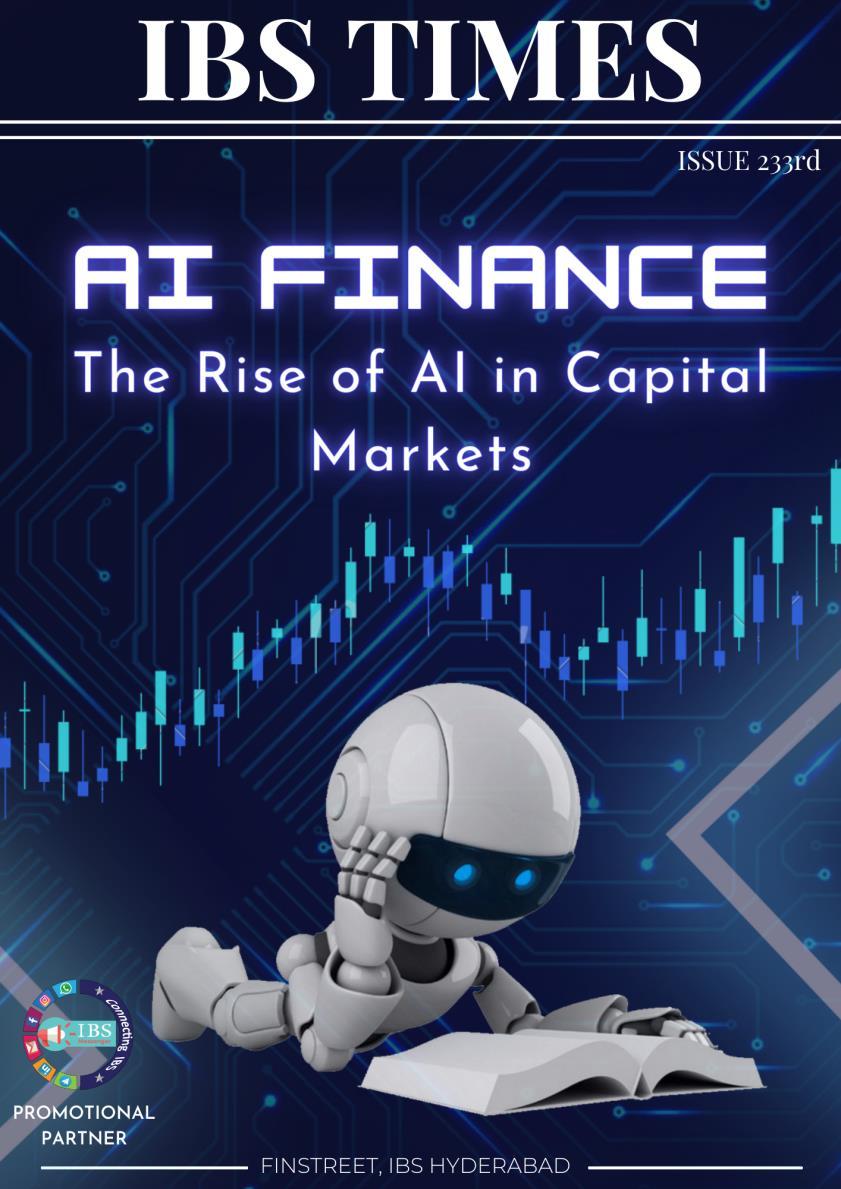
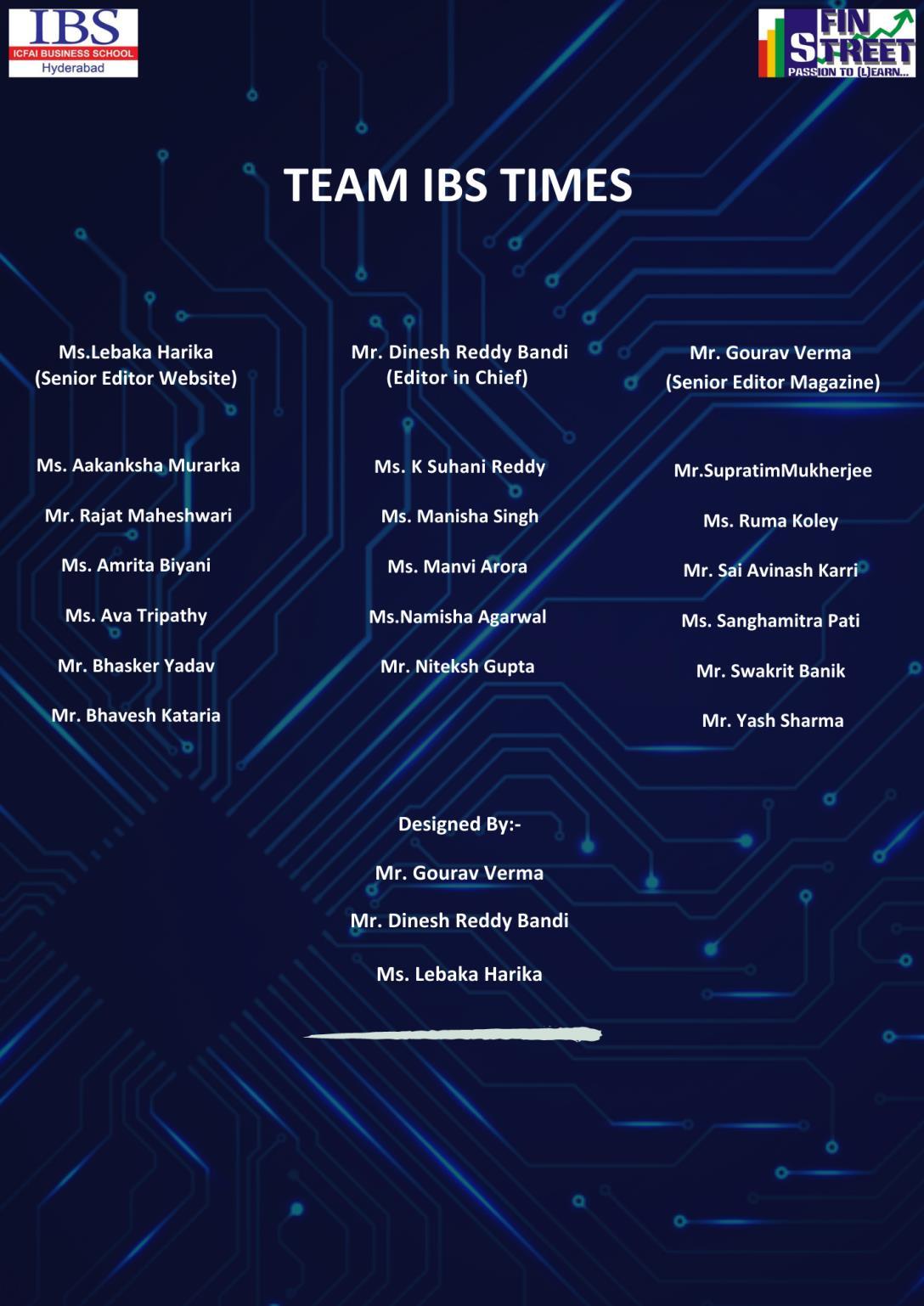
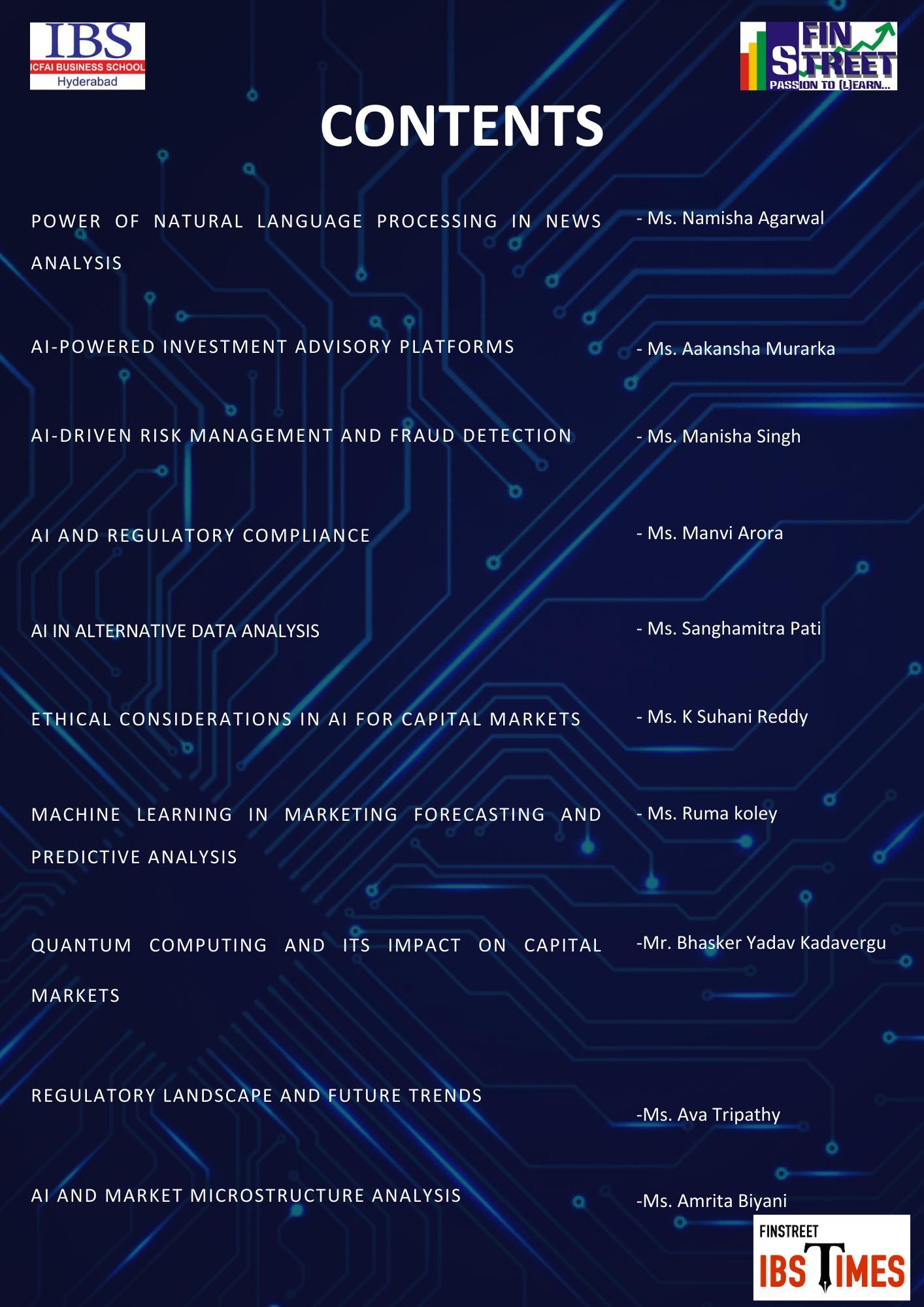
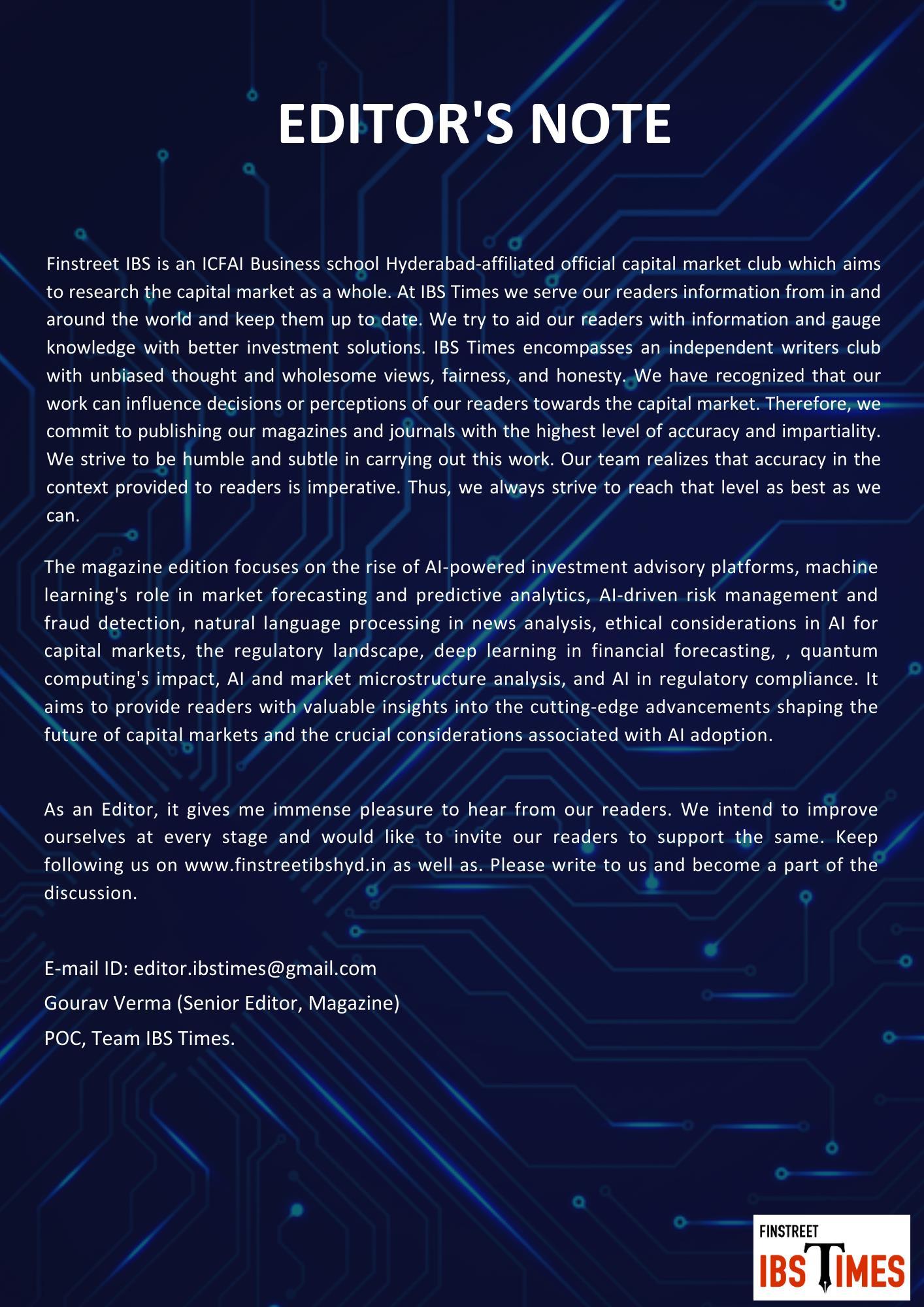
In today's fast-paced and interconnected world, news plays a vital role in shaping stock market trends and investor sentiment. However, the sheer volume of news articles and reports can be overwhelming for investors to analyse effectively. This is where artificial intelligence (AI) and natural language processing (NLP) techniques come into play. NLP, a branch of AI, empowers investors with the ability to extract valuable insights from news data, understand market sentiment, and make informed decisions. In this article, we will explore the power of NLP in news analysis and its transformative impact on the stock market
Natural Language Processing is a field of AI that focuses on the interaction between computers and human language. It enables machines to understand, interpret, and generate human language in a way that is meaningful and useful. NLP algorithms employ various techniques, such as text parsing, semantic analysis, entity recognition, and sentiment analysis, to extract information from unstructured textual data. In the context of stock market news analysis, NLP algorithms can process vast amounts of news articles, blogs, social
media feeds, and company reports. They can identify key entities (companies, individuals, and events) and analyse the sentiment associated with them. By understanding the context and sentiment expressed in news data, NLP algorithms can provide valuable insights to investors, helping them make more informed investment decisions.
The sheer volume of news data available can make it challenging for investors to keep up
with relevant information and identify its impact on the stock market. NLP techniques help address this challenge by automating the process of analysing news data and extracting key insights. One key aspect of NLP in news analysis is entity recognition. By automatically identifying and categorizing entities such as companies, people, and events mentioned in news articles, NLP algorithms can provide a comprehensive overview of the news landscape. This helps investors track news related to specific companies or industries and identify emerging trends or potential market-moving events.
Sentiment analysis is another powerful application of NLP in news analysis. By analysing the sentiment expressed in news articles, NLP algorithms can determine whether the sentiment is positive, negative, or neutral toward a particular entity. This allows investors to gauge market sentiment and understand how news events may influence stock prices. For example, news articles reporting positive developments for a company may indicate potential growth opportunities, while negative sentiment may suggest risks or challenges.
One of the significant advantages of NLP in news analysis is its ability to provide realtime insights and market relevance. Traditional methods of news analysis often rely on manual reading and interpretation,
which can be time-consuming and may result in delayed responses to market events. NLP algorithms, on the other hand, can process news data in real time, providing investors with up-to-date insights. By continuously monitoring news feeds and social media platforms, NLP algorithms can identify breaking news, market rumours, and significant events that may impact stock prices. This real-time analysis allows investors to react promptly to market developments and adjust their investment strategies accordingly. Additionally, NLP algorithms can filter news articles based on their relevance to specific companies or industries. This ensures that investors receive news updates that are most relevant to their investment portfolios. By eliminating noise and focusing on the most pertinent information, NLP algorithms enable investors to stay informed about news that directly affects their investment decisions.
NLP in news analysis goes beyond providing real-time insights and sentiment analysis. By analysing a vast amount of news data over time, NLP algorithms can uncover hidden patterns and market trends that may not be apparent to human analysts. For example, NLP algorithms can identify recurring themes or topics in news articles related to specific companies or industries. This analysis can help investors understand the key drivers of stock market performance and
anticipate future trends. By identifying correlations between news events and stock price movements, NLP algorithms can uncover valuable insights that may inform investment strategies. Furthermore, NLP techniques can analyse news data across different languages and geographical regions. This enables investors to gain a global perspective on market trends, international news events, and geopolitical factors that may impact stock markets. By considering a broader range of news sources, NLP algorithms help investors make more comprehensive and informed decisions.
Integrating NLP in news analysis has significantly enhanced the way investors make investment decisions. By providing real-time insights, sentiment analysis, and uncovering hidden patterns, NLP algorithms empower investors with a wealth of information to inform their investment strategies. Here are some key benefits of NLP in investment decision-making:
1. Improved Efficiency: NLP algorithms can process and analyse vast amounts of news data in a fraction of the time it would take for human analysts to do so manually. This significantly improves the efficiency of news analysis and allows investors to respond promptly to market events.
2.Enhanced Accuracy: NLP algorithms provide objective and data-driven insights by removing human biases and emotions from the analysis. This enhances the accuracy of
decision-making and reduces the risk of making investment choices based on subjective opinions.
3.Risk Mitigation: By analysing sentiment and identifying potential risks or challenges, NLP algorithms help investors proactively manage the risks associated with their investment portfolios. Timely identification of negative sentiment or adverse news can prompt investors to adjust their positions or implement risk mitigation strategies.
4.Identifying Opportunities: NLP algorithms can identify emerging trends, positive news developments, or undervalued stocks that may present investment opportunities. By scanning a vast array of news data, NLP helps investors stay ahead of the curve and capitalize on favourable market conditions.
The power of Natural Language Processing in news analysis has transformed the way investors approach the stock market. By leveraging NLP algorithms, investors can extract valuable insights from news data, understand market sentiment, and make informed investment decisions.. However, it is important to acknowledge the limitations of NLP algorithms. They are dependent on the quality and reliability of the data they process, and there may be challenges in accurately capturing the nuances of human language. Nonetheless, as NLP techniques continue to advance and improve, their impact on the stock market is set to grow, empowering investors with actionable insights and a deeper understanding of market dynamic.
In recent years, artificial intelligence (AI) has emerged as a game-changer in various industries, and the world of stock markets is no exception. With the ability to process vast amounts of data, identify patterns, and make predictions, AI-powered investment advisory platforms have revolutionized the way investors approach the stock market. These platforms leverage advanced algorithms and machine learning techniques to provide investors with data-driven insights and personalized recommendations, enabling them to make more informed investment decisions. In this article, we will explore the transformative impact of AIpowered investment advisory platforms and how they are reshaping the stock market landscape.
One of the key strengths of AI-powered investment advisory platforms lies in their ability to process and analyse vast amounts of data. Traditional investment strategies often rely on human analysts to sift through financial reports, news articles, and market trends. However, the human brain has limitations when it comes to processing such a massive volume of information. AI algorithms, on the other hand, can rapidly analyse data from diverse sources, including social media, news feeds, economic indicators, and historical stock prices. By incorporating this wealth of information, AIpowered platforms can generate valuable insights and identify hidden patterns that may elude human analysts.
Machine learning plays a crucial role in the functionality of these platforms. Through the iterative learning process, AI algorithms can recognize patterns and relationships in the data, continuously improving their predictive capabilities. As these platforms ingest more data and encounter new market scenarios, they become more accurate and reliable in their predictions. This dynamic
learning ability allows investors to access real-time market analysis and receive timely recommendations based on the most up-todate information available.
AI-powered investment advisory platforms employ sophisticated analytics and predictive modelling techniques to generate investment recommendations. These platforms leverage techniques such as natural language processing (NLP) to analyse news articles, social media sentiment, and company reports. By extracting key insights from textual data, AI algorithms can assess market sentiment, identify emerging trends, and evaluate the impact of specific events on stock prices. Moreover, these platforms can employ data visualization tools to present complex information in a user-friendly manner. Interactive charts, graphs, and dashboards allow investors to understand market trends, historical performance, and the potential risks associated with specific investment opportunities. This visual representation helps investors make informed decisions by providing a comprehensive overview of the stock market landscape.
One of the significant advantages of AIpowered investment advisory platforms is their ability to deliver personalized investment strategies. These platforms consider an investor's risk tolerance, investment goals, and preferences to generate tailored recommendations. By analyzing historical data and the investor's portfolio, AI algorithms can provide insights on asset allocation, diversification, and rebalancing strategies. Furthermore, AIpowered platforms can adapt to an investor's changing circumstances and preferences. As investors gain more experience or adjust their risk appetite, these platforms can dynamically adjust the recommendations to align with the investor's evolving needs. This personalized approach helps investors build and manage their portfolios in a more efficient and effective manner, optimizing their chances of achieving their financial goals.
Investing in the stock market can be daunting, especially for novice investors. The fear of making costly mistakes or missing out on potential opportunities often discourages individuals from entering the market. AIpowered investment advisory platforms address these concerns by providing investors with a sense of confidence and security. By leveraging AI algorithms, these platforms can offer data-backed recommendations, reducing the element of emotional bias that often affects investment decisions. Moreover, the transparency and
explainability of AI algorithms enable investors to understand the reasoning behind specific recommendations. This level of transparency builds trust and empowers investors to make informed decisions based on logical analysis rather than pure speculation.
ensuring that they operate within the bounds of existing regulations and provide transparency to investors.
4.Human Oversight: While AI-powered platforms offer valuable insights, human oversight remains essential. Investors should not blindly follow the recommendations provided but should consider them as part of their decision-making process. Human judgment, experience, and intuition continue to play a crucial role in successful investing.
While AI-powered investment advisory platforms hold significant promise, it is important to acknowledge some challenges and considerations associated with their adoption. Here are a few key points to consider:
1. Data Quality and Reliability: The accuracy and reliability of AI algorithms heavily depend on the quality and integrity of the data they process. Ensuring the availability of high-quality data from trustworthy sources is crucial to maintaining the effectiveness of these platforms.
2.Ethical Considerations: As AI-powered platforms make autonomous investment decisions, ethical concerns arise. It is essential to establish robust governance frameworks to ensure that these platforms adhere to ethical standards and avoid any discriminatory or unethical behaviour.
3.Regulatory Compliance: The use of AI in the financial industry is subject to various regulatory frameworks. Regulators need to address the challenges associated with AIpowered investment advisory platforms,
AI-powered investment advisory platforms have emerged as a transformative force in the stock market, revolutionizing the way investors approach investment decisions. By harnessing the power of data, advanced analytics, and machine learning, these platforms provide investors with data-driven insights, personalized recommendations, and enhanced confidence. However, it is important to acknowledge the challenges and considerations associated with the adoption of these platforms, including data quality, ethical considerations, regulatory compliance, and the need for human oversight. As the capabilities of AI continue to advance, AI-powered investment advisory platforms are expected to become increasingly sophisticated and influential in the stock market landscape. The integration of AI into investment strategies has the potential to unlock new opportunities, improve decision-making, and empower investors to achieve their financial goals in a more efficient and effective manner.
The stock market is a complex and everchanging environment, making it susceptible to fraud and other forms of financial crime. Artificial intelligence (AI) has become an effective tool for risk management and fraud detection in the stock market in recent years. By processing massive volumes of data more rapidly and effectively than humans can, AI-powered solutions can aid in the detection and prevention of fraud. Additionally, they can use past data to discover fraudulent behaviour patterns that might be challenging to find using more conventional techniques.
evaluate, and reduce a variety of risks related to trading and investing.
Some of the key aspects of AI-driven risk management in the stock market are:
Artificial intelligence-driven risk management in the stock market uses algorithms and strategies to recognize,
1.Risk Identification: To find potential threats, AI systems examine massive amounts of historical and real-time data. Analysing market patterns, previous price changes, trade volumes, news moods, and other pertinent data sources are part of this process. AI can assist in spotting possible risks, such as market volatility, liquidity issues, or sector-specific hazards, by spotting patterns and anomalies.
2.Risk Assessment: AI models evaluate the scope and potential effect of threats that have been discovered. They can measure risk exposures, calculate prospective losses, and assess the likelihood of various risk scenarios. Advanced statistical and machine learning methods are used by AI algorithms to analyse historical data and estimate the risk based on patterns and correlations.
3.Portfolio Optimization: Investment portfolios can be optimized by AI to properly control risks. AI algorithms can provide the best asset allocation plans by taking into account risk tolerance, return goals, and
other constraints. These algorithms can recommend rebalancing measures depending on shifting market conditions and assist in diversifying portfolios to lower concentration risk.
4.Scenario Analysis: Scenario analysis is a step in AI-driven risk management that assesses the probable effects of particular occurrences or market circumstances. AI systems can predict how investments might perform in challenging circumstances by modelling various scenarios and stresstesting portfolios. This aids in the decisionmaking process and allows risk managers and investors to gauge the strength of their portfolios.
regulatory risk management. It can assist in spotting potential offenses like insider trading or market abuse and make timely reporting and regulatory compliance easier.
The goal of AI-driven risk management in the stock market is to improve decision-making procedures, raise the reliability of risk assessments, and facilitate proactive risk mitigation. It's crucial to remember that AI models cannot replace human expertise and judgment and should only be used in conjunction with it. AI-driven insights, human interpretation, and adherence to regulatory frameworks must all be used in conjunction for effective risk management.
Artificial intelligence (AI)-driven fraud management in the stock market makes use of artificial intelligence tools and algorithms to find, stop, and reduce fraudulent acts that take place in the market. The following are some crucial components of AI-driven stock market fraud management:
5.Dynamic Risk Monitoring: AI regularly analyses important indicators and real-time market data to evaluate shifting risk situations. Based on market volatility, changes in liquidity, or other risk indicators, it can dynamically update risk models and tactics. AI algorithms can produce alerts and suggestions for proactive risk management.
6.Compliance and Regulatory Risk Management: By keeping an eye on and analysing trading operations to make sure that regulatory standards are being followed, AI can help with compliance and
1.Fraud Detection: To find trends and anomalies that can point to fraudulent behaviour, AI systems examine vast amounts of data, including trading activities, market data, news, and social media. These
algorithms can identify a variety of scams, including front-running, pump-and-dump schemes, insider trading, market manipulation, and unlawful trading.
2.Anomaly Detection: By examining historical data and market patterns, AI models create a baseline of typical behaviour. Any departures from this norm, such as unusual trade volume, strange price changes, or surprising correlations, might be recognized as anomalies that might point to criminal activity.
3.Pattern Recognition: AI algorithms can find intricate correlations and patterns in trading data to expose shady business practices. For instance, they can identify coordinated trading patterns, unusual order placements, or recurring trading patterns that might point to collusion or market manipulation.
4.Sentiment Analysis: AI can assess market sentiment and find potential fraud-related information by analysing news articles, social media messages, and other textual data. Artificial intelligence (AI) systems can spot growing fraud threats or market rumours that could have an impact on investors by tracking conversations and moods surrounding particular stocks or firms.
5.Network Analysis: To identify suspect networks or insider trading operations, AIdriven fraud management examines the links and interconnections between market participants. AI algorithms can locate concealed connections or out-of-theordinary connections that might signify fraudulent behaviour by looking at transactional data, account ties, and communication patterns.
6.Real-Time Monitoring: To quickly identify and stop fraudulent operations, AI systems can continually monitor real-time trading activities, order book data, and market circumstances. Real-time monitoring makes it possible to quickly spot erratic price fluctuations, suspicious order activity, and suspicious trading patterns.
7.Compliance and regulatory compliance: By keeping an eye on trading activity for conformity to legal and regulatory regulations, AI can help with compliance with regulatory obligations. AI algorithms can facilitate timely reporting and compliance by identifying potential violations, such as non-compliant trades, late registrations, or violations of insider trading laws.
8. Risk Scoring and Prioritization: AI-driven fraud management systems can rank trading operations or market participants according to the likelihood that they would engage in fraudulent activity. This aids in resource allocation and investigational prioritysetting.
The goal of AI-driven fraud management in the stock market is to improve fraud
detection and prevention, protect investor interests, and uphold market integrity. To understand the results, look into cases that have been flagged, and take appropriate action, human skill and oversight are still necessary, even though AI algorithms might offer useful insights. Effective fraud prevention also depends on regulatory frameworks and cooperation between market actors and regulatory organizations.
:-
The use of AI in risk management and fraud detection in the stock market has a number of advantages. These consist of:
process, which can help to save time and money.
d) Improved Decision-making: It is improved by AI-powered solutions since they offer information about the likelihood of fraud. Better investment choices can be made using this information, lowering the likelihood of suffering monetary losses.
a)Increased Accuracy: AI-powered fraud detection solutions have the potential to be more accurate than older technologies. This is because AI can uncover patterns of fraudulent behaviour that would be challenging to detect using conventional techniques.
b)Reduced false positives: AI-driven solutions can lower the incidence of false positives. This is crucial since false positives may trigger pointless inquiries and disturbances.
c)Increased Efficiency: AI-powered solutions can aid in automating the fraud detection
of AI-Driven Risk Management and Fraud Detection :-
While there are a several benefits to using AI for risk management and fraud detection, there are also some challenges that need to be addressed. These include:
1.Data accessibility: For training and efficient operation, AI-powered systems need a lot of data. It might be challenging to collect this information, particularly when it comes to fraud prevention.
2. AI Biasness: Artificial intelligence (AI) algorithms are subject to bias, which may result in false positives or false negatives. Numerous elements, including the data used to train the algorithm and the system's design, may contribute to this bias.
3.Explainability: Because AI algorithms can be challenging to comprehend, it might be challenging to understand why they do particular actions. Regulators and law enforcement organizations may find it difficult to comprehend how AI is being used to spot fraud because of this.
The stock market in India has benefited greatly from the incorporation of AI-driven risk management and fraud detection. By utilizing massive volumes of data and cutting-edge analytics approaches, AI algorithms have revolutionized risk assessment, portfolio optimization, and fraud detection. Investors, regulators, and market players may now make well-
informed decisions and take preventative action thanks to the capacity to identify possible hazards, model situations, and detect fraudulent activity in real time.
However, issues with data quality, system integration, and ethical considerations still exist. Accurate risk assessments and fraud detection depend on the availability of trustworthy data for training AI models. It takes meticulous preparation and execution to integrate AI technologies into current systems and ensure their ethical use. Additionally, the regulatory framework must keep up with technical developments, and effective risk management and fraud prevention depend on cooperation between market participants, regulators, and AI developers.
AI-driven solutions will become more crucial as the Indian stock market develops in terms of risk management and upholding market integrity.
AI-driven risk management and fraud detection will help build a more stable and secure stock market ecosystem in India with sustained innovation, cooperation, and responsible implementation.
In today's data-driven world, investment firms and financial institutions are constantly seeking new and innovative ways to gain a competitive edge. Traditional financial data, such as company financial statements and economic indicators, has long been the backbone of investment analysis.
However, with advancements in technology and the proliferation of digital information, alternative data sources are emerging as a game-changer in investment decisionmaking. By harnessing the power of artificial intelligence (AI) techniques, alternative data analysis offers the potential to generate unique insights that can significantly enhance investment strategies.
Alternative data refers to non-traditional sources of information that are not typically found in financial statements or economic reports. These sources encompass a wide range of data, including satellite imagery, social media data, sensor data, web scraping, credit card transactions, and more. What sets alternative data apart is its ability to provide a granular, real-time view of various aspects of the economy and individual companies. This wealth of information can be a goldmine for investors looking to uncover hidden trends, validate investment theories, and identify emerging opportunities or risks.
1. Satellite Imagery: Satellite imagery allows investors to monitor physical assets, track global supply chains, assess retail foot traffic, and even estimate crop yields. Highresolution images combined with AI algorithms enable investors to gain unique insights into economic activity and make more informed investment decisions. Companies like Orbital Insight and Descartes Labs have leveraged AI algorithms to analyse satellite images of parking lots, retail stores,
and industrial facilities to estimate consumer foot traffic, monitor inventory levels, and gauge economic activity. By applying machine learning techniques, these
companies can derive valuable insights and predict key performance indicators such as sales growth or quarterly earnings before official reports are released. Such information can give investors an edge in adjusting their positions or making informed decisions ahead of the market.
2. Social Media Data: Social media platforms generate vast amounts of data, encompassing consumer sentiment, product reviews, brand mentions, and more. Platforms like Twitter, Facebook, and Instagram generate an enormous amount of user-generated content every second. Sentiment analysis, natural language processing, and topic modelling algorithms can analyse this data to gauge public sentiment, track consumer preferences, and uncover emerging trends.
decisions about potential investments in related companies.
3. Sensor Data: Sensor data, derived from Internet of Things (IoT) devices, is yet another type of alternative data that has gained prominence in recent years. IoT devices are embedded with sensors that collect and transmit data on various parameters such as temperature, humidity, energy consumption, and more. This data can be harnessed to gain insights into supply chains, industrial production, and energy consumption patterns.
For instance, monitoring social media discussions about a particular product or brand can help investors assess consumer sentiment and predict future sales performance. Additionally, social media data can provide valuable insights into the success or failure of marketing campaigns, allowing investors to make more informed
By leveraging AI techniques such as anomaly detection and predictive modelling, investors can identify potential disruptions or opportunities in specific sectors or companies. For example, analysing energy consumption patterns of manufacturing facilities can provide insights into production levels and help investors gauge the financial health of industrial companies.
The integration of AI techniques is instrumental in transforming alternative data into meaningful insights. AI algorithms,
such as machine learning, deep learning, and natural language processing, enable investors to extract valuable information, detect patterns, and uncover hidden relationships within complex datasets. Here are some ways AI is revolutionizing alternative data analysis:
news articles, and extract valuable information from unstructured text sources.
:-
By harnessing the power of AI and alternative data analysis, investors can unlock unique insights that have the potential to generate alpha and inform investment strategies. Here are a few examples of how AI in alternative data analysis is being leveraged:
1. Data Cleaning and Pre-processing: Alternative datasets often come with noise, missing values, or inconsistencies. AI algorithms can automate data cleaning and pre-processing tasks, saving time and ensuring data accuracy.
2. Pattern Recognition: AI algorithms excel at recognising patterns within massive datasets. By applying machine learning techniques, investors can uncover hidden correlations and identify signals that impact investment performance.
3. Predictive Analytics: AI-powered predictive models leverage historical data from alternative sources to forecast future trends and generate investment insights. These models continuously learn and adapt, enhancing their accuracy over time.
4. Natural Language Processing: Sentiment analysis and text mining using natural language processing techniques enable investors to gauge public sentiment, analyse
1. Quantitative Investment Strategies: Alternative data sources combined with AI techniques enable investors to develop quantitative models that exploit market inefficiencies, identify trading opportunities, and optimise investment portfolios.
2. ESG Investing: Environmental, Social, and Governance (ESG) factors have gained significant importance in investment decision-making. AI algorithms can analyse alternative data to assess a company's ESG performance, allowing investors to integrate sustainability considerations into their strategies.
3. Risk Management: Alternative data analysis can enhance risk management capabilities by providing real-time insights into various risk factors. By monitoring alternative datasets, investors can proactively manage risks related to supply chain disruptions, regulatory changes, or reputational issues. The utilisation of alternative data sources combined with AI techniques has ushered in a new era of
investment strategies. By integrating diverse datasets, such as satellite imagery, social media data, and sensor data, investors can gain unique insights that were previously inaccessible through traditional data sources. AI algorithms enable the extraction of valuable information, the detection of patterns, and the prediction of future trends, empowering investors to make more informed decisions.
reliability. Not all alternative data sources are equally reliable, and inaccurate or biased data can lead to flawed insights and misguided investment decisions. Ensuring data accuracy and reliability requires rigorous data cleansing, normalisation, and validation processes.
Additionally, privacy concerns and regulatory compliance are crucial considerations when dealing with alternative data sources, especially social media data. Investors must navigate the legal and ethical landscape to ensure they are using data in a responsible and compliant manner. As AI continues to advance and alternative data sources become more abundant, the potential for generating unique investment insights will only grow. It is imperative for investors to embrace these technological advancements, adapt their strategies, and continuously refine their analytical frameworks to leverage the full potential of AI in alternative data analysis.
Conclusion :-
The benefits of AI in alternative data analysis extend across various aspects of investment strategies. From quantitative investment models that exploit market inefficiencies to ESG integration and risk management, AIdriven insights provide a competitive edge and enhance performance.
However, One of the major hurdles to utilising alternative data is data quality and
The fusion of AI and alternative data analysis represents a paradigm shift in the investment landscape. By harnessing the power of advanced algorithms and diverse datasets, investors can uncover hidden opportunities, mitigate risks, and make more informed decisions. The future holds immense potential for those who embrace this transformative approach, unlocking a world of untapped possibilities for investment strategies.
"Artificial intelligence is poised to be a gamechanger in regulatory compliance, providing financial institutions with the tools to navigate complex regulatory frameworks and maintain trust in the financial system."
- James Gorman, CEO of Morgan Stanley.Regulatory compliance has emerged as one of the most important factors for financial institutions in today's rapidly evolving economic landscape. Compliance with strict regulations, such as Know Your Customer (KYC) and Anti-Money Laundering (AML) procedures, is necessary not only to fight financial crimes but also to preserve trust and protect the financial system's integrity. Thankfully, regulatory compliance has undergone a significant paradigm shift due to artificial intelligence (AI) development. Financial institutions can use AI technologies, such as machine learning, automation, pattern recognition, and anomaly detection, as practical tools to streamline their compliance efforts, improve
efficiency, and ensure adherence to regulatory requirements.
The conventional method of ensuring regulatory compliance involved labourintensive manual procedures that were time-consuming and prone to human error, such as document verification and transaction monitoring. By utilizing AI algorithms, financial institutions can automate repetitive and routine tasks like data entry, validation, and tracking. Machine learning algorithms can soon analyse enormous volumes of data, including customer profiles, transaction records, and external data sources, to quickly identify suspicious activities, patterns, and potential risks.
Incorporating such cutting-edge technologies is beneficial for current business scenarios because AI and ML are the primary mega-trend drivers of today's business landscape. We will delve into the business transformation by AI in the upcoming eras, starting with reducing labour-intensive work to swiftly carrying out end-to-end processes.
Automation in AML and KYC:
The Know Your Customer (KYC) and AntiMoney Laundering (AML) processes have been revolutionized by automation, improving the effectiveness and efficiency of regulatory compliance in the financial industry.
Financial institutions can more easily identify and track suspicious activity using automation in AML. Machine learning algorithms can analyse large amounts of transaction data, customer profiles, and external data sources to find patterns and anomalies that could point to possible money laundering activities. Automating the procedure allows institutions to identify high-risk customers and transactions more quickly and accurately, lowering the risk of financial crimes. Thanks to automation, which is a critical component of anti-money laundering (AML) processes, financial institutions can effectively identify and stop money laundering activities. Following are some instances of how automation is changing AML:
1.Transaction Monitoring: AML automation uses AI algorithms to quickly and efficiently analyse enormous amounts of transactional
data. Machine learning models can be trained to find patterns and anomalies that might point to possible money laundering activities. For instance, if an account unexpectedly receives a sizable infusion of cash from several sources exceeding a predetermined threshold, an automated system may flag this as potentially suspicious activity, prompting compliance teams to investigate it further.
2.Customer Risk Profiling: Automating automation makes Customer risk profiling more accurate and effective. AI algorithms can assign customer risk scores by examining various data sources, including transaction history, customer behaviour, and external data from news articles and social media sources. For instance, an automated system may identify customers as high-risk if they display unusual transaction patterns or have ties to high-risk jurisdictions, invoking increased due diligence procedures.
3.Suspicious Activity Reporting: Automation makes creating suspicious activity reports (SARs) easier. Systems with artificial intelligence (AI) can examine transactional data, customer profiles, and other pertinent data to spot potentially suspicious activity. Financial institutions can ensure accurate and timely reporting to regulatory authorities by automating the SAR generation process, which will increase compliance with reporting requirements.
4.Data analysis and forensic investigation: In AML processes, automation makes it possible to carry out these tasks effectively. Large volumes of data, including customer profiles, transaction histories, and external
sources, can be quickly combed through by AI algorithms to find potential connections, relationships, and hidden patterns that could point to money laundering activities. This automated analysis lets Investigators prioritize and concentrate on the most critical and high-risk cases. In the finance industry, automation is becoming increasingly common, especially in Anti-Money Laundering (AML) processes, which are essential for improving efficiency and effectiveness.
Here are a few actual instances of automation being used in AML in the financial industry:
a. JPMorgan Chase& Co : To increase productivity and lower false positives, JPMorgan Chase, one of the most important financial institutions in the world, has automated its AML procedures.
b. HSBC: To improve the efficacy of transaction monitoring, the multinational banking and financial services company HSBC has automated its AML operations. Using AI-powered algorithms,
HSBC automated the analysis of customer behaviour and transaction data, making it easier to spot potential money laundering schemes. The bank's ability to identify suspicious patterns and anomalies has improved thanks to automation, which has improved compliance and risk mitigation.
c. Standard Chartered: To speed up its customer due diligence procedures, the international banking organization Standard Chartered has automated its AML efforts.
JPMorgan Chase automated its transaction monitoring system to analyse massive amounts of data and, more precisely, spot suspicious activity by utilizing machine learning algorithms. As a result of this automation, there were fewer false positive alerts, which freed up compliance teams to concentrate on cases with a higher priority that needed human investigation.
Standard Chartered automated checking of customer data against regulatory databases and sanctions lists using AI technologies. With the help of automation, the bank can quickly spot any matches with entities or people who pose a high risk to sanctions compliance and take the necessary action.
Similarly to this, automation is essential to KYC procedures. KYC entails confirming customers' identities and evaluating the risks they pose. Financial institutions can use automation to compare customers' data with various data sources, such as public records and social media profiles. With the aid of this automation, institutions can spot discrepancies or inconsistencies in the information given, potentially enabling them to spot fraud or regulatory non-compliance. By automating these verification processes, institutions can speed up customer onboarding while complying with regulatory requirements.
Here are some examples of how automation is being used in KYC:
1.Digital identity verification: By utilizing cutting-edge technologies like optical character recognition (OCR) and biometric authentication, automation enables financial institutions to streamline the verification of customer identities. For instance, AI-powered systems can automatically extract pertinent data from identification documents, compare it to data from reliable sources, and assess the documents' authenticity. Through automation, manual data entry is done away with, errors are decreased, and the customer onboarding process is sped up.
2.Risk Assessment and Scoring: By examining various data sources, such as transaction history, credit scores, and external data feeds, automation makes it easier to assess customer risk levels effectively. Customers can receive risk
scores from AI algorithms based on preestablished rules or machine learning models.
For instance, if a client has a history of loan defaults or is connected to high-risk jurisdictions, an automated system may flag them as higher-risk people, resulting in the need for increased due diligence.
3.Regulatory Reporting: Automation makes producing the regulatory reports that different authorities demand easier. AIpowered systems can gather and arrange the required data, ensuring reporting accuracy and consistency. By automating this process, financial institutions can efficiently meet reporting deadlines and lower the risk of non-compliance.
4.Enhanced Customer Experience: By cutting down on the time and effort needed for onboarding, automation in KYC processes enhances the overall customer experience. Customers can quickly complete the KYC process by electronically submitting their information thanks to automated data collection and verification. By reducing friction during the onboarding process and improving customer satisfaction, this automation helps financial institutions better attract and keep customers.
Know Your Customer (KYC) processes in the finance industry have been transformed by automation, which has completely changed how financial institutions confirm customer identities and evaluate related risks.
Here are a few actual cases of KYC applications for automation:
a. Citigroup: A major international banking organization, Citigroup, has improved its KYC procedures by utilizing automation.
streamlined Deutsche Bank's KYC procedures, decreased human error, and ensured compliance with legal requirements.
They have implemented a digital KYC platform that employs AI algorithms to automate risk analysis and document verification. The platform runs automated comparisons with different databases to search for discrepancies or potential threats.
Citigroup's KYC procedures have been streamlined thanks to this automation, which has increased accuracy and efficiency.
b. Deutsche Bank: To increase efficiency and compliance, the global banking and financial services firm, Deutsche Bank, has automated its KYC processes.
About: While AI significantly impacts regulatory compliance, issues, and ethical questions exist. To maintain regulatory compliance, AI algorithm transparency and comprehensibility must be guaranteed. Financial institutions must carefully plan and oversee AI systems to prevent biased or discriminatory outcomes. In addition, handling delicate customer data raises privacy issues. Implementing robust data protection measures and adhering to applicable data privacy regulations are essential.
Conclusion:
They have created a platform powered by AI called "Deutsche Bank KYC Factory" that streamlines customer data acquisition, verification, and administration. The forum starts customer profiles, conducts risk analyses, and runs automated checks against various data sources. This automation has
Financial institutions are benefiting from a revolution in regulatory compliance thanks to AI, making it easier and more efficient to comply with complicated AML and KYC requirements. Artificial intelligence (AI) systems can process enormous amounts of data, spot suspicious activity, and improve customer due diligence through automation, pattern recognition, and anomaly detection. Financial institutions must address challenges and ethical issues even though AI has enormous potential to ensure compliance and preserve public trust. AI has the potential to transform regulatory compliance and bolster the financial sector's capacity to combat financial crimes with careful implementation and oversight
AI (Artificial Intelligence) gives technical systems the ability to comprehend their surroundings, respond to what they see, solve issues, and take appropriate action to reach a target. Technologies with AI and machine learning capabilities are employed in many industries, including healthcare, robotics, science, education, the military, surveillance, finance and regulation, retail, customer service, and manufacturing. The financial markets sector is not an exception to the substantial effects of artificial intelligence (AI)'s rapid breakthroughs on numerous industries. Investment banks, asset managers, and wealth managers increasingly rely on AI to transform their businesses and stay on the cutting edge as digital assets disrupt the financial landscape.
Investment banks, asset managers, and wealth managers are changing their business practices due to the incorporation of AI into the capital markets. AI-driven solutions automate repetitive processes, improve risk management, optimize trading methods, and extract hidden insights from massive data. The market environment is now more effective, sophisticated, and competitive due to this paradigm shift in how businesses approach strategy and decision-making. Investment banks use AIdriven algorithms to streamline processes and carry out trades more quickly and precisely. AI-powered trading algorithms may discover trends and forecast market moves by examining historical data and realtime market information, allowing investment institutions to make better judgments and increase profits.
Additionally, asset managers use AI to sift through vast data and find untapped investment opportunities. Asset managers can process enormous amounts of organized and unstructured data using machine learning techniques, which enables them to spot patterns and trends that would be impossible to spot using conventional
approaches. Ultimately, this improves portfolio performance and lowers risk by allowing asset managers to make better decisions. Wealth managers use AI to give individualized financial advice, automate client communications, and streamline client onboarding procedures. Wealth managers can use AI-driven solutions to analyse customer information, preferences, and risk tolerance to make personalized investment recommendations that align with each client's financial objectives. Virtual assistants and AI-powered chatbots are also being used to automate client interactions, boosting customer satisfaction and reducing operational costs.
Capital market companies are significantly investing in AI and associated technologies like cloud computing and data analytics because they understand how important it is to remain competitive in a continually changing field This development shows how dedicated the sector is to staying on top of things and embracing the disruptive potential of digital assets.
Many capital market organizations have already started investing in new tools and platforms that AI powers in preparation for significant disruption in the financial services industry. Central international banks are devoting substantial resources to technology initiatives like cloud computing, blockchain, and artificial intelligence (AI) to increase their market competitiveness. Many financial institutions prioritize AIdriven solutions to revolutionize their operations and propel future growth; such
investments are not exclusive to just a few organizations. Notably, cloud computing is playing a crucial part in the industry's evolution. It enables businesses to grow operations more effectively and offers the infrastructure to deploy AI applications. Capital market organizations can use the power of AI and data analytics to make better decisions, optimize their operations, and provide more individualized services to their clients by implementing cloud-based solutions.
AI is employed in many financial procedures, such as fraud detection, risk management, and credit ratings, and as a result, it is crucial to the operations that support daily living. Unethical AI undermines system trust and lowers the value of financial services. Given that naturally biased humans create AI, expecting AI to be completely impartial is unrealistic. AI might unintentionally or purposely be narrow. As AI has developed, we are finding more malware every day. High-frequency trading may impact the number of stock market transactions, resulting in a wider bid-ask spread and a greater sense of risk among investors when volatility increases. This might impact investments and lead to hoarding in the context of behavioural finance. The need for
informative signals like dividends may be disturbed, the agency cost will rise, and the information asymmetry between management and investors will worsen. The commodity, options, and currency markets have all experienced comparable upheavals.
Human involvement is frequently used as a fix for AI automation problems. However, a manual fallback is only sometimes the ideal solution because humans are susceptible to systemic prejudice. It is well known that bias exists in systems designed to discern between the faces of persons from various ethnic backgrounds. This may result in the creation of defective products, more difficulties expanding to international markets, and a failure to meet regulatory requirements.
Financial services must continuously update their apps as new use cases and deployment levels increase due to the unquestionable fact that ethical AI is a challenging issue that is constantly changing. A company-wide endeavour should be launched to develop and use ethical AI. To guarantee that ethical practices are incorporated into each step of application development and execution, a top-down commitment is necessary. With such a strategy, it can be easier to lag in the difficulties of creating and upholding ethical AI and run into problems that could have been avoided. Businesses must build teams to identify issues, define and formulate solutions, implement them, track and monitor their progress, and achieve the best results. Executive teams must know the
dangers of creating unethical AI and the potential long-term financial and reputational consequences. However, they must also understand that moral AI is the doorway to innovation, enabling precise and effective financial services that can have a beneficial social impact on all clients, regardless of who or where they are.
Fairness and bias, transparency and trust, accountability, and security, privacy and privacy protection, and social benefit are the six main factors often connected to ethical AI. Even one of the things going wrong could harm both people and corporations. This involves the need for regulatory compliance, financial exclusion, and sluggish innovation and growth.
It is no longer an option to postpone or ignore the problem or to transfer responsibility to the technical, compliance, or legal teams. Regardless of the department, leaders inside organizations must actively strive to address the issues with their applications and take responsibility for the effectiveness of the AI they use.
An internal champion, such as the chief AI ethics officer, should oversee the Responsible AI program. This leader gathers stakeholders, cultivates supporters inside the organization, and provides rules and guidelines to guide the creation of AI systems. However, more than authoritybased leadership is required. No one person is able to process all of the answers to the challenging problems that have been
discovered. Organizations need ownership that incorporates a range of viewpoints to have any natural effect. A powerful way to ensure a range of perspectives is to establish an AI committee that is responsible for the discipline of AI and assists in overseeing the entire program and resolving complex ethical issues like unintended consequences and prejudice. Public relations, compliance, legal, other business divisions, and individuals from different backgrounds and geographic locations should all be represented on the committee.
The age in which AI research was confined to the lab has passed into history for humanity. Modern life is now heavily influenced by the continuous integration technology known as artificial intelligence (AI). People believe that technology can help people make better, safer, more equitable, and informed decisions and that, if AI is appropriately applied, it may have a substantial positive impact on economies and civilization. However, such a promise would only be realized with great caution and dedication. This includes considering how the technology's development and use should be regulated and what degree of legal and ethical monitoring with whom and when is necessary.
Until now, self- and co-regulatory approaches based on current laws and recommendations from corporations, academia, and allied technical groups have successfully restricted harmful AI usage.
These actions will continue to be sufficient in the vast majority of situations, experts say, within the constraints imposed by current governance mechanisms. This does not, however, eliminate the need for government intervention. Instead, this paper urges national and international civil society organizations to contribute significantly to the AI governance discussion. The report specifically points out five areas where the government, civil society, and AI practitioners could assist in defining expectations for applying AI in various situations. Among them are frameworks for general accountability, safety considerations, criteria for human-AI collaboration, and standards for explainability.
Financial services companies may achieve their regulatory requirements, create fair, transparent, and secure systems, and show their continued dedication to protecting their consumers by adhering to the six ethical considerations. But failure to address ethical issues could result in problems down the road. It may eventually end in noncompliance with legislation and lead to the creation of goods and services that exclude clients. While financial services may safeguard and enhance their brand identity and generate consumer trust by including ethical issues in AI development and implementation, customers will be treated properly. We must ensure that AI technologies perform equitably for all users and that privacy rights are honoured when developing and utilizing them
“Predicting the future isn’t magic, it’s artificial intelligence.”
~Dave Waters By:- Ruma koleyMachine learning has revolutionized various industries, and marketing is no exception. By leveraging vast amounts of data and powerful algorithms, machine learning enables marketers to make informed decisions, optimize campaigns, and improve customer experiences. In the dynamic world of marketing, staying ahead of the competition requires leveraging cuttingedge technologies. Machine learning has emerged as a powerful tool that enables marketers to unlock valuable insights from vast amounts of data. By harnessing the capabilities of machine learning algorithms, businesses can revolutionize their forecasting and predictive analysis strategies, leading to improved decisionmaking, optimized campaigns, and enhanced customer experiences.
1. In today's digital age, companies have access to an abundance of data from multiple sources, including customer
interactions, social media, website analytics, and more. Machine learning algorithms excel at processing and analysing this vast amount of data, uncovering valuable insights that were previously hidden. By leveraging these insights, marketers can make datadriven decisions and create more effective strategies.
2. Machine learning algorithms can analyse historical customer data and identify patterns, trends, and correlations that humans may overlook. This allows marketers to forecast customer behaviour with greater accuracy. For instance, predictive models can identify potential churners, estimate customer lifetime value, predict purchase intent, and anticipate product demand. Armed with these forecasts, marketers can tailor their campaigns, offers, and communications to specific customer segments, maximizing the chances of success.
3. One of the key advantages of machine learning is its ability to personalize marketing efforts at scale. By analysing customer data, such as browsing history, past purchases, and demographics, machine
learning algorithms can create detailed customer profiles and segmentations. Marketers can then deliver personalized content, recommendations, and offers to each customer, enhancing their experience and fostering brand loyalty.
4. Machine learning algorithms can analyse and optimize marketing campaigns in realtime. By continuously monitoring campaign performance, machine learning models can identify patterns and adjust targeting, messaging, and budget allocation accordingly. This enables marketers to achieve better ROI by directing resources toward the most effective channels and audiences.
5. Machine learning algorithms can analyse social media conversations, customer reviews, and sentiment data to gauge public opinion and track brand perception. This allows marketers to understand customer sentiment, identify potential brand crises, and address customer concerns promptly. Sentiment analysis also provides valuable insights into emerging trends and customer preferences, helping marketers stay ahead of the competition.
6. Machine learning algorithms can analyse market conditions, competitor pricing, and customer behaviour to optimize pricing strategies. Dynamic pricing models can adjust prices in real-time based on demand, supply, and other factors, maximizing revenue and profitability. Additionally, machine learning algorithms can assist in inventory management by predicting demand fluctuations, minimizing stockouts, and reducing overstock situations.
In the digital age, businesses generate and collect massive volumes of data from various sources, such as customer interactions, online behaviour, purchase history, and social media activity. Machine learning algorithms excel at processing and analysing this data, revealing patterns, trends, and correlations that can drive marketing success. By adopting a data-driven approach, marketers can make informed decisions backed by robust statistical models and predictive analytics.
Machine learning algorithms enable marketers to forecast customer behaviour with unparalleled accuracy. By analysing historical customer data, such as purchase patterns, browsing behaviour, and demographic information, algorithms can identify hidden insights and predict future actions. Marketers can leverage these forecasts to anticipate customer churn, estimate customer lifetime value, optimize cross-selling and upselling strategies, and personalize marketing campaigns to individual preferences that varies
Machine learning plays a pivotal role in delivering personalized marketing experiences at scale. By analyzing vast amounts of customer data, algorithms can create detailed customer profiles and segmentations, enabling marketers to
understand individual preferences, interests, and needs. This granular understanding empowers marketers to craft personalized content, recommendations, and offers, resulting in higher engagement, conversion rates, and customer loyalty.
sentiment data, algorithms can provide valuable insights into customer sentiment, emerging trends, and potential brand crises. Marketers can proactively address customer concerns, make necessary adjustments, and maintain a positive brand reputation in a rapidly evolving digital landscape.
Conclusion:
Machine learning algorithms can continuously monitor and analyse marketing campaign performance, allowing marketers to make real-time adjustments for optimal results. By identifying patterns, trends, and customer responses, algorithms can optimize targeting, messaging, and budget allocation. Marketers can leverage these insights to allocate resources to the most effective channels, refine campaign strategies, and maximize return on investment (ROI).
Perception: Sentiment analysis, powered by machine learning, enables marketers to gauge public opinion and track brand perception across various online platforms. By analyzing social media conversations, customer reviews, and
Machine learning has become a gamechanger in marketing, enabling businesses to leverage data-driven insights, forecast customer behaviour, and personalize marketing efforts. By harnessing the power of machine learning algorithms, marketers can optimize campaigns, enhance customer experiences, and stay ahead in a competitive market. As technology continues to advance, the integration of machine learning in marketing will only grow stronger, paving the way for more effective forecasting and predictive analysis in the future. Machine learning has become a game-changer in marketing, transforming forecasting and predictive analysis. By harnessing the power of machine learning algorithms, marketers can unlock valuable insights, forecast customer behaviour, deliver personalized experiences, optimize campaigns in realtime, and stay ahead in a competitive market. As businesses continue to embrace data-driven strategies, the integration of machine learning in marketing will only grow stronger, enabling marketers to make databacked decisions, enhance customer experiences, and achieve marketing success in the digital age.
Quantum computing has emerged as a transformative technology that holds immense potential for revolutionizing various industries, including capital markets. Capital markets, characterized by vast amounts of data and complex optimization problems, can greatly benefit from the computational power offered by quantum computers. Quantum computing has the ability to solve problems that are beyond the capabilities of classical computers, enabling faster and more efficient solutions to complex optimization, portfolio management, and risk assessment challenges.
mathematics, engineering, economics, and computer science. I'll provide an example of a complex optimization problem and outline a general approach to solving such problems.
Example: Portfolio Optimization
Let's consider a portfolio optimization problem, where an investor aims to allocate funds among different assets to maximize their return while minimizing the risk. The investor has a set of assets available for investment and needs to determine the optimal allocation of funds to each asset.
Define the objective: Start by clearly defining the objective of the optimization problem. In our example, the objective is to maximize the return while minimizing the risk of the portfolio.
Complex optimization problems involve finding the best solution among a vast number of possibilities, considering multiple constraints and objectives. These problems arise in various fields, including
Formulate the problem mathematically: Express the problem as a mathematical model with decision variables, constraints, and an objective function. In our example, the decision variables represent the allocation of funds to each asset, the constraints could include budget constraints, minimum and maximum allocation limits, and the objective function may involve a combination of return and risk measures.
Choose an optimization algorithm: Based on the problem characteristics (e.g., linear/non-linear, continuous/discrete variables, convexity), select an appropriate
optimization algorithm. Various algorithms are available, such as linear programming, mixed-integer programming, quadratic programming, genetic algorithms, or simulated annealing.
the portfolio and identify the assets with the highest allocation.
Refine and iterate: If the results are not satisfactory or the problem changes, refine the mathematical model or algorithm and repeat the optimization process until an acceptable solution is achieved.
Portfolio optimization:
Implement the model: Implement the mathematical model using a programming language or optimization software. Many programming languages provide optimization libraries (e.g., Python's SciPy. Optimize) or specialized optimization software packages (e.g., MATLAB's Optimization Toolbox, Gourbi, CPLEX).
Solve the problem: Use the selected optimization algorithm to solve the problem and find the optimal solution. The algorithm will explore different combinations of variables while respecting the constraints and optimizing the objective function. The solution obtained represents the optimal allocation of funds to each asset in the portfolio.
Evaluate and analyse the results: Examine the solution obtained from the optimization process. Assess the trade-offs between different objectives and constraints. In our example, analyse the risk-return profile of
Portfolio optimization is a technique used to select the best combination of assets that maximize returns while minimizing risk. Artificial intelligence (AI) can be employed to enhance the portfolio optimization process by leveraging advanced algorithms and computational power to analyse large amounts of data and generate optimal portfolios. Here's an example of how AI can be used for portfolio optimization:
Data Collection: Gather historical data for a set of assets, including their prices or returns over a specific period. This data should also include relevant market indices or benchmarks.
Pre-processing: Cleanse and pre-process the data by removing outliers, normalizing the values, and handling missing data. This step ensures the quality and consistency of the dataset
Define Objectives: Specify the objectives of the portfolio optimization, such as maximizing returns, minimizing risk, or achieving a specific risk-return trade-off. This step helps in formulating the optimization problem.
Feature Engineering: Extract relevant features from the dataset that can aid in portfolio optimization. These features can include volatility, correlation, historical performance metrics, or fundamental indicators.
AI Model Selection: Choose a suitable AI model for portfolio optimization. Common approaches include machine learning algorithms like mean-variance optimization, risk-parity optimization, or advanced techniques such as genetic algorithms, neural networks, or reinforcement learning.
Model Training: Train the AI model using the pre-processed dataset. This involves feeding the historical data to the model, which learns the underlying patterns and relationships between the assets. The model can learn to identify optimal asset allocations based on the defined objectives.
Back testing and Evaluation: Test the trained model on historical data that was not used during the training phase. This step evaluates the performance of the model in terms of risk-adjusted returns, consistency, and robustness.
Portfolio Rebalancing: Once the AI model is trained and evaluated, it can be used to rebalance the portfolio periodically. Rebalancing involves adjusting the asset
allocations based on market conditions and the AI model's recommendations.
Monitoring and Iteration: Continuously monitor the performance of the optimized portfolio and iterate on the AI model as new data becomes available. This iterative process helps improve the accuracy and effectiveness of the portfolio optimization strategy over time.
Execution: Finally, implement the optimized portfolio by allocating the recommended weights to each asset. Regularly review and update the portfolio to adapt to changing market conditions or investment goals.
Risk Management:
AI risk management in capital markets involves identifying and mitigating potential risks associated with the use of artificial intelligence (AI) in financial trading and investment activities. Here's an example of how AI risk management can be applied in the context of capital markets:
Example: Algorithmic Trading
Algorithmic trading, also known as automated trading or black-box trading, involves using computer algorithms to execute trades in the financial markets.
These algorithms are often powered by AI and machine learning techniques to analyse large amounts of data, identify patterns, and make trading decisions. While algorithmic trading offers various benefits such as increased speed and efficiency, it also poses certain risks that need to be managed.
1. Data Integrity Risk: One of the critical aspects of AI in algorithmic trading is the quality and integrity of the data used to train and feed the algorithms. If the data is incomplete, inaccurate, or biased, it can lead to erroneous trading decisions. Risk management steps may include:
leading to model risk. Risk management steps may include:
Regularly validating and stresstesting the trading algorithms to assess their performance in different market scenarios.
Implementing strict model governance and version control to ensure that only validated and approved models are deployed for trading.
Monitoring the performance of the algorithms in real-time and promptly addressing any anomalies or deviations.
3. Market Impact Risk: Algorithmic trading strategies can generate a high volume of trades within short time frames, which can impact market liquidity and prices. Risk management steps may include:
Establishing robust data collection processes to ensure data accuracy and completeness.
Implementing data validation and cleansing techniques to identify and address data quality issues.
Regularly monitoring and updating data sources to account for changing market conditions.
2. Model Risk: The algorithms used in algorithmic trading are built on specific models that make predictions and decisions based on historical data. However, these models may not always accurately capture the complexities of the financial markets,
Conducting pre-trade and post-trade analyses to assess the potential market impact of the trading algorithms.
Implementing controls and circuit breakers to limit the order flow and prevent excessive market volatility.
Collaborating with regulators and exchanges to ensure compliance with market rules and regulations
4. Operational Risk: The use of AI in algorithmic trading introduces operational risks, such as system failures, cybersecurity threats, and connectivity issues. Risk management steps may include:
Enhanced Computational Power: Quantum computers have the potential to solve complex optimization problems exponentially faster than classical computers. This can enable more accurate and timely decision-making in capital markets.
Improved Portfolio Optimization: Quantum algorithms can analyse large datasets and optimize portfolio allocations based on multiple variables simultaneously. This can lead to improved risk-return trade-offs and better portfolio diversification strategies.
Implementing robust technology infrastructure and redundancy measures to minimize the risk of system failures.
Conducting regular cybersecurity audits and implementing robust security protocols to protect trading systems and data.
Developing comprehensive business continuity plans to ensure continuity of trading operations during unforeseen events.
Pros of Quantum Computing in Capital Markets:
Efficient Risk Assessment: Quantum computers can efficiently simulate complex financial scenarios, enabling faster and more accurate risk assessments. This can help market participants identify and mitigate potential risks, leading to improved risk management.
Cons of Quantum Computing in Capital Markets:
Current Limitations: Quantum computing is still in its early stages, and practical, largescale quantum computers are not yet widely available. The technology is evolving rapidly, but it may take time before its full potential is realized in capital markets.
Implementation Challenges: Integrating quantum computing into existing capital market infrastructure may pose implementation challenges. Quantum algorithms and models need to be developed, and significant investments in hardware and expertise are required.
While the potential of quantum computing in capital markets is exciting, there are several challenges and considerations to address:
a. Hardware Limitations: Quantum computers are still in their early stages, and building scalable, error-corrected quantum systems is a significant challenge. The current quantum hardware has limitations in terms of qubit coherence times, error rates, and the number of qubits available. Overcoming these limitations is crucial for practical implementations in complex financial applications.
b. Algorithm Development: Designing and optimizing quantum algorithms for specific capital market problems is an ongoing research area. Developing quantum algorithms that can outperform classical algorithms in a real-world financial context requires substantial effort and expertise.
vast amounts of data, and extracting meaningful insights from this data requires efficient data pre-processing, feature selection, and noise reduction techniques suitable for quantum algorithms.
d. Transition from Classical to Quantum: Integrating quantum computing into existing financial systems and processes will require careful planning and considerations. Transitioning from classical to quantum computing may involve challenges such as compatibility issues, data migration, security concerns, and training personnel with quantum expertise.
Conclusion:
c. Data Challenges: Quantum computing's potential benefits depend on the availability and quality of data. Capital markets generate
Quantum computing has the potential to revolutionize capital markets by providing enhanced computational power for solving complex optimization problems, portfolio optimization, and risk management. While the technology is still in its nascent stages, ongoing advancements suggest a promising future. As quantum computers continue to evolve and become more accessible, they can unlock new insights, improve decisionmaking processes, and enhance overall performance in capital markets. However, significant challenges remain, and further research and development are needed to fully harness the benefits of quantum computing in this domain
One of the current finance sector megatrends is artificial intelligence (A.I.). In a wide range of use cases, such as predictive data analytics, liquidity risk analysis, sentiment analysis, securities research, stock selection, voice-to-text natural language processing, intelligent matching of trades, market abuse, and financial crime surveillance, credit scoring, marketing, etc., many financial institutions have adopted and implemented A.I. into their daily operations. A.I. has the potential to have a beneficial worldwide influence on the financial markets sector. Financial institutions may profit from more incredible transaction execution speed and decreased investment service costs that result from using A.I., as well as other substantial efficiencies and advantages for financial institutions and investors. Regulators across the APAC region and beyond are considering their current and future regulation approaches as capital market players deploy A.I. for several use cases.
The use of A.I. in many different areas, including the capital markets, is governed by broad rules in many other nations. These laws frequently concentrate on the security, privacy, and fairness of A.I. systems.
1.Financial rules that apply to capital markets may indirectly influence the deployment of A.I. Securities laws, rules governing the financial markets, and guidelines for market behaviour are some of these regulations. Regulatory organizations that regulate capital markets and uphold fair practices include the U.K.'s Financial Conduct Authority (FCA) and the U.S. Securities and Exchange Commission (SEC).
2.High-frequency trading (HFT) and algorithmic trading both heavily use A.I. To address the possible hazards related to these practices, regulators have put in place certain restrictions.
These regulations frequently concentrate on topics like order routing, systemic risk, market manipulation, and the requirement for adequate risk management and system protections.
3.When applying A.I. in the capital markets, regulatory frameworks emphasize the necessity for solid risk management and
governance practices. Financial organizations must have precise rules and processes to evaluate, manage, and mitigate the risks related to A.I., including model risk management, model validation, and data quality.
4.To ensure fairness and prevent biased results, the use of A.I. in the capital markets has to be transparent and accountable. The ethical and responsible use of A.I., particularly the avoidance of discriminatory practices and the need for algorithmic decision-making to be explicable, is a growing concern for regulators.
5.The necessity for international collaboration and coordination in regulating A.I. is becoming increasingly evident, given the global character of finance markets. Standards and guidelines for the use of A.I. in financial markets are being developed by organizations like the Financial Stability Board (FSB) and the International Organisation of Securities Commissions (IOSCO).
they follow all applicable rules and regulations.
Applying artificial intelligence (A.I.) in the financial markets has several legal ramifications. Here are some crucial points to consider:
6.Intellectual property: A.I. systems may produce insightful insights, profitable trading methods, and efficient algorithms.
It's important to remember that the regulatory environment is changing, and new laws addressing A.I. in capital markets may be passed. Financial institutions should keep up with regulatory changes and ensure
As a result, problems with intellectual property rights might develop. Determining ownership and safeguarding AI-related ideas through patents, copyrights, or trade secrets is crucial for businesses.
7.Data security and privacy: The processing of a lot of private data, such as financial and personal data, is required when using A.I. in the capital markets. Data protection regulations, such as the General Data Protection Regulation (GDPR) in the European Union, must be followed. Companies must follow proper procedures for gathering, storing, and processing data as well as securing the required individual consent.
8.Liability and Accountability: Due to their complexity and autonomy, A.I. systems raise concerns about who is responsible for failures or errors. Product liability, carelessness, and contractual commitments may be considered while determining accountability. Establishing explicit contractual terms, outlining duties and obligations, and putting in place procedures to deal with possible liabilities is essential.
9.Insider Trading and Market Manipulation: Regulators are worried about the possible abuse of A.I. algorithms for insider trading and market manipulation.
businesses must work to reduce bias in data, algorithms, and decision-making models.
11.Observing current financial regulations, such as securities legislation, market behaviour guidelines, and reporting criteria, is necessary to apply A.I. in capital markets. Businesses should consider how A.I. systems affect regulatory requirements, including trade monitoring, risk management, and anti-money laundering (AML) controls.
12.Transparency and Explainability: There is an increasing desire for A.I. systems to be transparent and understandable. For example, regulators and stakeholders want transparent justifications for AI-based judgments in areas like credit scoring, investment advice, and risk assessments. Companies should work to create clear and understandable A.I. models and be ready to justify their decisions when asked.
Businesses must ensure that their A.I. systems abide by laws governing ethical business practices and guard against the misuse of sensitive data.
10.Discrimination and bias: A.I. systems can unintentionally reinforce prejudices or discriminate against particular people or groups. This raises questions about how anti-discrimination rules will be followed and how fair the decision-making process will be. To maintain compliance and fairness,
Future developments in capital market A.I. adoption are reshaping the sector and changing how financial organizations function. These significant tendencies should +be noted:
1.Enhanced Trading Techniques: A.I. algorithms will keep developing and adopting more complex methods like deep learning and reinforcement learning. These developments may result in more precise forecasts, improved trading methods, and enhanced risk control.
To help stakeholders comprehend the justification for AI-generated decisions, XAI seeks to develop transparent and interpretable A.I. models.
4.Reinforcement Learning in Portfolio Management: Reinforcement learning methods are used in portfolio management, allowing artificial intelligence (A.I.) systems to discover the best trading strategies through making mistakes. This strategy may improve portfolio optimization and enable adaptation to shifting market conditions.
2.A.I. systems can interpret and analyse human language thanks to natural language processing (NLP) technology. Better news aggregation, sentiment analysis, and qualitative data interpretation are made possible by it, which helps determine the best action for investments and keeps tabs on market trends.
3.A.I. that can be explained is known as explainable A.I. (XAI), and its relevance is growing as A.I. systems get more complicated.
5.Predictive analytics and alternative data: Financial institutions rapidly combine IoT data, satellite images, and social media feeds into their A.I. algorithms. They can produce alpha, find novel insights, and spot market trends using predictive analytics.
6.Artificial intelligence (A.I.) is being used to fuel regulatory technology (RegTech) systems that will automate regulatory compliance procedures, including AML, KYC, and transaction monitoring. These technologies can simplify regulatory reporting requirements, lower expenses, and improve accuracy.
7.While still in its infancy, quantum computing has enormous potential for accelerating computations and addressing challenging optimization challenges. Quantum computing can transform algorithmic trading, risk management, and portfolio optimization in the financial sector.
8.A.I. solutions collaborating with human traders' knowledge and A.I. algorithms are becoming more popular. To make better investment decisions and control risks, these systems combine the powers of A.I. with human judgment.
decision-making. This combination can improve operational efficiency in the capital markets by streamlining transactions.
Conclusion:
The financial services industry will see both advantages and hazards from A.I. The regulation of A.I. should continue to be guided by market safety, investor protection, and market integrity to ensure continuity. In addition, financial access should be a regulatory goal so that A.I. can be utilized to help financial intermediaries and people who were previously shut out of economic prospects.
9.Ethical A.I. and Responsible Innovation: As the use of A.I. spreads, ethical issues and responsible innovation are becoming increasingly important. To preserve regulatory compliance and public confidence, financial institutions are expected to assure fairness, minimize prejudice, and handle the ethical implications of A.I. systems.
10.Integration of A.I. and Blockchain: The combination of A.I. and blockchain technology opens up possibilities for the execution of intelligent contracts, safe and open data exchange, and decentralized
The availability of financing will aid in adopting A.I. to the general population. To achieve this goal, A.I. may be used to optimize capital on peer-to-peer platforms, provide consumers with more affordable access to knowledge through robo-advisers, and use RegTech services to improve KYC/CDD processes, hence lowering costs. To strengthen ex-ante and increase ex post the protection of people who use roboadvisers, as well as to address how individual rights, such as privacy rights and data rights, can be protected to enable the conduct of more effective KYC processes, more specific rules need to be develope