “engineering and operation of machines that can autonomously or semi-autonomously perform physical tasks on behalf of a human” (Soriano et al., 2022). Robots routinely perform simple tasks such as automated medication reminders or deliver a medication to a patient in isolation. One article showed how robots are assisting the elderly with tasks such as bathing, task reminders, monitoring, supervising wellness activities, giving exercises for cognitive stimulation, and music therapy (Rong et al., 2022).
Robots alone do not incorporate AI, but the combination of robots with AI is emerging in healthcare. Natural language processing (NLP) is a branch of AI where computers analyze text or speech data and mimic human understanding. This mechanism was illustrated in one study where nurse chatbots simulated conversation with patients to assist with self-management of chronic diseases, and increase access to healthcare information (Hernandez, 2019). The large language model (LLM) mentioned earlier, ChatGPT, is a specific application of NLP which goes beyond basic text analysis and uses advanced algorithms to learn from vast amounts of data and generate convincingly human-like text.
When these elements of AI, such as NLP, LLM and ML, are combined with the physical hardware or “body” of a robot, these AI embedded robots have the potential to modify and adapt behavior and perform more complex tasks. Such tasks may include communicating with persons with limited social participation and engagement, serving as a companion, or tailoring therapeutic activities to an individual’s needs (Rong et al., 2022; Soriano et al., 2022).
Researchers have described robotics in nursing as “systems of mechanical, electrical, and control mechanisms used by trained operators in a professional health care setting that perform tasks in direct interaction with patients, nurses, doctors, and other health care professionals” (von Gerich et al., 2022; Soriano et al., 2022). These systems can “modify their behavior based on what they sense in the environment” (von Gerich et al., 2022; Soriano et al., 2022).
Some of these authors recommended approaching robotics in nursing as a “three-party relationship between patients, nurses (healthcare professionals), and robots and to effectively use robots and AI as tools and technologies in this relationship” (Soriano et al., 2022). It is also important to clarify how the robot should function in each environment and among team members and for the healthcare team to provide feedback for developing new robots.
training to prepare future nurses for the use of such technology in the workplace (Pepito & Locsin, 2019; Thibault, 2020 as cited in NCSBN, 2021). Similar to how CAT personalizes the exam to the student, virtual simulations offer an individualized learning experience. The NCSBN Environmental Scan from 2023 showcased an example from the Ohio State College of Nursing which partnered with College of Engineering to build “extended reality learning tools” to prepare student nurses for clinical settings and test them on clinical scenarios (NCSBN, 2023). These adaptive learning experiences challenge students at their current level of competency and can be especially useful where clinical experiences are hard to come by.
Benefits and Challenges of AI in Nursing
The use of AI tools offers potential benefits to nurses and patients. This article has covered examples of AI assisting in decision making with healthcare providers through AI assisted diagnosis and the use of AI generated tools to predict adverse health events or craft balanced nurse-patient ratio assignments (Lindberg, et al., 2020; Song et al., 2021; Fritz, et al., 2022; Moreno-Fergusson, et al., 2021).
There are also some aspects of AI which could be interpreted as a challenge or benefit to the nursing profession. While some have expressed fear that AI could eventually take over jobs, the nursing workforce is facing increasing demands with more complex
patients, and the added stresses of frequent staffing shortages and burnout, which were only exacerbated by the COVID-19 pandemic. Researchers have estimated that by the year 2025, the US may have a gap of 200,000 to 450,000 nurses (Berlin et al., 2022, as cited in NCSBN, 2023). Texas has one of the lower ratios of employed RNs per population with fewer than 750 RNs per 100,000 people (U.S. Bureau of Labor Statistics, 2022 as cited in NCSBN 2023). With the current nursing shortage in many parts of the US and anticipated worsening shortage of nurses, it’s important to consider how AI tools can help address the nursing shortage, reduce risk of burnout, and compensate for nurses leaving the workforce (NCSBN, 2023).
Novel technologies may not take the place of a nurse any time soon, but many envision changes in nursing roles and responsibilities due to AI integration. There may be different competencies and skills for nurses to learn in healthcare environments which incorporate AI tools. A report by McKinsey Global Institute argues that there is already a robotic revolution happening in nursing and these robots have made tasks and procedures more efficient and safer (McKinsey Global Institute, 2017 as cited in Pepito & Locsin, 2019). Pepito and Locsin provided examples of how robots are already working in hospitals as supplemental healthcare workers, as companions, and automated medication dispensers (Pepito & Locsin, 2019). Beyond select nursing tasks, researchers have expressed concerns regarding
the capability of robots to express human like characteristics, such as compassion, and empathy (Mason, 2021; van Wynsberghe, 2014 as cited in Soriano et al., 2022). Furthermore, nurses have skills that AI has a harder time replicating, such as critical thinking and reacting accordingly to unpredictable human behavior and emotions which are subjective and constantly changing (Pepito& Locsin, 2019).
One of the challenges of AI in nursing was cited in the literature review by von Gerich et al., which found that AI research applicable to nursing lacks comprehensive evaluation of outcomes regarding the subject of the AI project (von Gerich et al., 2022). Not every new tool is better than what was used before, and it is important to verify usefulness and explain to nurses how the given tool will benefit their practice and patient care. These researchers also found a “sparsity of nurses in AI development”, highlighting the need for more nurse involvement and understanding of AI tools.
Future Directions and Implications for Nursing
It helps that nurses are naturally set up for innovation by way of working in healthcare settings and fields that are constantly evolving. Nurses must learn how to adapt to advancing technology, how to supplement and enhance skills, and ultimately determine the trajectory of how the nursing profession is to impact and work with advancing technologies (Pepito & Locsin, 2019). Nurses are poised to advocate for the use of
AI tools in their practice area or come up with their own devices to advance methods of patient care and improve healthcare outcomes. Remember that the standards of nursing practice require nurses of all levels of licensure to make a reasonable effort to obtain orientation/training for competency when encountering new equipment and technology or unfamiliar care situations [Board Rule 217.11(1)]. Another standard requires nurses to be responsible for their own continuing competence in nursing practice and individual professional growth [Board Rule 217.11(1)(R)].
Research indicates that whether nurses are directly involved in AI development or not, it benefits nurses to help decide which aspects of their role can be enhanced with technology and which aspects cannot, while ensuring the needs of the patient are met (Pepito & Locsin, 2019). With so many opportunities for AI to be involved in nursing and patient care, the onus is on nurses to uphold the Nursing Practice Act and all Board Rules when incorporating AI tools into practice, such as clarifying any order or treatment regimen that the nurse believes is inaccurate, non-efficacious, or contraindicated by consulting with the appropriate licensed practitioner, notifying the ordering practitioner when the nurse makes the decision to not carry out the order or treatment, and collaborating with the healthcare team in the best interest of the patient [Board Rule 217.11(1) (N) & (P)].
When incorporating novel technology such as AI into nursing practice, nurses should also consider the ethical principles of nursing and how those relate to the use of AI. One of these ethical principles is autonomy, or the freedom to choose and goes along with being a patient advocate. Nurses must be informed and able to educate patients on all elements of the care plan so the patient can make an informed decision [Board Rule 217.11(1)(F)]. Similarly, when it comes to making decisions regarding a patient’s healthcare, that decision is ultimately made by the patient and healthcare provider, while possibly making use of AI tools (WHO, 2021, as cited in NCSBN, 2022).
Another ethical principle is non-maleficence, or “do no harm”. AI should not be harmful but should support the principle of beneficence or “doing good”. Patient safety is paramount in nursing care and nurses are obligated to implement measures to promote a safe environment for others [Board Rule 217.11 (1)(B)]. The World Health Organization (WHO) also supports that AI must be transparent, inclusive, and equitable, which goes along with other ethical principles of fidelity and justice (WHO, 2021, as cited in NCSBN, 2022). If using AI generated tools such as algorithms, nurses should be aware of potential biases and how certain algorithms may work better for some populations, because those algorithms were only tested among certain populations (von Gerich et al., 2022; NCSBN, 2020). For nurses working in the development of AI tools, it’s important to be aware
of biases in data collection and know who the technology is being developed for, the end users, the intended population, the intended setting, intended age group, etc., and incorporate those stakeholders in the development [Board Rule 217.11(1)(L)]. Researchers and developers should also make efforts to ensure accessibility of these tools benefit all populations and healthcare settings.
Conclusion
Just as all nurses should know how to take a manual blood pressure in case the blood pressure machine malfunctions or to double-check a reading, the nursing knowledge, skills, and judgment from human nurses are essential in the provision of safe patient care, regardless of whether AI tools are used. With respect to evolving technology and AI, the Board continues with the mission to protect and promote the welfare of the people of Texas by ensuring that each person holding a license as a nurse in the State of Texas is competent to practice safely. AI is either already in a nurse's area of practice or will be coming soon, and it is up to nurses to incorporate AI into their practice safely.
References
22 Texas Administrative Code, §217.11 (2007). https://www.bon.texas.gov/ rr_current/217-11.asp.html
Al-Antari M. A. (2023). Artificial intelligence for medical diagnostics-existing and future AI technology. Diagnostics (Basel, Switzerland), 13(4), 688. https://doi. org/10.3390/diagnostics13040688
Berlin, G., Lapointe, M., Murphy, M., & Wexler, J. (2022, May 11). Assessing
the lingering impact of COVID-19 on the nursing workforce. McKinsey & Company. https://www.mckinsey.com/industries/ healthcare-systems-and-services/our-insights/assessing-the-lingering-impact-ofcovid-19-on-the-nursing-workforce
Fakoor, R., Ladhak, F., Nazi, A., & Huber, M. (2013, June). Using deep learning to enhance cancer diagnosis and classification. In Proceedings of the international conference on machine learning (Vol. 28). New York, USA: ACM.
Fritz, R., Wuestney, K., Dermody, G., & Cook, D. J. (2022). Nurse-in-the-loop smart home detection of health events associated with diagnosed chronic conditions: A case-event series. International journal of nursing studies advances, 4, 100081. https://doi.org/10.1016/j.ijnsa.2022.100081
Hernandez, J.P. (2019). Network diffusion and technology acceptance of a nurse chatbot for chronic disease self-management support: A theoretical perspective. The Journal of Medical Investigation, Volume 66 Issue 1.2 Pages 24-30. https:// doi.org/10.2152/jmi.66.24
Lindberg, D. S., Prosperi, M., Bjarnadottir, R. I., Thomas, J., Crane, M., Chen, Z., Shear, K., Solberg, L. M., Snigurska, U. A., Wu, Y., Xia, Y., & Lucero, R. J. (2020). Identification of important factors in an inpatient fall risk prediction model to improve the quality of care using EHR and electronic administrative data: A machine-learning approach. International journal of medical informatics, 143, 104272. https://doi.org/10.1016/j.ijmedinf.2020.104272
Mason, C. (2021). Artificial Compassion—From An AI Scholar. Preprints 2021. https://doi:10.20944/preprints202104.0784.v1
McKinsey Global Institute. (2017). JOBS LOST, JOBS GAINED: workforce transitions in a time of automation. San Francisco: McKinsey & Company.
Moreno-Fergusson, M. E., Guerrero Rueda, W. J., Ortiz Basto, G. A., Arevalo Sandoval, I. A. L., & Sanchez-Herrera, B. (2021). Analytics and lean health care to address nurse care management challenges for inpatients in emerging economies. Journal of nursing scholarship : an official publi-
cation of Sigma Theta Tau International Honor Society of Nursing, 53(6), 803–814. https://doi.org/10.1111/jnu.12711
National Council of State Boards of Nursing (NCSBN). (2014). Pencils down, booklets closed. In Focus. (Spring 2014, pp 10-13).
National Council of State Boards of Nursing (NCSBN). (2019). The NCSBN 2019 environmental scan: 40th anniversary edition. Journal of Nursing Regulation, S1-S40, 9(4). https://doi: 10.1016/S21558256(18)30177-7
National Council of State Boards of Nursing (NCSBN). (2021). NCSBN’s environmental scan COVID-19 and its impact on nursing and regulation. Journal of Nursing Regulation, S1-S36, 11(4). https:// Doi:10.1016/S2155-8256(21)00002-8
National Council of State Boards of Nursing (NCSBN). (2023). The NCSBN 2023 environmental scan: Nursing at a crossroads--an opportunity for action. Journal of Nursing Regulation, S10-S48, 13(4). DOI: 10.1016/S2155-8256(23)00006-6
Pepito, J.A., and Locsin, R. (2019). Can nurses remain relevant in a technologically advanced future? International Journal of Nursing Sciences. 106-110. https:// DOI: 10.1016/j.ijnss.2018.09.013
Robert, N. How artificial intelligence is changing nursing. Nurs Manage. (2019) Sep;50(9):30-39. https://doi: 10.1097/01. NUMA.0000578988.56622.21. PMID: 31425440; PMCID: PMC7597764.
Song, W., Kang, M. J., Zhang, L., Jung, W., Song, J., Bates, D. W., & Dykes, P. C. (2021). Predicting pressure injury using nursing assessment phenotypes and machine learning methods. Journal of the American Medical Informatics Association : JAMIA, 28(4), 759–765. https://doi. org/10.1093/jamia/ocaa336
Soriano, G.P., Yasuhara, Y., Ito, H., Matsumoto, K., Osaka, K., Kai, Y., Locsin, R., Schoenhofer, S., and Tanioka, T. (2022). Robots and robotics in nursing. Healthcare 2022, 10, 1571, 10(8). https://doi. org/10.3390/healthcare10081571
Thibault, G. E. (2020). The future of health professions education: Emerging trends in the United States. FASEB BioAdvances, 2(12), 685–694. https://doi.org/10.1096/ fba.2020-00061
U.S. Bureau of Labor Statistics. (2022). Occupational Employment Statistics. Retrieved from, http://www.bls.gov/oes/ oes_emp.htm
van Wynsberghe, A. (2015). Healthcare robots: Ethics, design and implementa-
tion in emerging technologies. Ethics and International Affairs; Routledge: London, UK, 2015. ISBN 9781032098609
Vial, A. Stirling, D., Field, M., Ros, M., Ritz, C., Carolan, M. & Miller, A.A. (2018). The role of deep learning and radiomic feature extraction in cancer-specific predictive modelling: a review. Translational Cancer Research, 7(3), 803-816.
von Gerich, H., Moen, H., Block, L., Chu, C., DeForest, H., Hobensack, M., Michalowski, M., Mitchell, J., Nibber, R., Olalia, M., Pruinelli, L., Ronquillo, C., Topaz, M., and Peltonen, L. (2022). Artificial intelligence-based technologies in nursing: A scoping literature review of the evidence. International Journal of Nursing Studies. Mar;127:104153. https://doi: 10.1016/j. ijnurstu.2021.104153. Epub 2021 Dec 7. PMID: 35092870.
Wakefield, K. (2023). Predictive modeling analytics and machine learning. SAS Institute. Retrieved from https:// www.sas.com/en_gb/insights/articles/ analytics/a-guide-to-predictive-analytics-and-machine-learning.html
World Health Organization. (2021). Ethics and governance of artificial intelligence for health. WHO Guidance. https://apps. who.int/iris/handle/10665/341996. License: CC BY-NC-SA 3.0 IGO