6 minute read
Judgement calls
Judgementcalls
Intelligent AI’s Anthony Peake was motivated by a tragedy to improve the quality of data used to identify risk. But with that comes great responsibility
“The reason we started Intelligent AI was Grenfell Tower.”
Anthony Peake, co-founder and CEO of the platform for risk underwriters, is talking about the night in June 2017 when fire ripped through a 24-storey block of flats in West London, leaving 72 people dead and exposing a shocking catalogue of safety failures. From the combustible cladding that fed the flames, to the woeful lack of readily available, detailed information on the building’s construction and layout that hampered rescue efforts, the data that might have flagged Grenfell as a disaster waiting to happen had never been fully collated or, more importantly, shared. Insurers were as much in the dark as anyone else.
Like many watching the tragedy unfold live on TV, Peake, who’d spent a lifetime in IT, was first appalled and then angry that information technology could have been used to avert this and other disasters, but wasn’t.
At the time of the Grenfell Tower fire, Peake was already involved in analysing fire service call-out data on behalf of insurers elsewhere, and he was curious to see the records for the block. It emerged that firefighters had been called to the tower 15 times during the previous year, but no claims had been made by residents as half did not have insurance, and the other half could not afford it, so there was no obvious data trail to inform any underwriting process.
“You had two different views of risk, one side completely blind (insurers) the other side (fire services) data-rich. And none of the insurers involved with Grenfell at that time were looking at fire service call-out data,” says Peake. surveillance of autonomous processes.
IBM found that, while 71 per cent of insurers have data-centric products and services in their portfolios, many still lack a cohesive data strategy. And yet the information and level of detail they have access to is only likely to grow. According to Accenture’s 2021 Global Insurance Consumer Study, seven out of 10 people would share their data, including medical records and driving habits, with insurers if it meant more personalised pricing. The responsible application of AI in determining outcomes based on this data requires insurers to do two things: ensure transparency in their sourcing of data and, secondly, monitor for bias in decision-making algorithms.
Since last year, heavy-hitters like Google and Microsoft have committed to encouraging more ethical digital practices, providing advice on data security and AI development to the wider industry. And, in 2021, Intelligent AI teamed up with tech organisation Digital Catapult as part the Innovate UK ethical AI project, which aims to increase support for companies like Peake’s as they develop and deploy AI. The aim is to make sure it is used in a way that does not unintentionally harm any individual or society at large.
“When you unlock a lot of data, you create profiles of organisations, in our case commercial businesses,” says Peake. “Along with some of the consortium members, I was very concerned that, quite often, you could unlock data that an insurer did not have, and, in our process, for example, present a profile of an organisation as riskier than the insurer initially thought.”
A big area of focus for the Innovate UK project was to make sure that AI does not discriminate against smaller companies in particular. For large businesses, insurers often look past potential risk as they are guaranteed reliable returns, but this often does not extend to SMEs.
And so, with insurance professional Neil Strickland, he set about creating a solution that made sure insurers did see the full picture, using AI, data analytics and satellite image analysis to help deliver more accurate real-time commercial property underwriting and risk management – an improvement that, crucially, could save lives.
Intelligent AI plugs the knowledge gaps by taking a ‘digital twin’ approach to generating a statement of value (SoV), the file of information, often compiled manually, that traditionally underpins risk assessments – and which, according to Intelligent AI, often only contains 40 per cent of the available data. The insurtech uses AI to first cleanse, then analyse and compile data from more than 300 sources to create a virtual 3-D mirror image of a property. This visual representation of a SoV not only provides an assessment of the precise property, but also of the surrounding area in which it sits.
Such an approach to information gathering means that autonomous machines are surfacing and making judgement calls on ever more granular data in complex situations. And, while better data is of obvious benefit in terms of preventing loss of life and reducing the liability on insurers’ books, it potentially raises questions over its ethical collection and use. That’s a judgement call Anthony Peake is well aware of, which is why he’s currently working with Innovate UK on its ethical AI project.
You could unlock data that an insurer did not have, and present a profile of an organisation as riskier than the insurer initially thought
With growing reliance on immediate automated services, AI is fast becoming an integral part of insurance, but in parallel to its growing presence is the call for more robust regulation and
Unintended consequences
Though the road to unbiased claims processing is paved with good intentions,
regulators underestimate the sophistication of this feat. Algorithms work by locating patterns, and they are slow in taking individual context into account. One case, in private real estate, which was investigated in a study by the University of California, Berkeley, illustrates the point. It found that black American homeowners were being charged mortgage rates of interest that were, on average, 5.3 basis points higher than those of their white counterparts. The algorithmic lenders studied matched rates of interest to location, not a race, so the resulting bias was indirect, but the unfair impact in the real world was obvious. And it illustrates the challenge involved in monitoring algorithms.
The current insurance landscape is being propelled by multiple forces – both internal and external – towards the adoption of AI-backed risk management. But there is an urgent need to address any biases hidden in legacy datasets used to train predictive models, otherwise policyholders and wider society will begin to question whether their claims are being treated fairly, consistently and honestly. Insurers are aware of the danger and have taken steps to combat it. In the States, insurer Lemonade has proposed a solution through uniform loss ratio (ULR). When AI identifies a pattern among a demographic, it can highlight it, not as data on which to base claims, but as an unfair anomaly to avoid. Enough of this data will accumulate to then be comprehensive enough that the process cannot discriminate against that demographic. Lemonade uses the analogy of the rate of police arrests and how the algorithm would correct this: “As data accumulates, the ‘been arrested’ group would subdivide, because the AI would detect that, for certain people, being arrested is less predictive of future claims than for others. The algorithm would self-correct, adjusting the weighting of this data to compensate for human bias.”
While Lemonade is modifying its algorithms to unlearn biases, Aviva is providing policyholders with the option to have either machine or human-led claims processing. So, insurers are finding ways to balance AI to deliver large-scale, transparent services. Meanwhile, the Solvency II directive also insists insurers make their AI-based systems and performance results as digestible as possible for consumers, be that in risk management or claims processing.
Peake says the industry must understand that ‘if you take old data and build new models, you will end up with old biases on a large scale’, but that can be addressed.
“In the ethical framework developed with Innovate UK, we have ensured companies need to be fully aware of such bias and account for this in the training data. Also, that people have a right to query the results, have a clear point of contact, and a simple way to ask for corrections.”
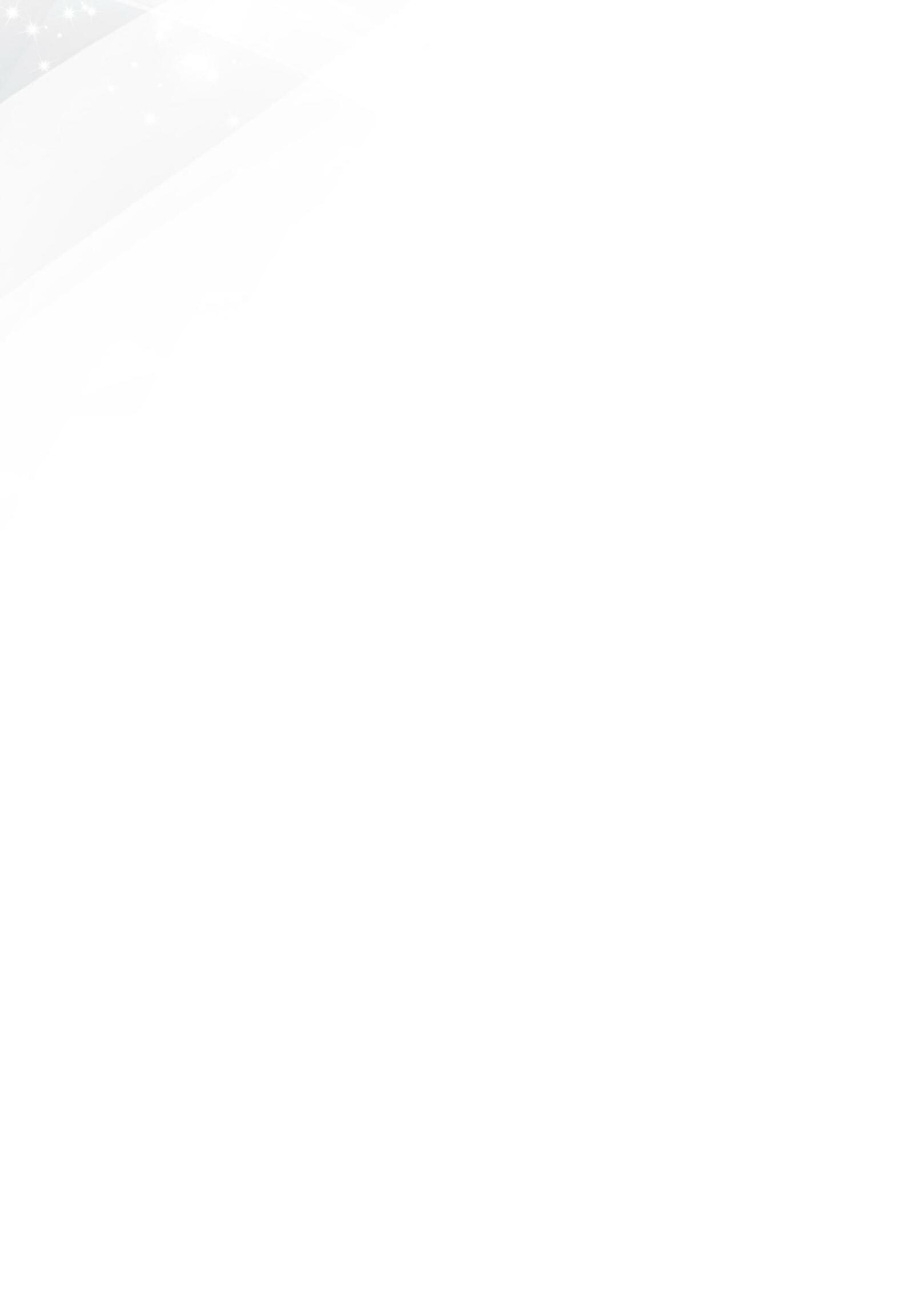
A tragedy waiting to happen:
Better insight into data might have helped at Grenfell Tower
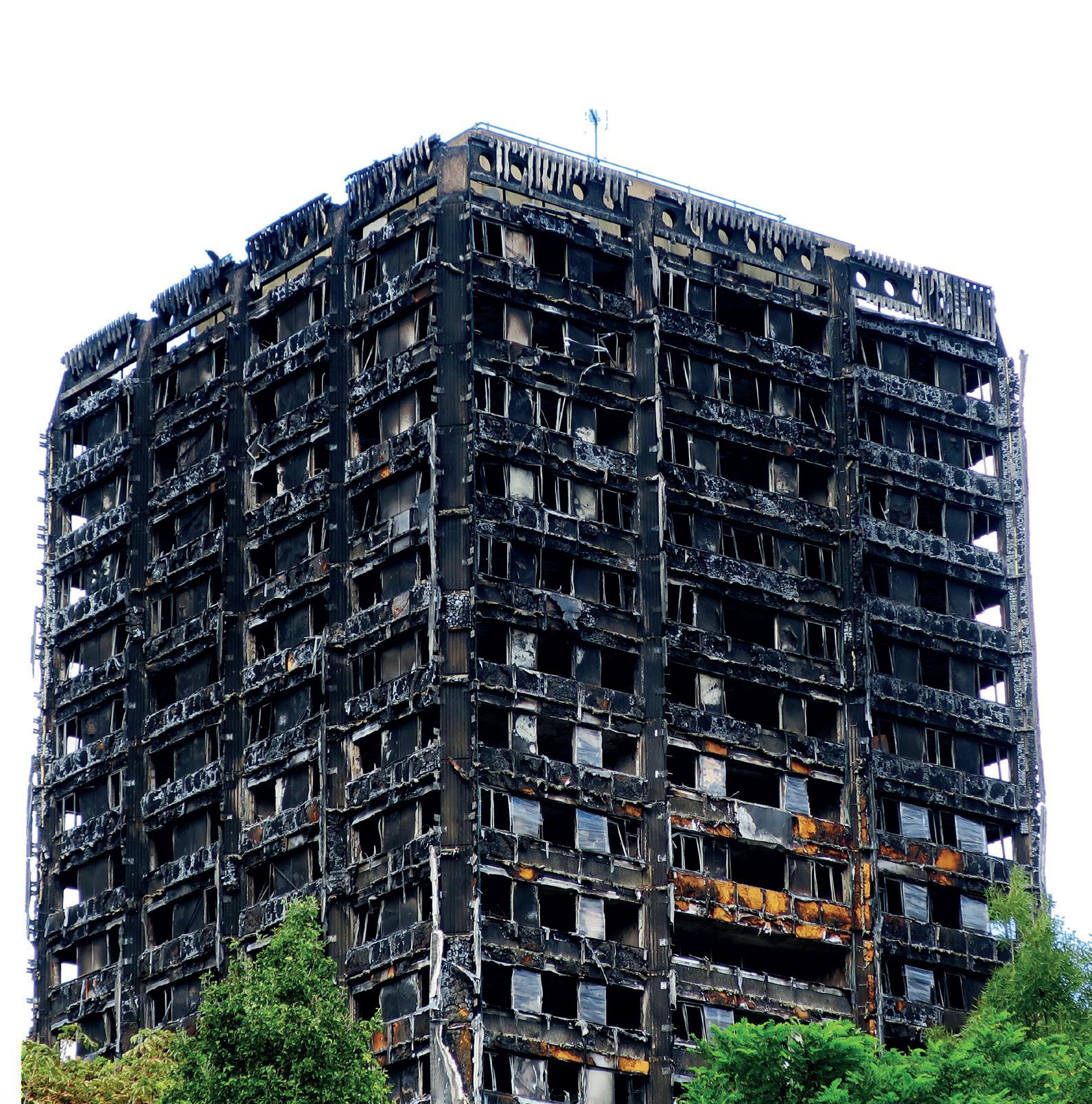