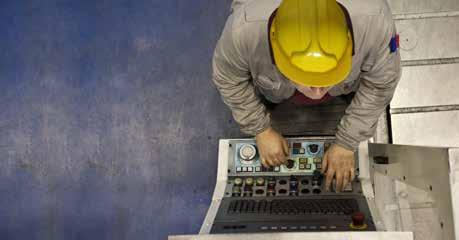
4 minute read
Manufacturing insights for all
from AMT OCT/NOV 2021
by AMTIL
Imagine what could be accomplished if everyone has access to the data they need and knows what to do with it, writes Owen Keates.
The democratisation of manufacturing data won’t unlock the value of the Industry 4.0 era. Despite all the industry talk about it, democratisation of data isn’t enough. You need to focus on the democratisation of insights. You need to get actionable insights based on real-time data into the hands of people who can use them, to achieve business objectives such as fewer shutdowns, improved health and safety outcomes, higher productivity, better throughput or increased profit margin. But will your people actually trust these insights? Every business will experience this somewhat differently, but there are challenges we consistently observe, and tried and true tactics that work. The amount of data isn’t the only issue
There’s so much data to manage, and so many different types of data are required to paint the whole picture. Manufacturers need the infrastructure to support the seamless integration of data from a variety of disparate sources; data of different types and in different formats, structured differently or not at all. To make the most of your data, you need to be able to ingest all that disparate data and make it useful and available to analytics, automation and machine learning solutions. You also need to know what questions to answer, and what data sets to select. This is a whole-of-business task
Democratisation of data is all about making digital information accessible to everyone across the business that needs it, whether or not they have technical data skills. Employees shouldn’t need to request help from the IT team, coding or new tools every time someone needs data access. It’s time to enabling the sharing of data across multiple parts of the business and put an end to gatekeeping. Democratisation of insights takes that to a new level, involving the right mix of machine learning, analytics, automation, custom algorithms and more to draw useful insights out of the right data sets. It involves mixing data with suitable external data sets. And, crucially, it means feeding those insights to the people who need them, when they need them. You can’t make it IT’s responsibility to figure out how to sort all of that out. They can’t read the minds of everyone who needs data. Include the people who will actually use the insights, so you know what questions you need the data to answer. Recognise that different roles may require different views of the same data in order to rapidly comprehend, contextualise and action the information and insights from this data. It’s no good if people don’t trust the data
Involving a cross section of your people early in this process is best practice. That includes people who have always based decisions on gut feel and experience. You need the machine learning to make the kinds of decisions these subject matter experts would make if they could combine that experience with all the data and processing power of advanced analytics solutions. Invite your team members to participate in discussions with the data engineers and data scientists around how the analytics work, how machine learning algorithms are trained, or AI systems are developed, so that trust in the data and the process can be strengthened. The initial results of a predictive algorithm may not be very accurate, but models can be continually improved with support from the team. Then, when you start democratising insights, they will recognise those insights as having been developed with their input. That’s powerful. The interface and user experience matter
If your colleagues have been using the same tools and processes for a long time, they are likely to be resistent to new ones even if you promise them better information. Invite team members to contribute to the design of the user interfaces. Consider solutions that work within your existing ecosystem, integrate with familiar tools, and even present the insights via existing dashboards or trusted interfaces. Most solution providers want to sell you on a ‘single pane of glass’ – while that’s the ideal outcome, that pane of glass can be your current system with new, modernised solutions in the background. Ease of use goes a long way towards user adoption and return on investment. Solve specific challenges
To achieve true business value you need to quantify your technology investment against real business objectives. Here are two examples: • Tackle management of hygiene within manufacturing operations, by leveraging data gathered via industrial
IoT devices and applying advanced analytics. Monitor this data in real time and feed predictive, actionable insights to your personnel. • Reduce the impacts of supply chain disruption on your production flow. If your team members are fed insights based on a real-time assessment of production and assembly processes, they can dynamically reschedule processes or substitute components.
This kind of flexibility is mission critical when component supply can be suddenly interrupted by border closures or other shipping delays. With the world still in a state of uncertainty, it’s more important than ever to be datadriven. To do so, you must be able to make your data available, reliable and presented as actionable insights. Quality insights lead to improved decision-making. Democratisation of insights gives a business significant competitive advantage when all team members embrace the knowledge generated.