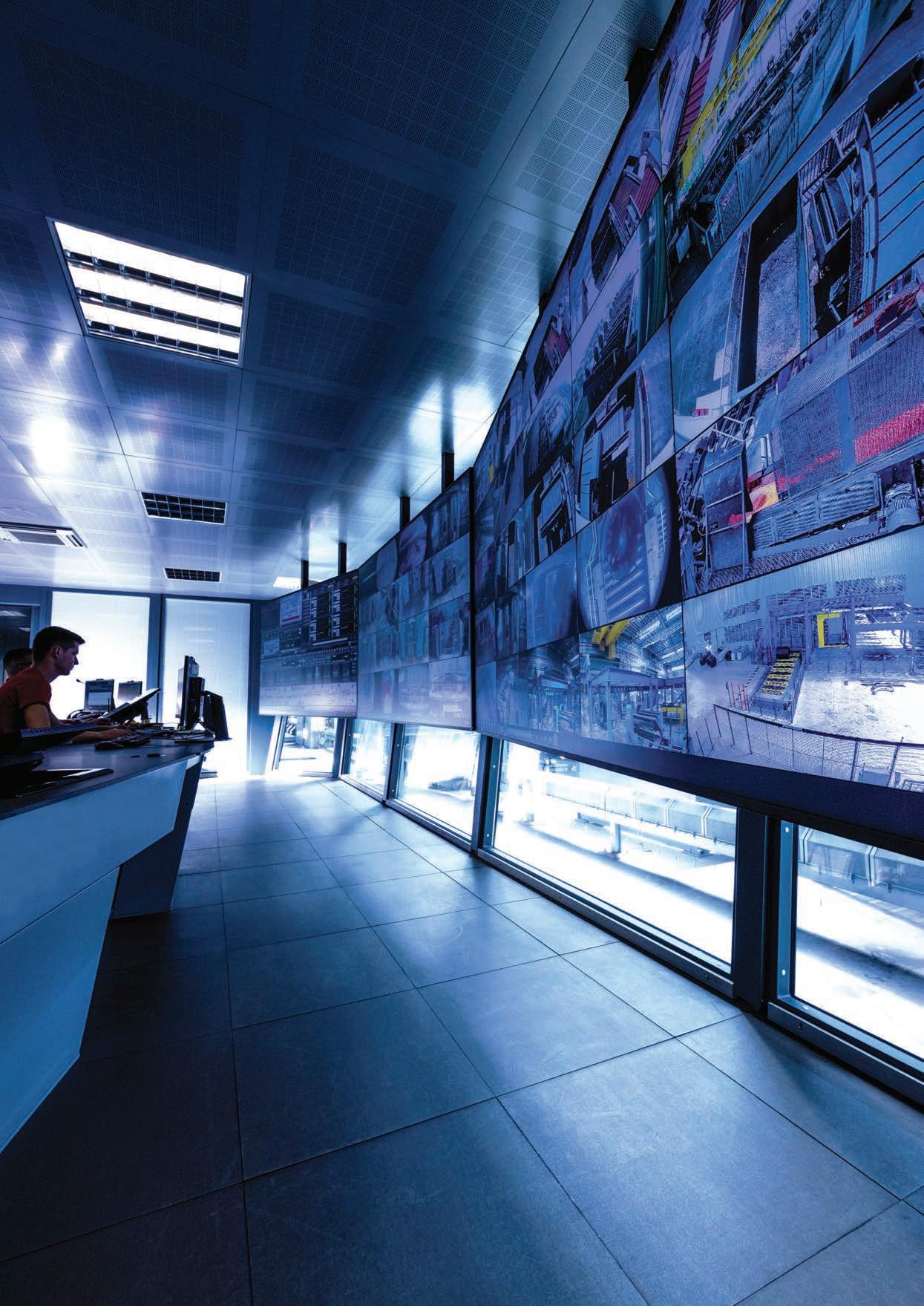
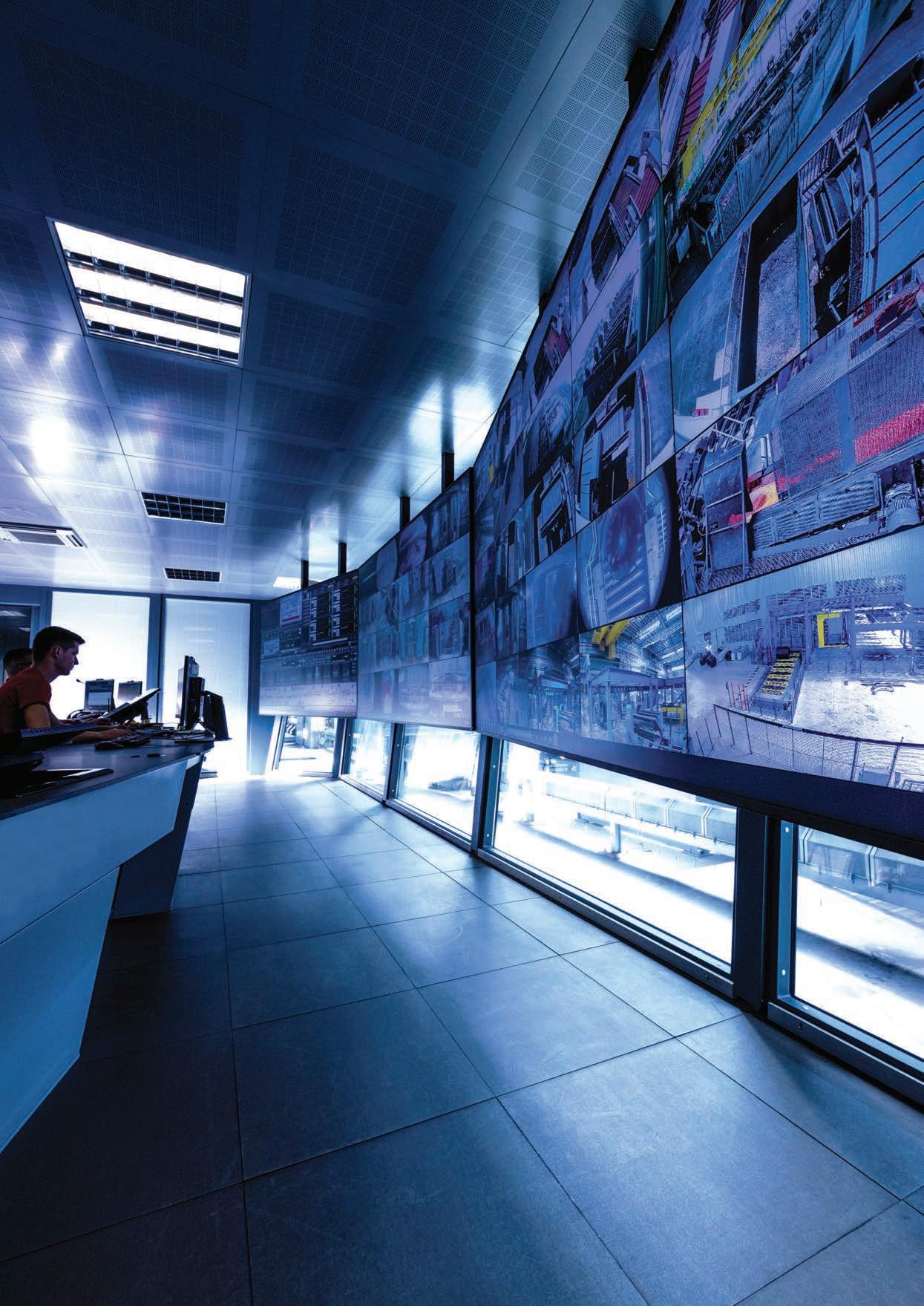
PSImetals
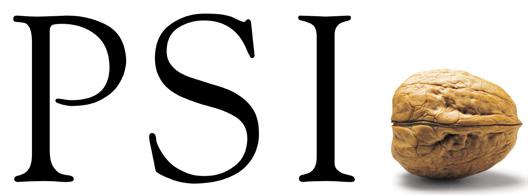


empowers
sustainable operations for generations to come
Cover: Danieli
Overview of the latest generation Danieli Automation DIGI&MET highly automated control pulpit, operative at ABS QWR plant (Italy)
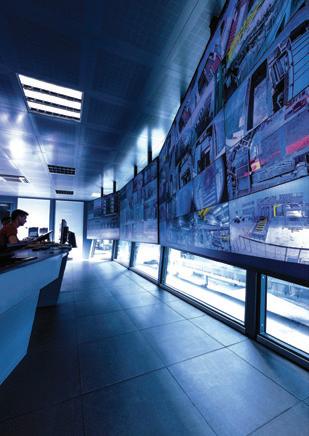
Editorial Editor / Programme Director
Matthew Moggridge +44 1737 855151 matthewmoggridge@quartzltd.com
Editorial Assistant Catherine Hill +44 1737 855021
Production Editor Annie Baker
Advertisement Production Martin Lawrence Sales
International Sales Manager Paul Rossage +44 1737 855116 paulrossage@quartzltd.com
Sales Director Ken Clark +44 1737 855117 kenclark@quartzltd.com
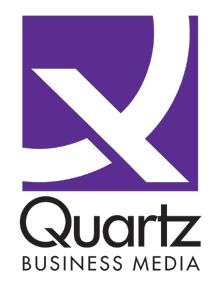
Corporate Managing Director
Tony Crinion
Published by: Quartz Business Media Ltd Quart House, 20 Clarendon Road Redhill, Surry RH1 1QX, UK +44 1737 855000 www.steeltimesint.com
© Quartz Business Media, 2022
Contents
2
Introduction by Matthew Moggridge, programme director. 4 Summit programme. 6 Speakers’ biographies. 11 Sponsors. 12 Data science: Data science for a steel factory. 16 Artificial intelligence: Transforming steel plants with AI. 20 Machine learning: Building a smart factory. 30 Tracking systems: Neural networks for visual tracking.
Welcome!
The two biggest and most dynamic areas of development within the modern global steel industry today are decarbonization and digital manufacturing (Industry 4.0). These two subjects are top of the publicity tree if you’re looking outside of the news agenda on industry in general. Quite obviously, there are other issues of great importance to steelmakers, notably the urgency surrounding the price of energy that has risen its ugly head as a direct result of the equally ugly war in Ukraine, brought to us all by Russia’s President Vladimir Putin.
Steel Times International is currently at the sharp end of both decarbonization AND digital manufacturing. With the former we have just announced the first LIVE Sustainable Steel Strategies Summit (to be held in Abu Dhabi in March 2023). With the latter we have the already highly successful Future Steel Forum conference, launched in June 2017, which next year will run in September. Between now and then we have our online Artificial Intelligence and Steelmaking event. This digital supplement provides you with all the information you need: the programme, the speakers, the sponsors and some interesting articles from Arcadia Steel, Tenova, Canvass AI, and Polytec USA/ BM Group Polytec Italy.
I hope you enjoy the event and look forward to seeing you in Abu Dhabi for the Sustainable Steel Strategies Summit live conference, and at a venue yet to be decided for the September Future Steel Forum, both in 2023 – the event’s SEVENTH highly successful year!
Moggridge, Programme Director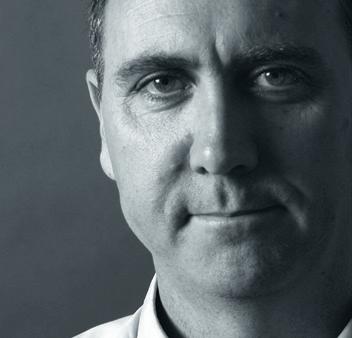
Use AI to succeed where others have failed
A lot of industrial companies talk about artificial intelligence and digital transformation. But only 16% of their efforts have actually improved performance or laid a foundation for sustained progress. AI needs the correct infrastructure to succeed. Not only do we make that possible—we make it practical. Our purpose-built, AI-driven monitoring systems can be installed and generating alerts within a few weeks. We deploy and manage all the hardware, software, and AI. All you need to do is respond to the alerts. Diagnostics are 99.9% accurate, and the solution delivers value at any scale—insights at the machine, plant, and portfolio levels. With improved reliability, reduced downtime, optimized production, and increased yield, typical customer pay-back time is just 3-4 months.
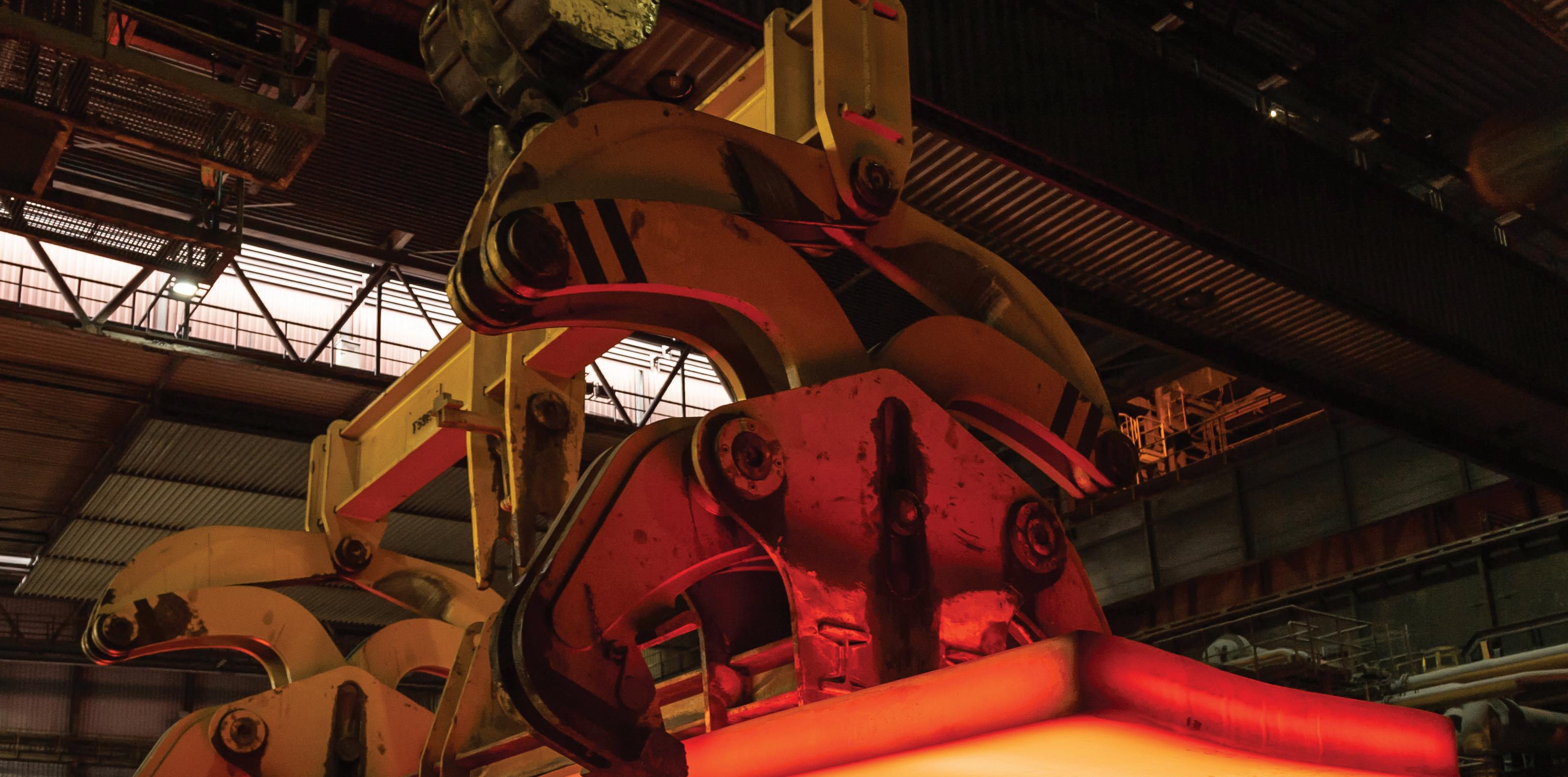
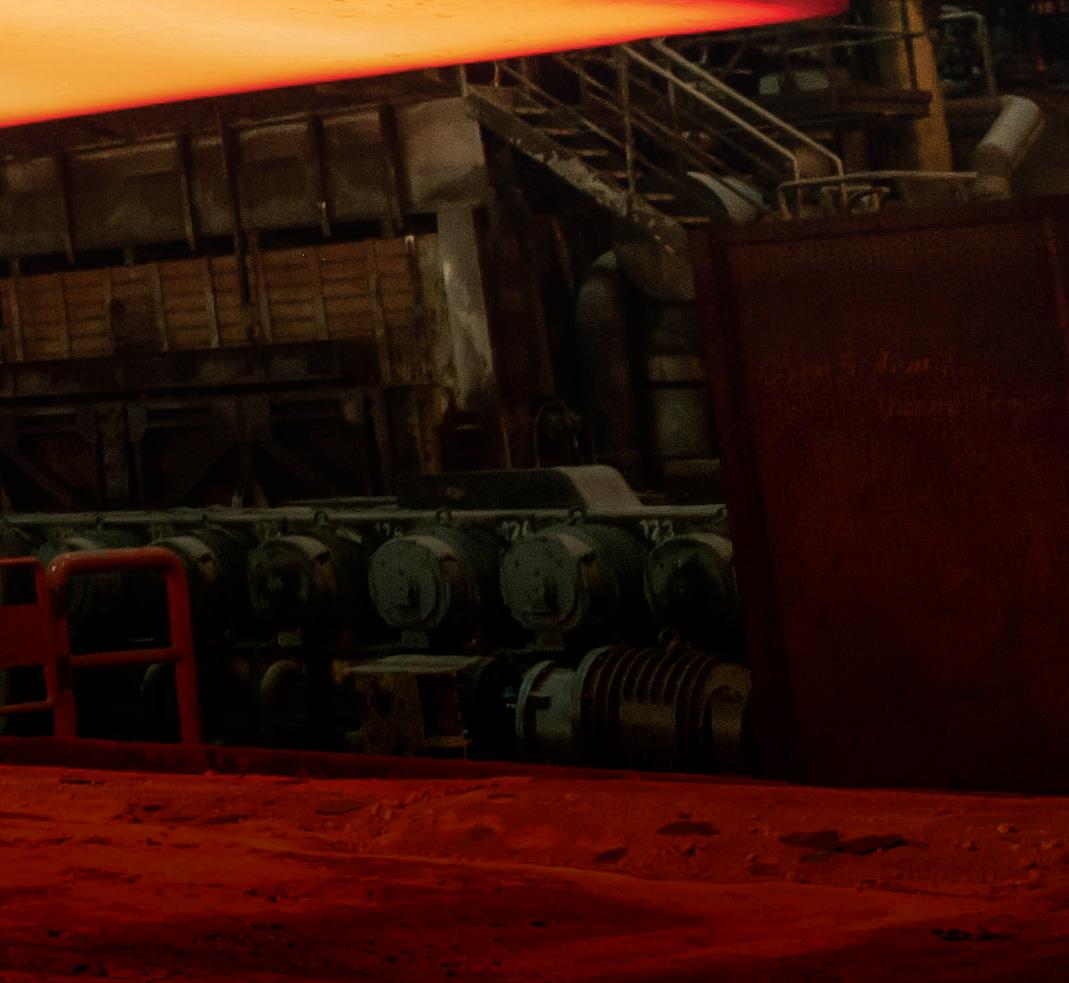

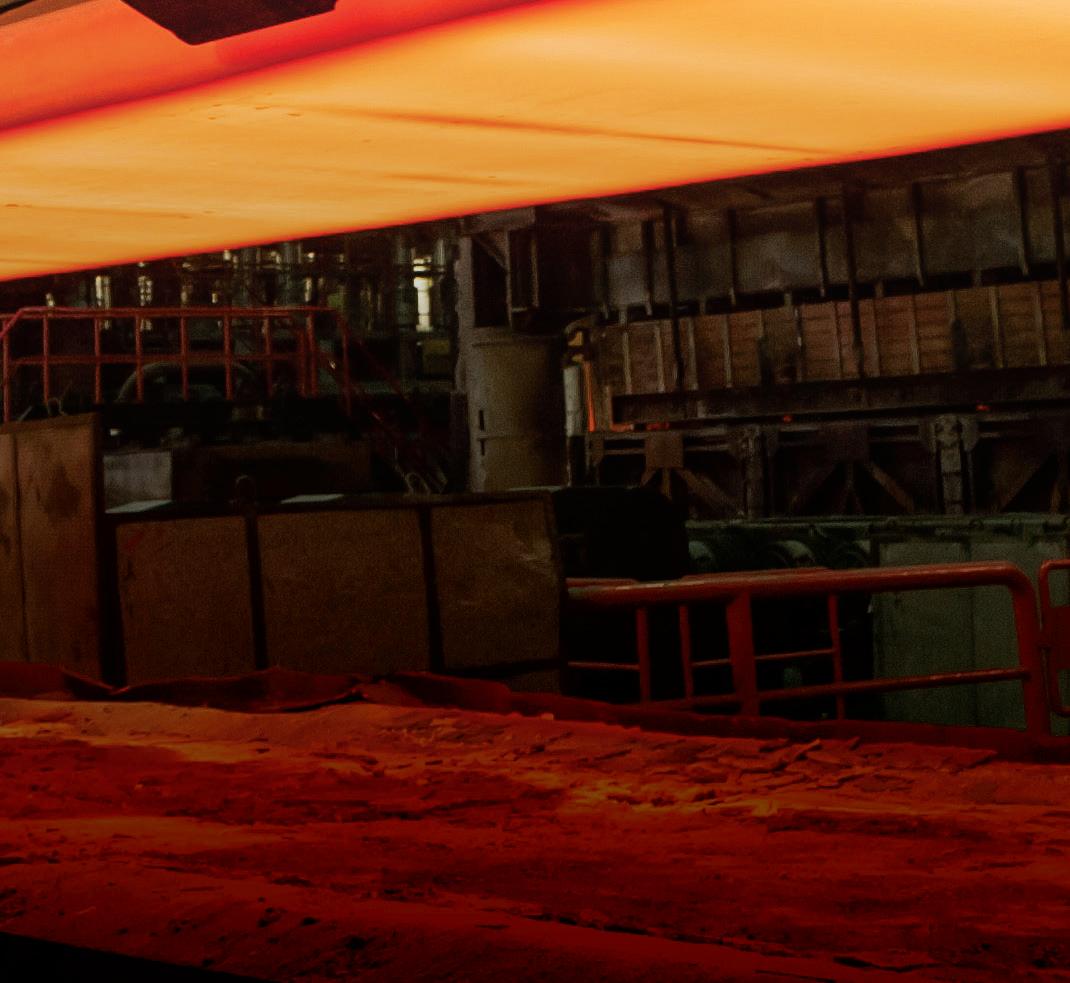
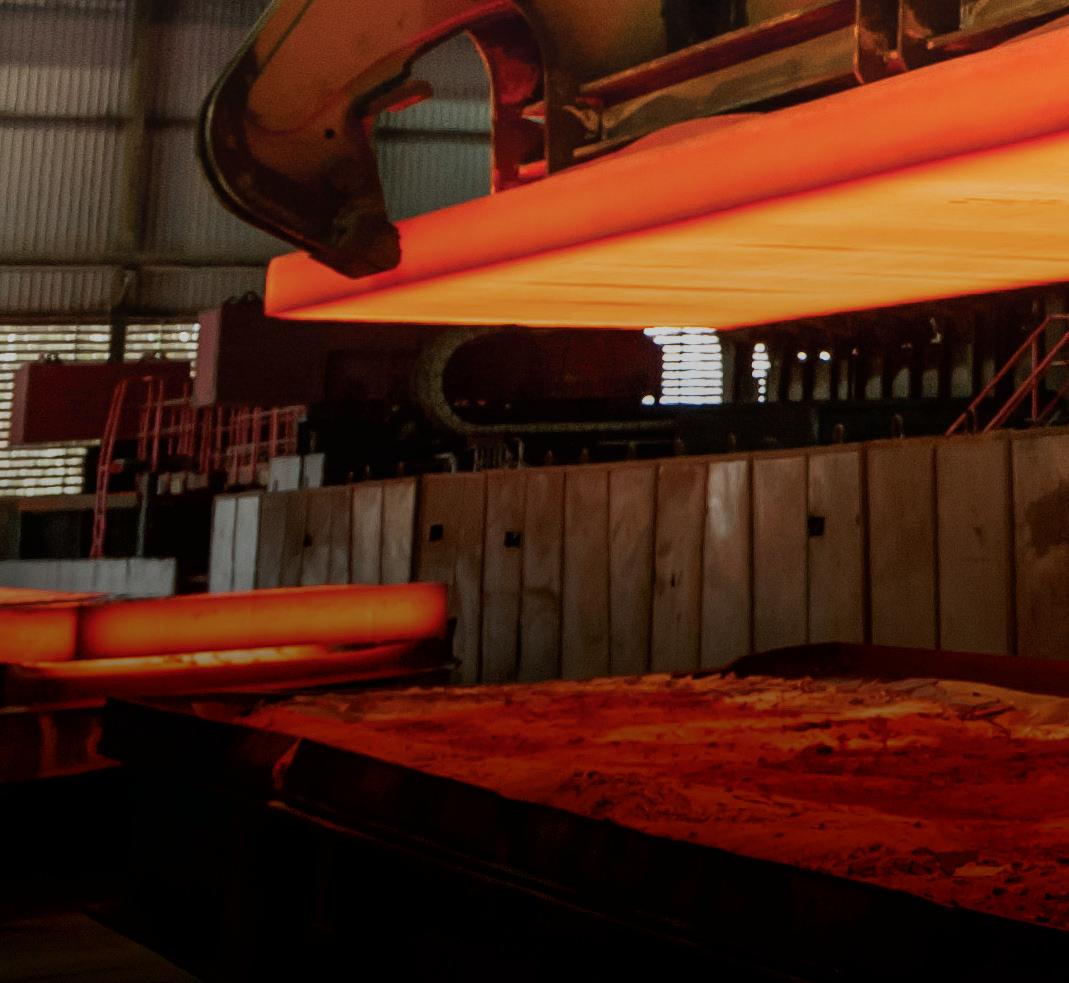
Turn your digital transformation into a success story.
bently.com
Copyright 2022 Baker Hughes Company. All rights reserved.CONFERENCE PROGRAMME
10.00: Introduction by Matthew Moggridge, Editor Steel Times International
10.10: Data Science and Big Data Tools for a Steel Factory: models and a technology stack by Boris Voskresenskii, Arcadia Steel.
10.30: Worker-centric AI: The Industrial Revolution is Here, by Sandeep Pandya, chief executive officer, Everguard.ai
11.00: Machine Learning Applications in a Steelmaking Factory by Giovanni Bavestrelli, director of data science, Tenova SpA. 11.30: Networking Break
12.00: Industrial AI: Beyond the Stars or Down to Earth by Luc van Nerom, innovation manager, PSI Metals, and managing director, PSI Metals Belgium; and Robert Jäger, senior supply chain expert and product manager, PSI metals.
12.30: How India’s leading iron ore processor and pig iron producer controls emissions through an AI-powered process control digital twin, by Ramesh Kumar, head of technical solutions, Eugenie.ai
13.00: Lunch Break

CONFERENCE PROGRAMME
14.00: Predicting Cobble in Steel Mills using Artificial Intelligence, by Satish Agarwal, principal, manufacturing practices, affine.ai.
14.30: The promise of AI versus the reality of AI today, by Christopher Zinn, enterprise solution architect, Bently Nevada (part of Baker Hughes).
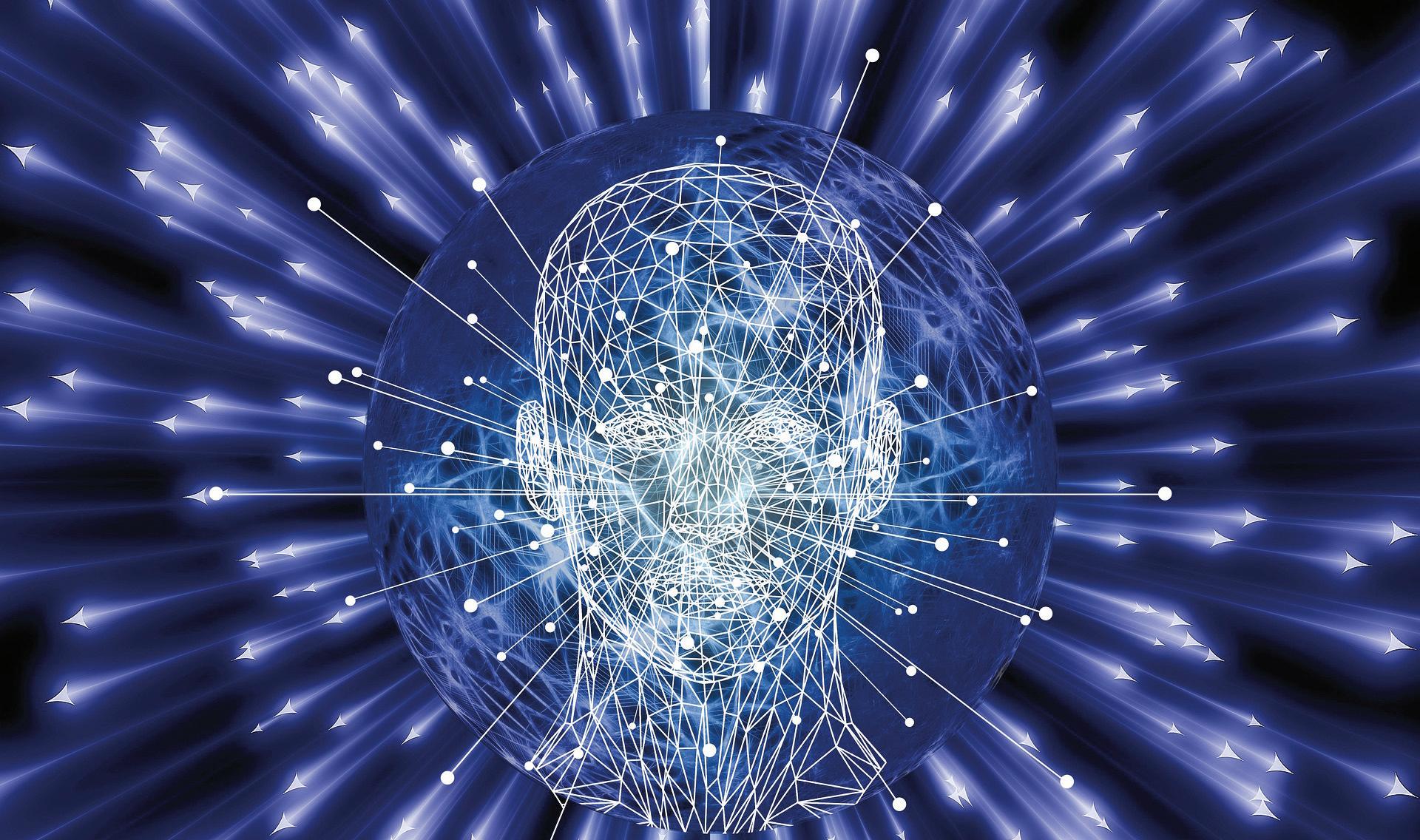

15.00: Neural Network Technologies for Visual Tracking by Gianluca Maccani, chief technology officer and president, Polytec USA Corporation.
15.30: Networking Break
16.00: Leveraging Industrial AI to Increase Throughput in Steel Manufacturing by Forogh Askari, industry lead, Canvass AI.
16.30: Conference Closes.
SATISH AGARWAL
PRINCIPAL, MANUFACTURING PRACTICES, AFFINE.AI
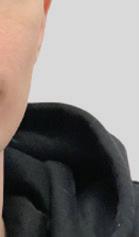
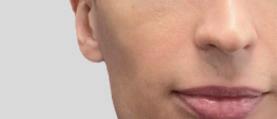
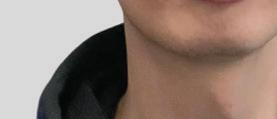
Satish Agarwal has over a decade of experience within the field of digital transformation, with experience using artificial intelligence (AI) and machine learning (ML) for analytical solutions to business problems. He is currently working on business development in the domain of the AI and ML world and working on solving real challenges in the industry.
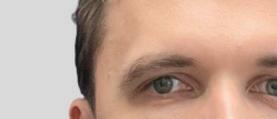
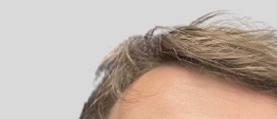
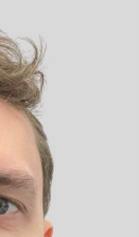

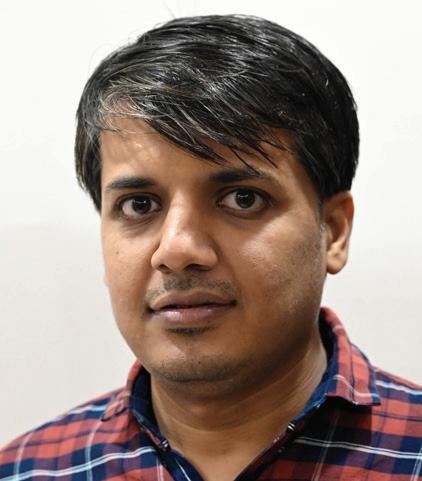
BORIS VOSKRESENSKII CO-FOUNDER, ARCADIA STEEL
Boris Voskresenskii is a founder of Arcadia Steel and ex-CDO of a leading Russian steel company. He and a part of his team left Russia and quit their jobs due to their position on the Russian-Ukraine war. Boris joined the Russian steel company in 2017 as head of data science and was appointed CDO in 2020. He was awarded the status of Kaggle competition master in 2018. Boris has also worked in data science and was head of a risk management department in a factoring company.
ZINN
ENTERPRISE SOLUTION ARCHITECT, BENTLY NEVADA

Christopher Zinn has a background in electrical design and IT/network infrastructure, as well as over 11 years of experience with Bently Nevada, a Baker Hughes business. He joined the company as a field services engineer and later moved to the machinery diagnostics team. He then brought his in-house expertise to Bently Nevada’s Supporting Services team where he worked with one of the company’s key offshore customers before finally taking on the enterprise solution architect (ESA) role. As an ESA he focused on supporting enterprise software solutions and analytics using first principle physics models and artificial intelligence (AI).
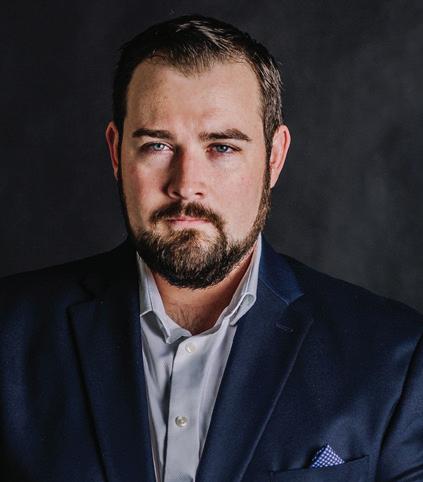
GIANLUCA MACCANI
CTO AND PRESIDENT, POLYTEC USA CORPORATION
Gianluca Maccani is chief technology officer and president of Polytec and has over 15 years of experience with the company. He is passionate about advanced automation, large drives, process digitalization, robotics, and production processes for the steel industry, and has a deep understanding of the steelmaking process. He is an experienced technologist with a desire to discover creative ways to blend new and legacy technologies to minimize operators’ exposure to dangerous tasks and simultaneously improve quality and productivity in heavy manufacturing processes (including steel, pulp and paper).
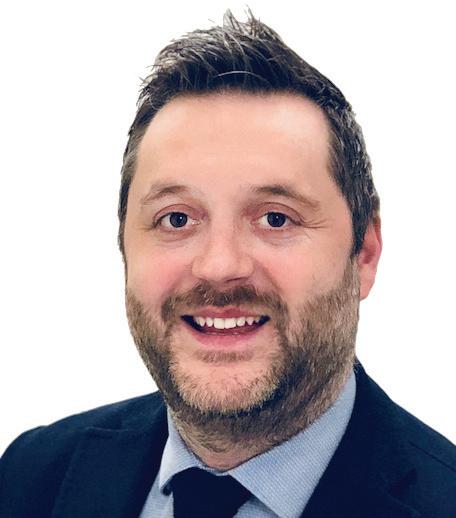
SANDEEP PANDYA
CEO, EVERGUARD.AI
Sandeep Pandya is CEO of Everguard.ai, a company leading the way in the incorporation of wearables with computer vision, machine learning, and artificial intelligence (AI) to make the world’s industrial environments safer and more sustainable. Formed in 2019, Everguard.ai stemmed from Pandya’s belief that the world is experiencing a technological renaissance, and that the nexus of AI, digital connectivity, and information sharing will fundamentally reshape and improve the way people and their communities sustain each other. Pandya is a technology veteran whose experience spans product introductions and innovations in semiconductors, connected devices, wireless infrastructure, cloud services, and novel AI/CV applications. His leadership has helped organizations of all sizes establish high-growth markets globally. Sandeep recently wrote and published The ESG Revolution: How Worker-Centric AI is Transforming Industrial Sustainability which aims to support leaders in reshaping the way they think about ESG as well as providing the tools needed to transform their companies.

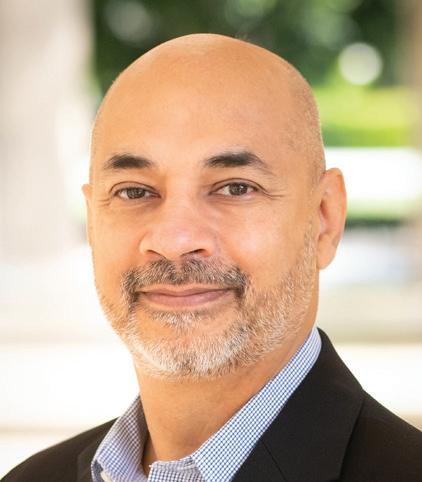
Luc Van Nerom studied mathematics and computer science and started as a researcher at the AI Lab of the Vrije Universiteit Brussel. In 1986 he created a spin-off company ‘Artificial Intelligence Systems’, bringing AI and optimization technology to the metals industry. After a merger, the products of this company have been embedded in the production management software of PSI Metals. Today, Luc is focusing on product innovation management and industrial intelligence at PSI and PSI Metals.
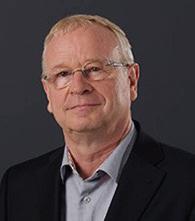
ROBERT JÄGER
SENIOR SUPPLY CHAIN EXPERT AND PRODUCT MANAGER, PSI METALS
After several years implementing PSImetals’ planning solutions at multiple plants, Robert Jäger spent10 years in a consultancy and technical sales support role, helping in the requirements analysis and design of planning solution architectures tailored to specific metals supply chains. As product manager and building upon his supply chain expertise, Robert is now driving PSImetals’ planning product roadmap with a vision centred on smartness, adaptiveness, collaboration and sustainability.
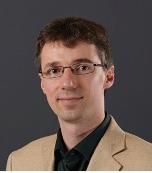
RAMESH KUMAR

HEAD OF TECHNICAL SOLUTIONS, EUGENIE.AI
Ramesh is an AI-led digital transformation leader for Industry 4.0 with a large focus on the manufacturing sector. He holds more than16 years of experience across metals and mining, aviation, heavy engineering and hi-tech industries. Prior to his current position, he held managerial roles within multiple companies focused on digitalisation and manufacturing.
Ramesh holds an MTech from the Indian Institute of Technology, Kharagpur, and a BTech from the National Institute of Durgapur.
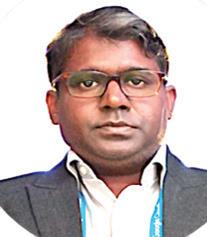
GIOVANNI BAVESTRELLI
DIRECTOR OF DATA SCIENCE, TENOVA SPA
SPEAKERS
Giovanni Bavestrelli set his hands on a computer keyboard for the first time while at high school in South Africa in 1981. It was love at first touch. He went on to study software engineering at the Politecnico di Milano and worked as a software engineer for various companies in different sectors. Since 2000 he has worked for two companies; first Unisys Italia, as a manager in the team that developed the Hermes Editorial System for major newspapers and magazines throughout the world, and since 2004 for Tenova, a worldwide leader in the development of solutions for the metals and mining industries. In Tenova, he initially led the software development team for Pomini roll grinding machines. In 2017 he helped create and then lead the Tenova digital team, tasked with assisting various business units in introducing new technologies related to Industry 4.0. He now leads Tenova’s data science team and is particularly interested in machine learning. He still loves coding.
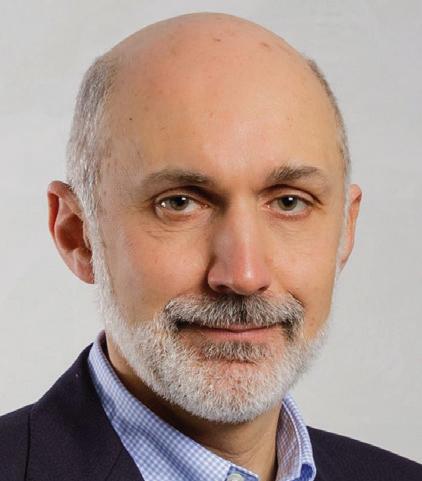
FOROGH ASKARI
INDUSTRY LEAD, CANVASS AI

Forogh is an industry lead at Canvass AI, a software provider that makes artificial intelligence accessible to industrial engineers. In her role, Forogh enables and educates users on the benefits of using AI in industrial operations, thus bridging the divide between industrial process engineers, data science and machine learning. Prior to joining Canvass, she held similar roles at Emerson Impact Partners across Canada for over 10 years, where she worked with manufacturing and oil and gas companies to develop their digital transformation initiatives through process automation and data analytics. Forogh has a Bachelor of Applied Science degree in chemical engineering from the University of Waterloo.
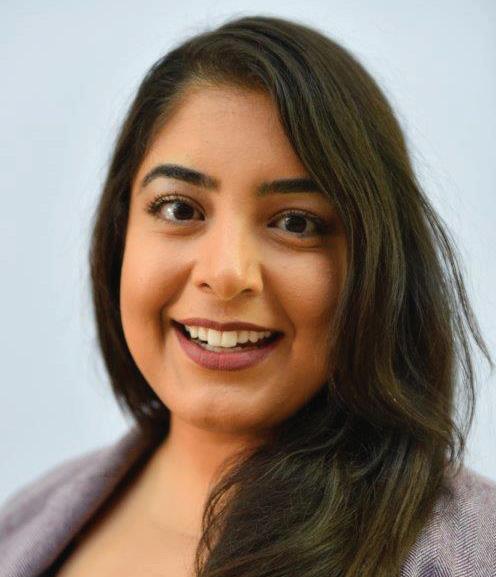
MEET THE SPONSORS
BENTLY NEVADA, A BAKER HUGHES BUSINESS
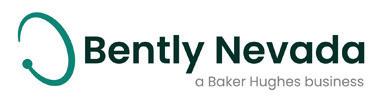
Bently Nevada recognizes that the performance of business assets fundamentally governs the performance of any organization, which is why it has dedicated the last 60+ years to safeguarding the health of the machines that matter most. Bently Nevada's hardware and software offerings, enabled by analytic insights and managed services, help organizations improve asset performance while effectively managing risk and reducing costs. Today, by leveraging domain expertise from Bently Nevada, ARMS Reliability, and Augury, the company delivers industry-leading APM technology, content, and services to help customers achieve the highest level of reliability possible – from the edge to the enterprise. Bently Nevada is a critical business in the broader Baker Hughes Industrial Asset Management (IAM) portfolio. When combined with the recent acquisition of ARMS Reliability, a partnership with Augury, and the BakerHughesC3.ai alliance, Baker Hughes continues to progress its IAM portfolio, harnessing the power of engineering, data, and science to redefine what's possible.

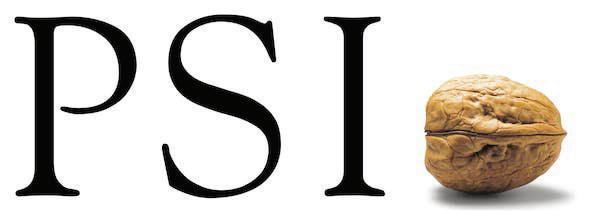
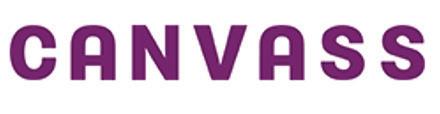
CANVASS AI
PSI METALS
Canvass AI is a leading industrial AI software provider that puts the power of artificial intelligence in the hands of industrial workforces to solve day-to-day operational problems. Some of the world’s largest companies use Canvass AI’s patented industrial AI Platform to reduce carbon emissions and waste, improve yields, and optimize facility operations. Backed by Alphabet and Yamaha Motor Ventures, the company is recognized by CB Insights as one of the world’s top 50 technology companies that is advancing manufacturing.
PSI Metals is a leading provider of innovative production management software for the metals industry, combining supply chain management, advance planning scheduling, and manufacturing execution systems within one Service Platform (SP). PSI’s end-to-end solutions enable steel and aluminium producers to stay competitive by delivering tailored and configurable solutions since 1969. To support all processes such as planning, production, quality, logistics and energy, the PSImetals Service Platform allows sustainable and highly integrated solutions. PSI considers the latest developments in the field of digitalization and user empowerment and supports its clients on their decarbonization missions.
There are many examples of effective data science solutions in the steel industry. Yet, widespread adaptation of this technology is still a far-off concept. For this to change, it is essential to share modern tools from data science and big data approaches in the professional community.

Data science for a steel factory Data science for a steel factory
factory factory
Let’s start with the core of any digital product – data and how to work with it. As I, with Kseniia Kingsep, wrote in a previous article: for any product, you need data, a product owner, and a discernible effect for your company. But where can you find data in an accessible way to start a project quickly, and how can you use the data in your real-time application?
There are two main ways of starting your data warehouse using information from your manufacturing units:
1. Clean data approach
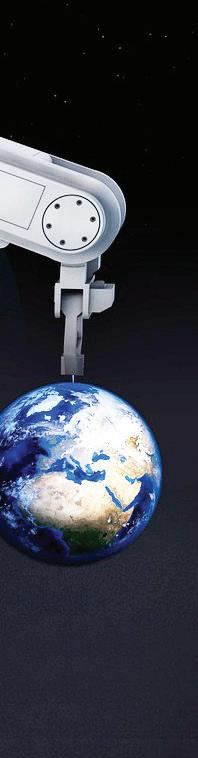
2. Raw data approach
With the clean data approach, you should create a data governance process, clean your data first, and after that, you can store your data in any data warehouse. Because the data is structured and you don’t have too much of it, you can use any relational databases (Postgres, Oracle, MS SQL, for example).
But it is a very slow and costly approach. For a much quicker start, you can use the raw data approach. Store all the data that you have and then extract it with big data technology. For example, you can use Apache Hadoop2 and its hdfs component as a file system. In Hadoop, you can sync all your data across different sources, such as your sensors, MES, and cameras. Then you can use Hive as an abstraction layer over the data to create tables and process this data with SQL. Also, you can process it with Apache Spark when you need something more than SQL. This approach allows you to store easily and process petabytes of data without huge software expenses. As disk space is relatively cheap, it is reasonable to store all data first and process it after you understand what you want to achieve with this data. You certainly don’t want a case to happen when you have a potential project with a $100m net present value and don’t have all the data for it because you have not yet ended your data governance process.
How can you receive your data from sensors in a reliable way? And how can you integrate your real-time data science solutions with your units? Here you have Apache Kafka3 as a reliable distributed event streaming platform for this task. You can develop your own Java or Python software to communicate between PLCs and Kafka, so
all information from your PLCs goes straight there. That software can also listen to Kafka, and when control parameters from your data science solutions are there, push them into your units through their PLCs. Kafka doesn’t need many CPUs for this scenario; the key hardware part here is fast SSDs.
You can also sync all the data from Kafka with your Hadoop cluster utilizing tools like Apache NiFi4 The main benefit of Apache Kafka is that you can have many software solutions with Kafka without conflicts; these solutions can take data from Kafka simultaneously, process it at its own pace, and send the results of this process to their models’ topics.
So, you have many small software solutions that directly control your units. How can you orchestrate and support them? We have two essential technologies – Docker Containers5 and Kubernetes6 . With Docker Containers, you can create isolated spaces for your software solutions. If you update versions of your software libraries, for example, it won’t affect your solutions in Docker Containers. And these dockers can run consistently on any infrastructure that you have. Kubernetes (also known as K8s) is a container orchestration engine for automating containerized applications’ deployment, scaling, and management. With this system, you can orchestrate billions of software solutions with reasonable human support.
Let’s continue with the software solutions. Using a data science approach, how can we create something reliable to control units? For this, I recommend Python as a programming language. Of course, you can use R language for research, which is pretty popular, especially in the scientific community, but for real-time solutions, Python is preferable.
Let’s talk a little bit about the workplace of your data scientist. Usually, you can see that data scientists do something in Jupyter Notebook7 and show you beautiful plots. Still, when you start to create real-time software, you should go a little deeper into the best practice of software development. First, you should use Git for all your code, including notebooks, and store it on GitHub/ GitLab or a similar developer platform.
Second, you should use a code editor that is comfortable for your scientists, such as VS Code or PyCharm. And, of course, you should
treat your data scientists as any other software developer with a code review process and other essential procedures.
When you have a big data science team, you should standardize your Python code which wraps your models and communicates with other modules or systems such as Kafka. For this, I recommend writing your wrapper, but also you can use other open-source wrappers, such as typed-blocks8
The next important thing in developing data science tools is reproducibility. It is essential to do reproducible research because you can’t continuously build your applications without it. For example, you want to check why your model starts to work strangely on the actual data after a half year in production, or you want to check how your different models act in this environment, or you want to show your new colleagues how you achieve your result. For all these tasks, you should use the reproductive research approach, which is a standard in data science.
For this purpose, I can recommend such tools as:
1. MLflow9. With MLflow, you can
track your experiments, manage your models, and store ML code in a reproducible form.
2. DVC10 (Data Version Control). With this version control system, you can store all the datasets of your previous experiments. It is crucial for reproducibility because your datasets can be changed over time, and you may have considerable trouble reproducing your experiments without keeping the same version of the dataset.
Let’s talk briefly about models and general hints about when you should use them.
1. If you have a small dataset in tabular form – use linear or logistic regressions. Linear is for regression tasks, and logistic is for classification tasks.
2. If you have a large dataset in tabular form – you can use gradient boosting models such as xgboost, lightgbm or catboost, or random forest models. In most cases, you should not use neural networks for these tasks.
3. You need to create a recommendation system on top of a predictive model in many cases. In these cases, you should not forget that correlation
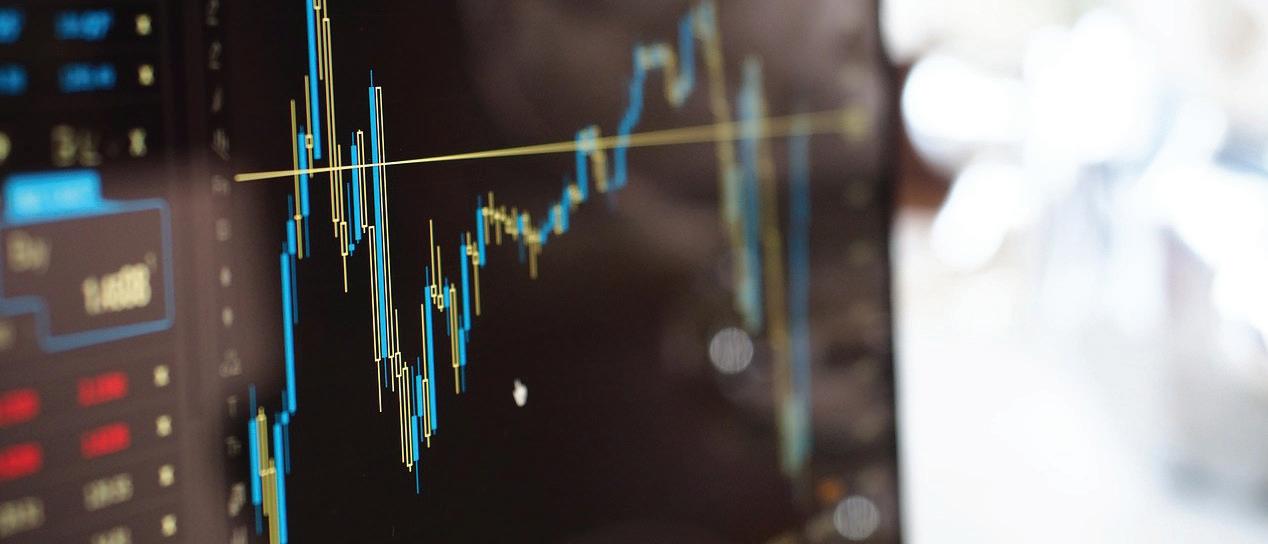
does not mean causation. I have an article with a case from a reversal steel mill on that topic11
4. If you have text or image data, you should use various neural networks and be ready to have GPU servers for these tasks. There are two main neural networks –Tensorflow and PyTorch.
5. If you don’t have enough data –use a Generative Adversarial Network for its generation.
6. If you want to improve your unit’s sequence of control operations, you can use reinforcement learning models. The most challenging part is coding a digital twin for your unit. Here, you can find an example of using reinforcement learning agents for a pickling line12
Simplicity rules
At the end of the day, the most crucial part of all your solutions is to be as simple as possible. If you develop a good heuristic for your task and it is provable by data, then use it. In many cases, you will see that a good heuristic will have a similar result as a highly sophisticated neural network. �
References 2. https://hadoop.apache.org/ 3. https://kafka.apache.org/ 4. https://nifi.apache.org/ 5. https://www.docker.com/resources/what-container/ 6. https://kubernetes.io/docs/home/ 7. https://jupyter.org/ 8. https://github.com/severstal-digital/typed-blocks 9. https://mlflow.org/#community 10. https://dvc.org/ 11. https://towardsdatascience.com/causal-inference-for-a-steel-mill-1e4dff991501 12. https://arxiv.org/abs/2008.06933v2
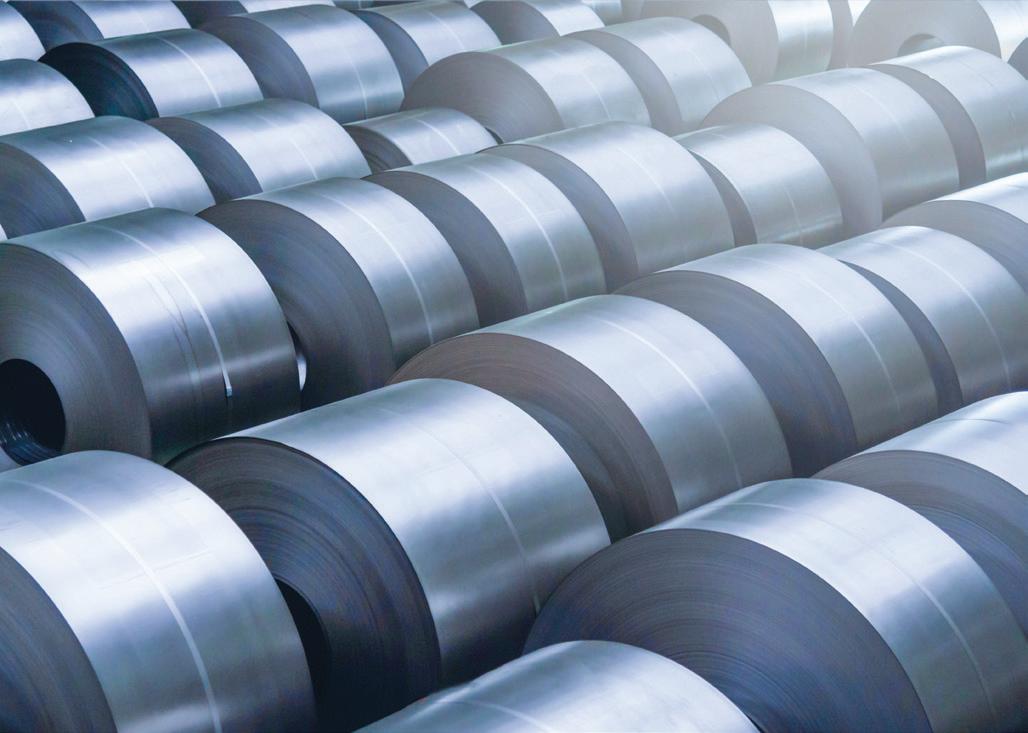

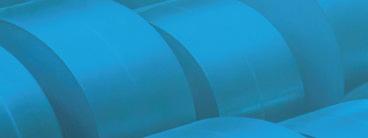
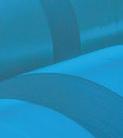
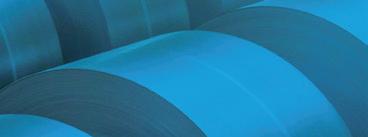
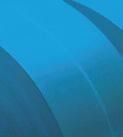
Transforming steel plants with AI Transforming steel plants with AI
Artificial intelligence (AI) is increasingly being adopted across different industries, as organizations look to harness its potential to drive efficiencies, improve decision-making and remain competitive. The steel industry is no different – in fact, it is one of the most rapid early adopters of AI.
Steel making is complex, relatively well instrumented and relies on a large skilled workforce to efficiently operate capital intensive assets. All these characteristics help steel production plants drive transformational value through Industrial AI for maximizing production, improving sustainability, and reducing costs.
In this article, we’ll explore important ways in which Industrial AI is being used in the steel industry and the potential benefits that it can bring to the production teams.
Unlocking the full potential
The global steel industry is under pressure. Increasing costs, competition from low-cost countries, overcapacity and geopolitical concerns have led to consolidation and the need for more efficient production.
Forward-thinking companies are already using Industrial AI to gain a competitive edge, and adoption is expected to grow rapidly within the steel industry in the near future. Recent advancements in specialized Industrial AI are opening new opportunities for the industry to increase efficiency and optimize operations. From identifying and classifying defects, to predictive maintenance, sustainability and quality control, purposebuilt Industrial AI is finding new applications in steel companies to solve problems in more efficient and cost-effective ways.
In a recent study by Boston Consulting
*CEO and founder, Canvass AIGroup that included steel companies in two key global markets, it was found that by connecting assets through data and by generating insights to change processing instructions and raw material input mixes, companies could achieve significant results. They were able to:
� Reduce raw material input costs by more than 5%
� Improve throughput at bottlenecks by more than 6%
� Increase end-to-end product yields by more than 15%
Pre-packaged Industrial AI solutions are designed to solve industry-specific problems. Pre-packaged solutions help organizations start with smaller but important problems, derive immediate value, build trust in AI with the workforce and expand into additional
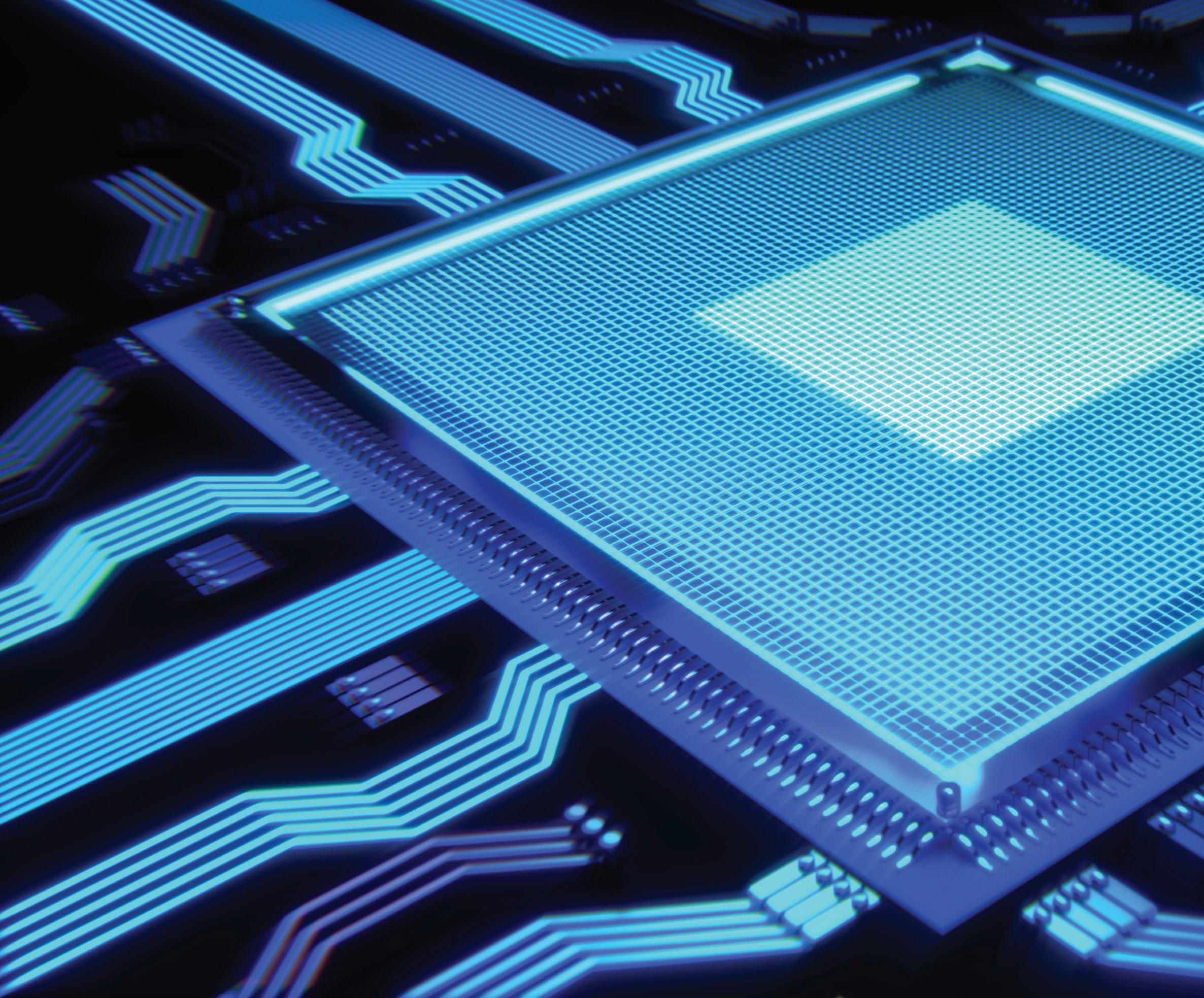
ARTIFICIAL INTELLIGENCE
areas of value. This ‘start small and expand’ strategy helps organizations adopt AI at the pace they are comfortable with, while deriving value at every stage to realize the full potential of AI over time.
Making the industry more sustainable
As one of the most essential industries in the world, the steel industry is responsible for producing the materials that are used in everything from bridges to buildings to cars. The steelmaking process is also one of the most polluting in the world. The World Steel Association estimates that the industry is responsible for around 7% of global greenhouse gas emissions.
Industrial AI is being used to improve energy efficiency and significantly reduce CO2 emissions to help organizations with their net-zero targets. Industrial AI can also contribute with use cases for a circular economy. For example, Industrial AI can be
used to develop new methods of recycling steel waste or improve water re-use. Additionally, AI can inform quality control during the production process so that less scrap is produced, and rework is minimized.
Effective AI deployment
Steelmakers can effectively implement Industrial AI to predict optimal throughput speed for common steel-making processes, such as annealing, to maximize production, while maintaining quality and increasing yield. Let’s understand this better with the help of a real-world example.
A North American steel manufacturer used the Canvass AI platform to increase line throughput, while maintaining product quality.
This manufacturer caters to a wide range of industries such as aerospace, automotive, construction, defence, energy, infrastructure, and mining, among others.
The steel manufacturer wanted to apply AI and ML to improve its annealing process.

This process essentially heats and cools down steel coils to set points at different stages. This is expected to affect the material’s crystalline structure, thereby improving its mechanical properties, and increasing strength. Once this is done, samples are taken manually from each lot to measure their properties. This testing is a time-consuming process and presents an ideal use case to apply a predictive Industrial AI solution.
The Canvass AI platform was used to analyse the company’s historical data of the annealing process and generate Industrial AI models for prediction of product quality in real-time, essentially eliminating the manual testing process. Canvass’s AI platform was applied to help the company boost line speed by over 11% without impacting the quality of the final product. This, in effect, allowed them to increase yield by more than 9%. What is even more impressive is that this manufacturer was able to scale the Canvass AI solution and learnings to eight other processes in less than
In this article, Humera Malik* explores important ways in which Industrial AI is being used in the steel industry and the potential benefits that it can bring to the production teams.

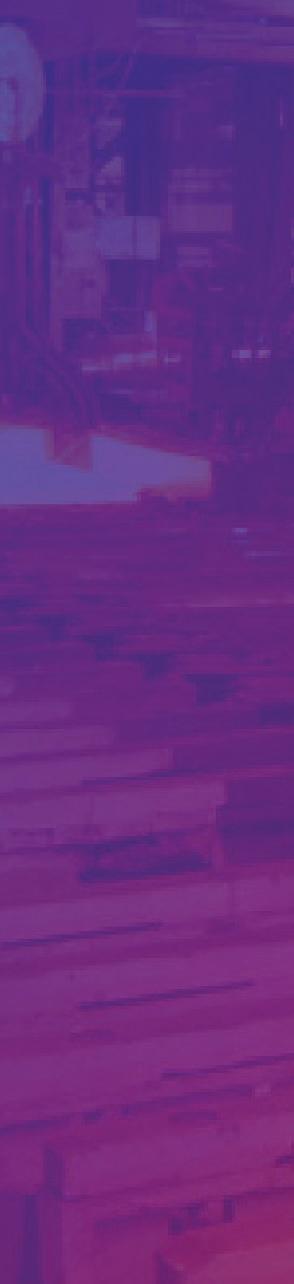
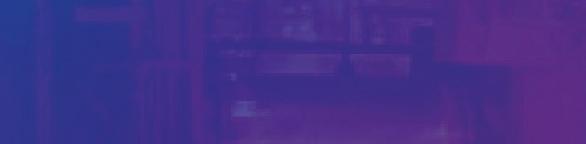
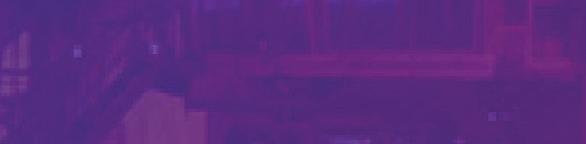
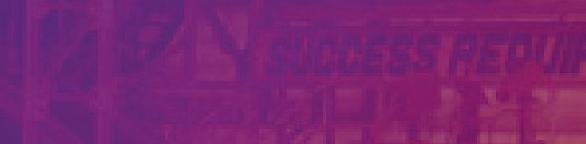
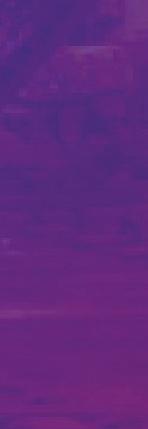

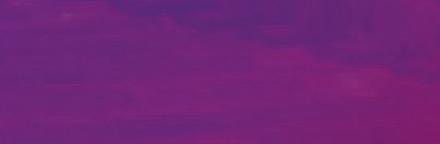
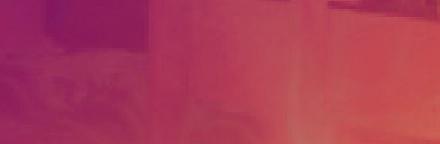
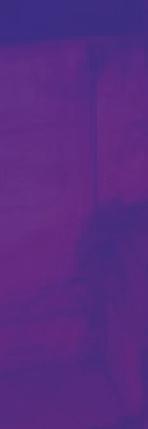
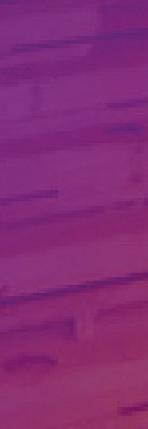

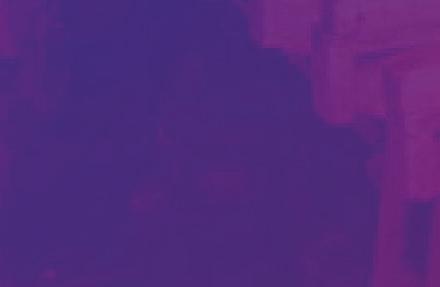
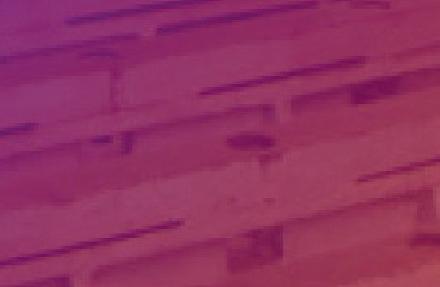
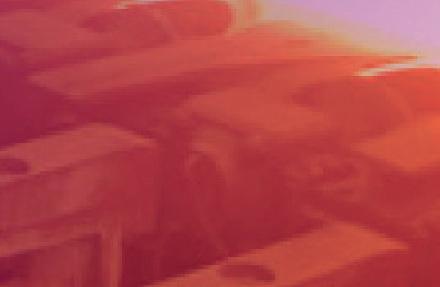
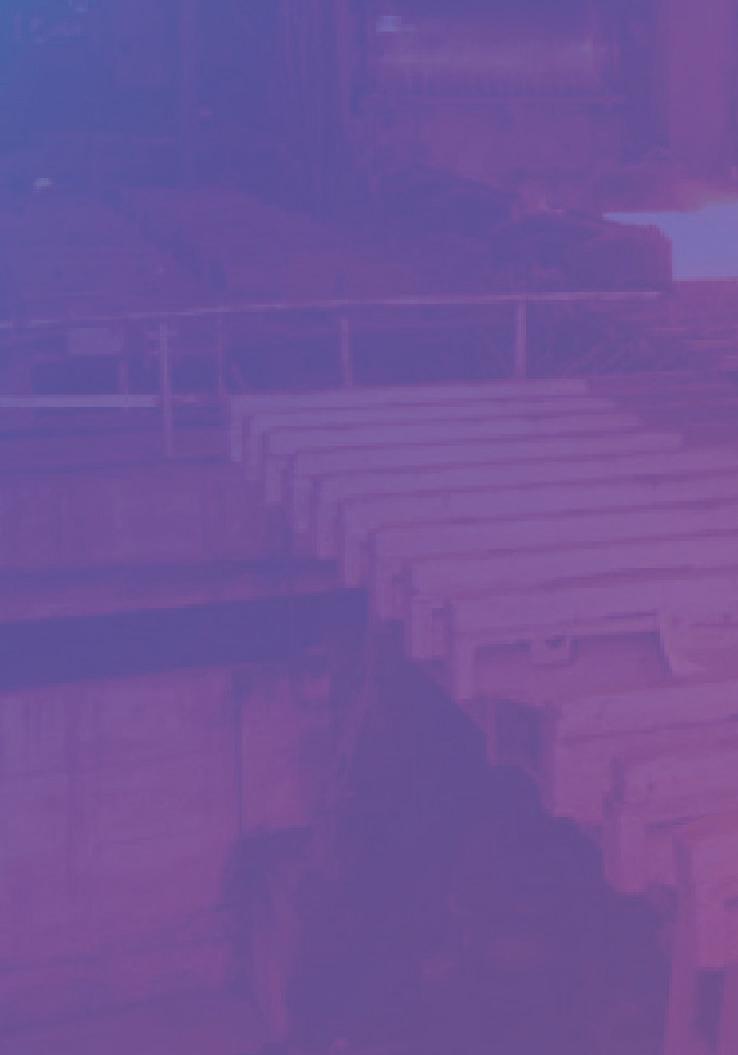
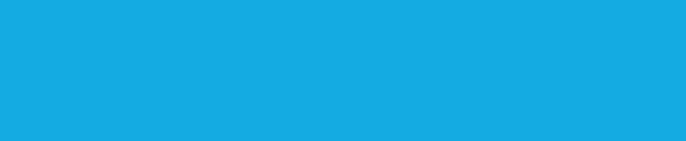
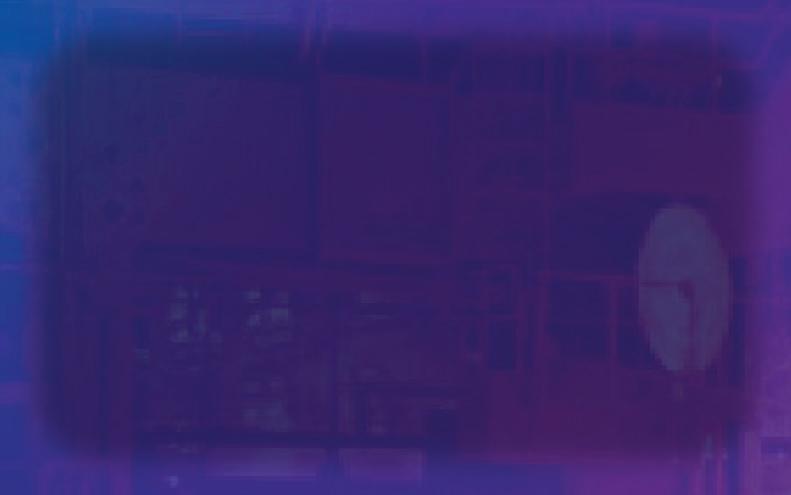
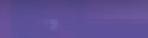


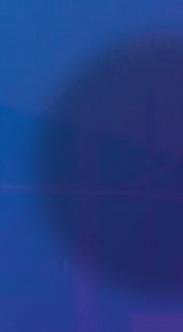

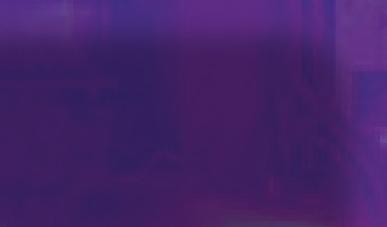

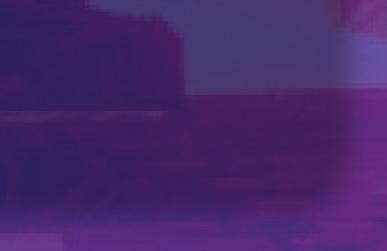

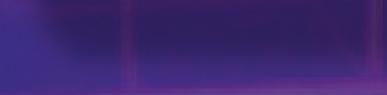





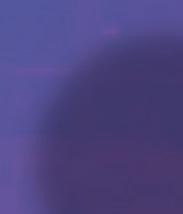

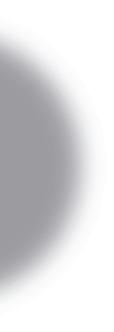
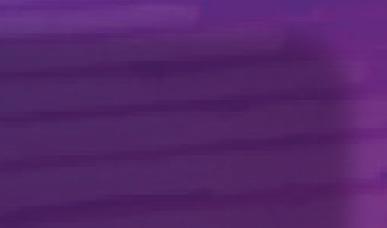
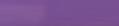

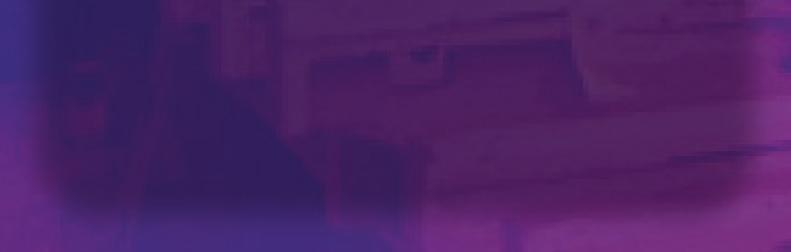

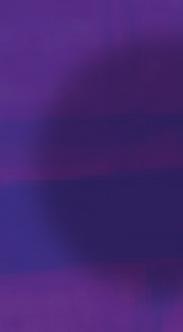
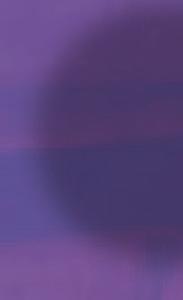

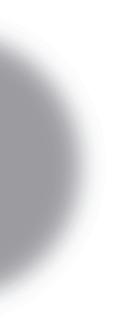
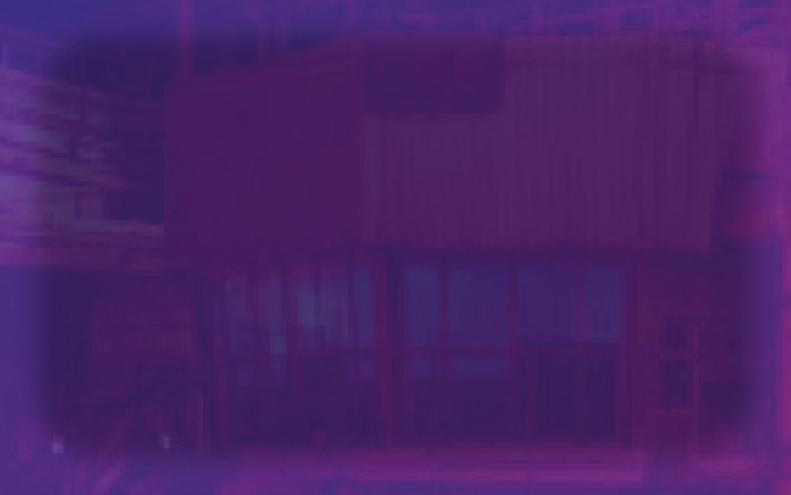

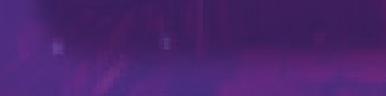
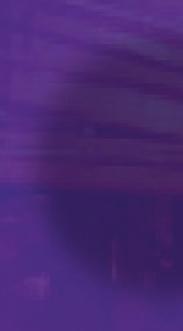


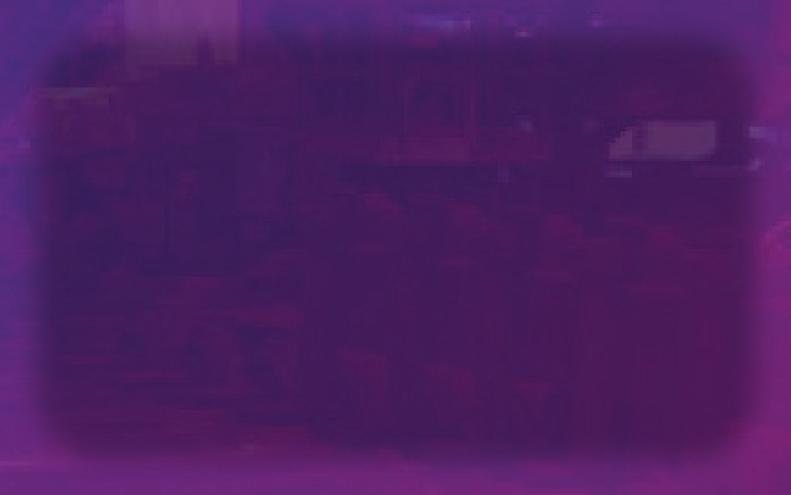

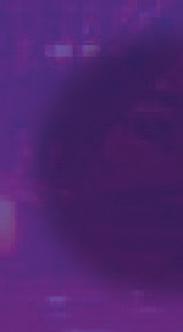

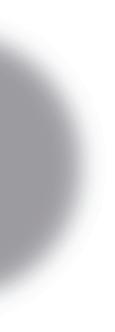
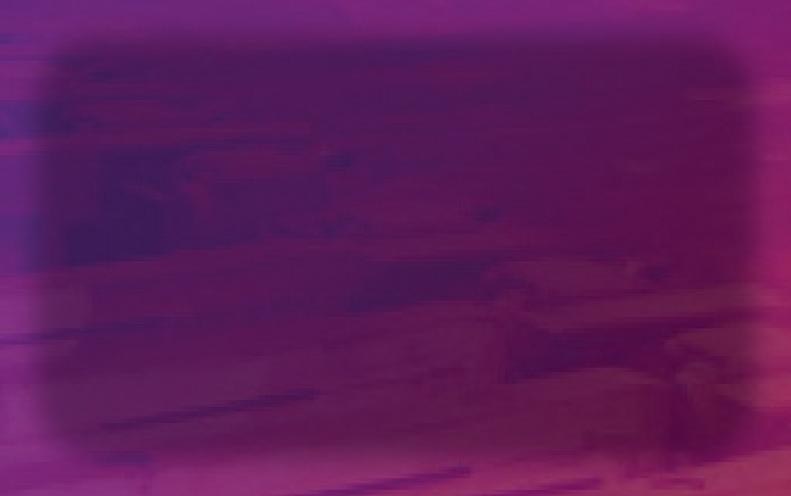
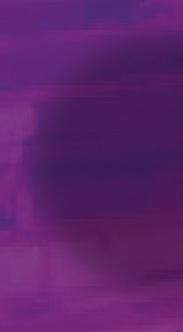
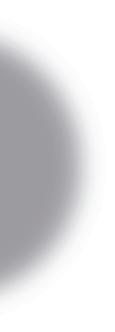
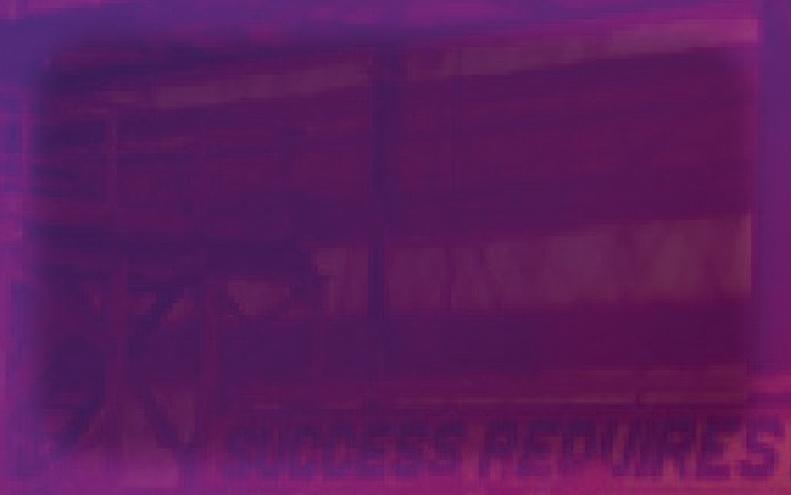

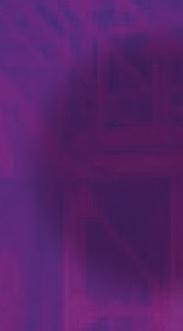

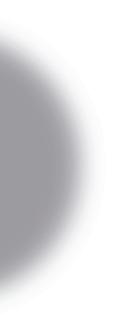
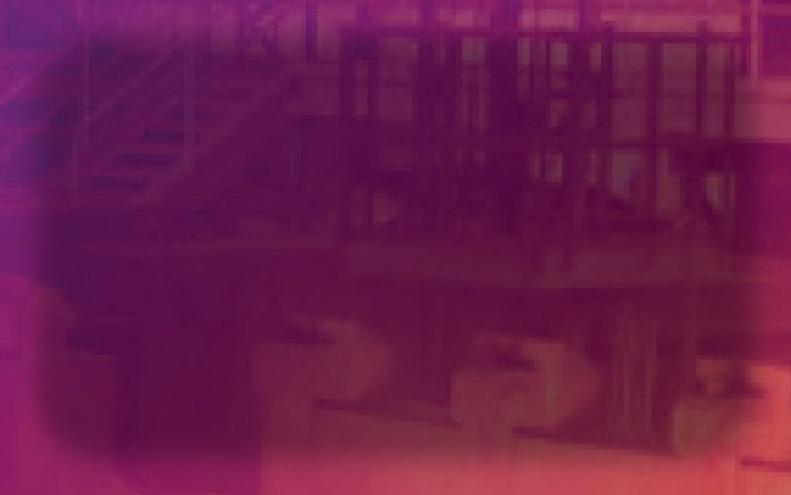
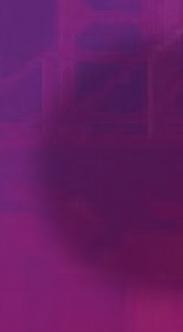

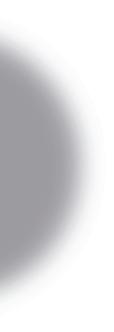
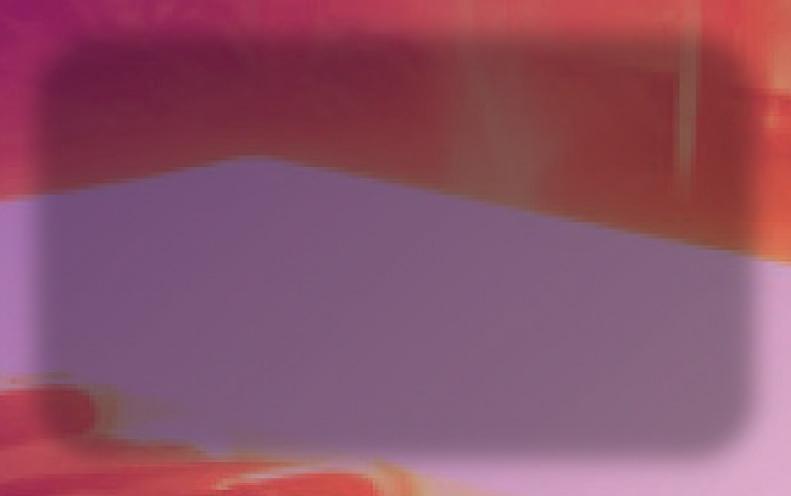

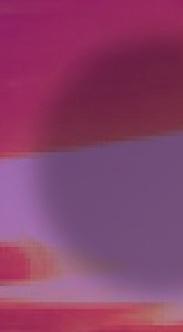
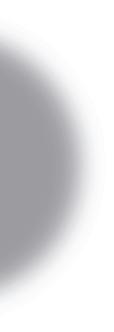
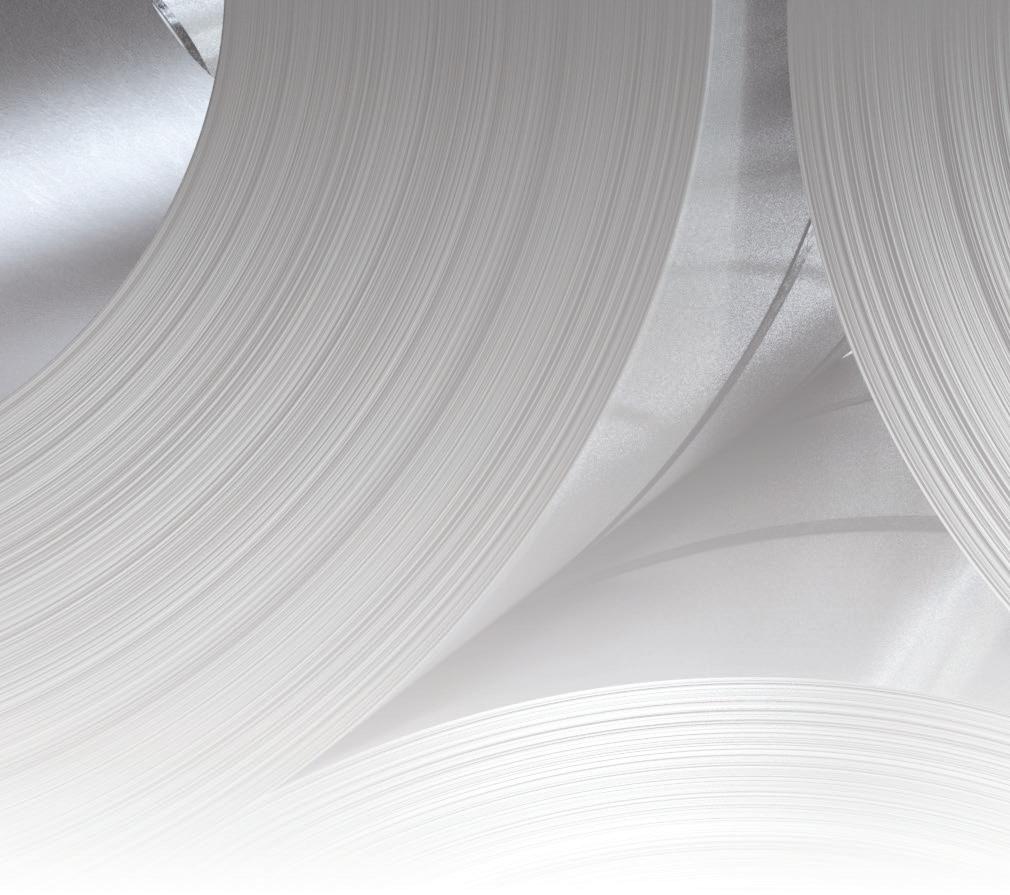
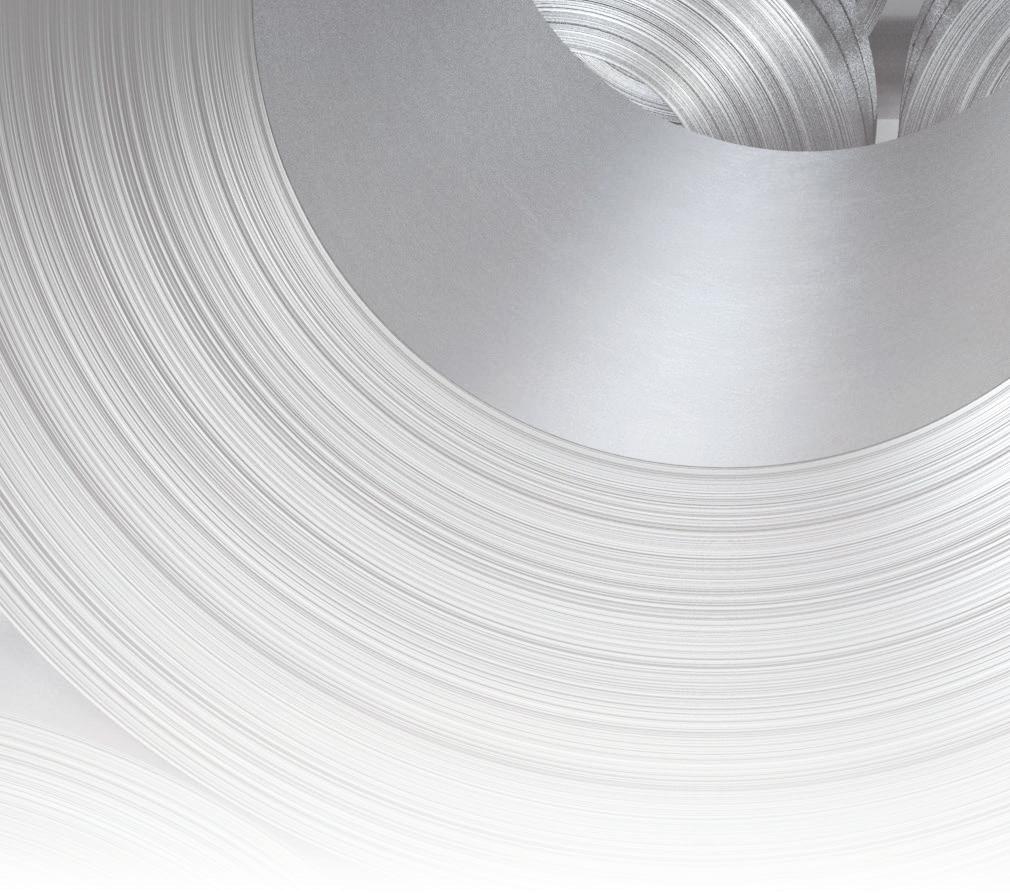
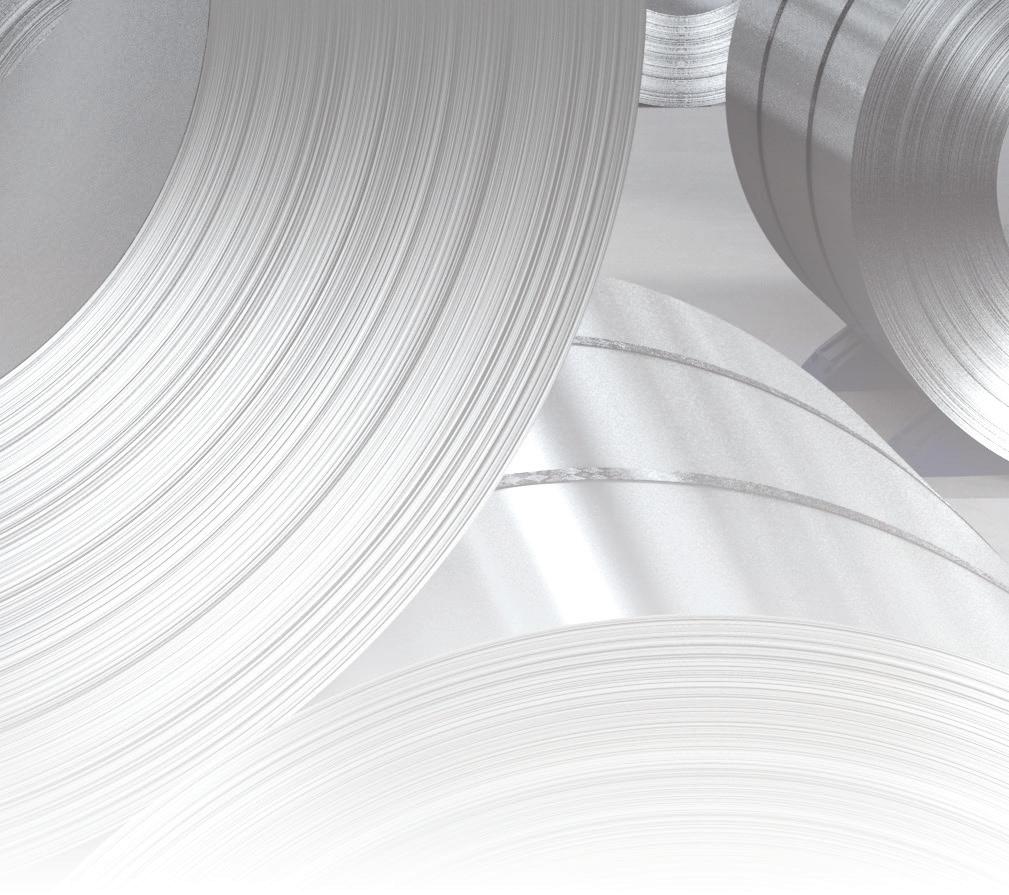
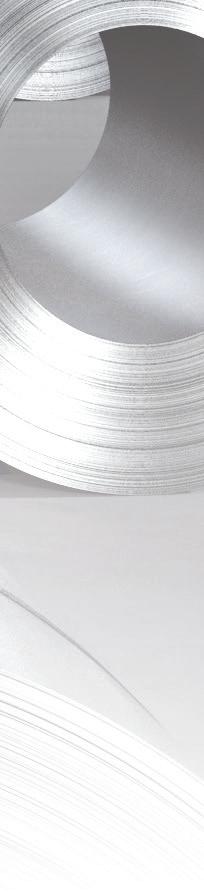
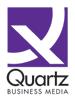
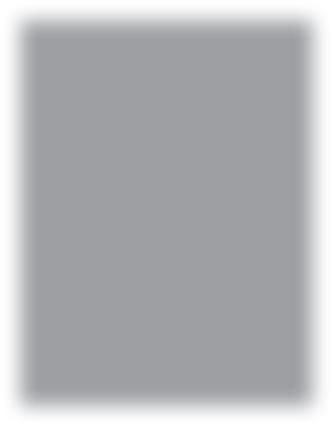
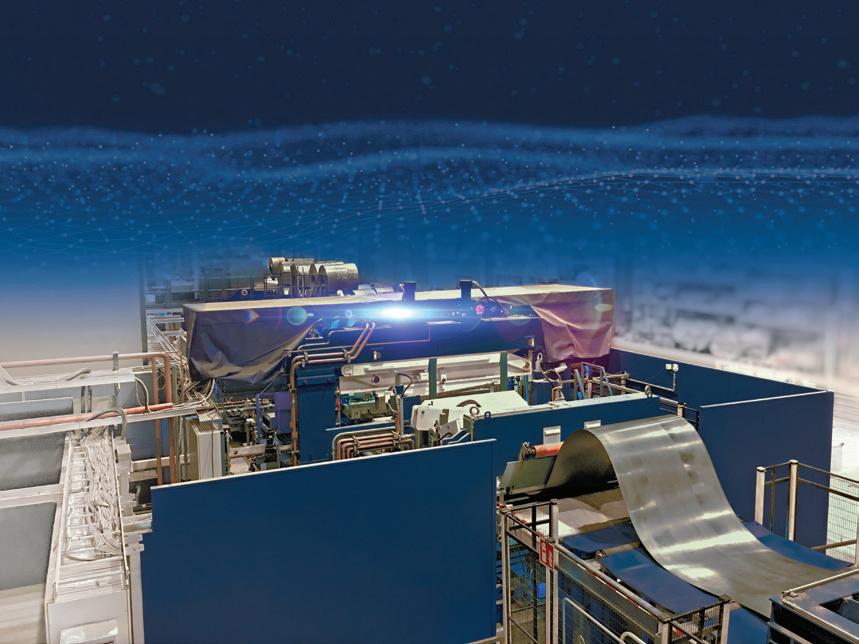

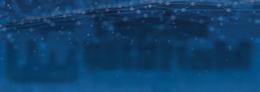



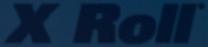



ARTIFICIAL INTELLIGENCE
a week.
In another example, the Canvass AI Platform was used to quickly develop an accurate model of the production train. With Canvass AI Live functionality, the model is able to capture real-time data and forecast production quality and yield up to two hours in advance. The timely and accurate forecasts are utilized by the control room operator to adjust process conditions to improve product quality and increase production resulting in revenue growth of $6m/year.
Canvass AI gives steel manufacturers all the capabilities they need to build efficiencies, reduce costs, and significantly improve yield. The Canvass AI journey is comprised of the following steps:
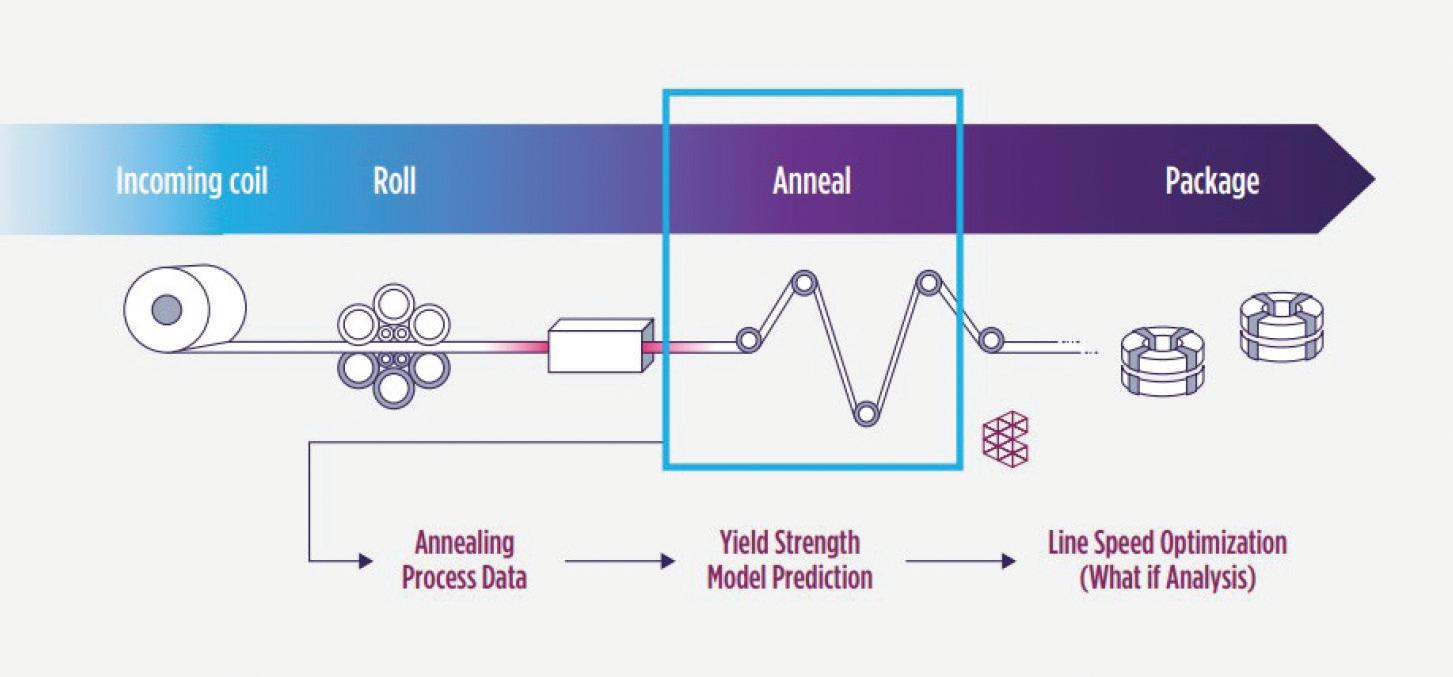
� Set clear objectives: It is imperative that manufacturers identify their business goal from leveraging Industrial AI. It can be enhancing the life of assets, increasing yield, or optimizing overall efficiency, or something else. This will allow the team to build a framework to start small, realize immediate value and expand to achieve their goal.
� Get your data ready: Industrial AI can start with lean data or rich data sets, and the first step of any AI journey is to assess data readiness and sufficiency. The quality and depth of the data will influence the value opportunity and types of problems you can
start to solve.
� Identify the right use case: Identifying the right use case sets the ball rolling for a successful Industrial AI roll-out. It helps demonstrate viability, obtain buy-in, and create favourable conditions for scaling.
A successful use case is one that helps the organization demonstrate clear value, has sufficient and relevant data to work with, augments your existing process or asset, demonstrates tangible results, and is scalable to other processes.
� Start small: As more and more industrial companies ramp up their digital investments, it is imperative to start small –with one asset or one work stream, derive immediate value, build trust in the AI solution with the workforce and then apply solutions in
other areas to expand on a large scale.
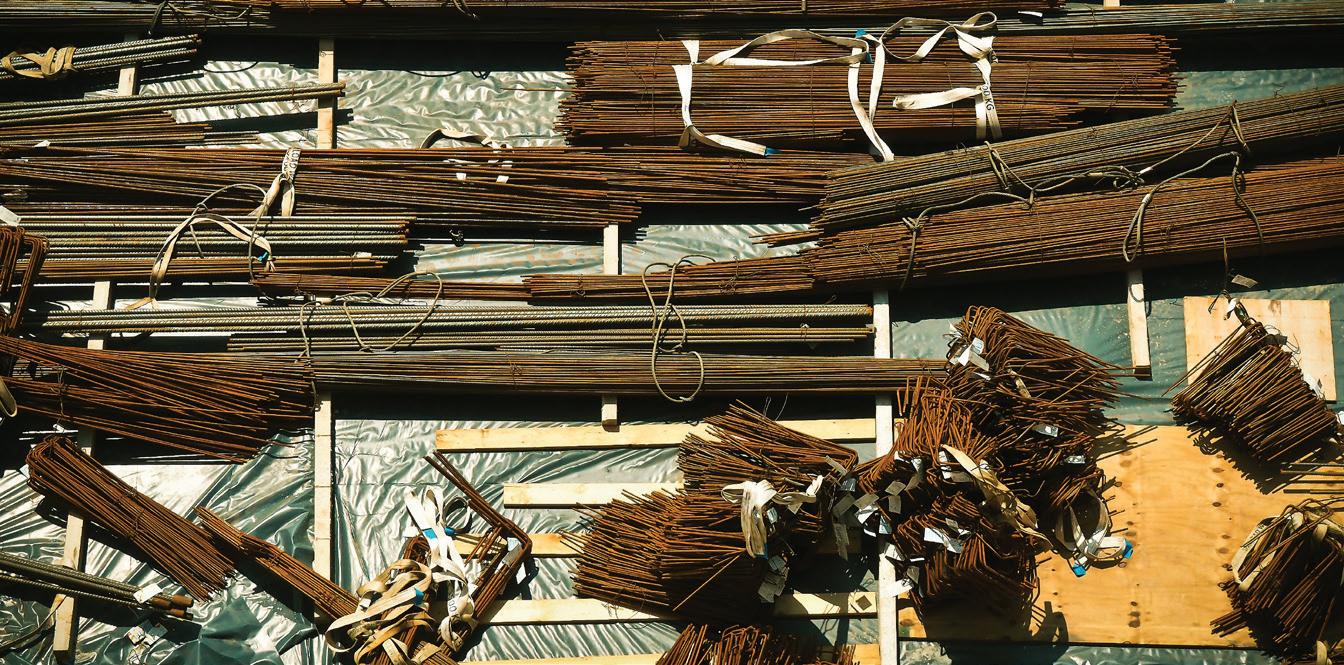
� Architect enterprise scalability: Architect enterprise scalability of the AI use case around the early learnings and success allows organizations to prepare the workforce, test their processes, validate the technology, and prepare the organization to scale-up across the enterprise. The people, processes and tools must all come together to achieve the end goal.
With Canvass’ AI platform, steelmakers can realize value in days instead of months. For example, a large North American steel manufacturer was able to complete an end-to-end implementation of an Industrial AI solution in just 45 business days to drive immediate value. �
This article describes the design, development and deployment of machine learning applications implemented at the Ori Martin Steel Mill in Brescia, Italy, as part of the Lighthouse Plant ‘Acciaio_4.0’ project in collaboration with Tenova. The project aims to create a Cyber Physical Factory at the Ori Martin Steel Plant by integrating the enabling technologies of Industry 4.0 in the steelmaking process.
By1. Introduction
Giovanni Bavestrelli*
In electric arc furnace (EAF) steelmaking, more than 60% of the total production cost corresponds to metallic raw materials (ferrous scrap). The different international steel scrap specifications define the scrap categories (qualities) by their origin, shape, and content in residual elements. These different grades of steel scrap are combined to produce the targeted carbon steel quality (scrap mix).
EUROFER estimated that scrap will play an increasingly important role in the EU steel strategy for the reduction of CO2 emissions (Fig. 1).
In this scenario, scrap characteristics are expected to drastically change and deteriorate due to repeated recycling and the recycling rate (steel is a permanent material) and consequent heterogeneity of available ferrous material (e.g., combination of steel with plastics and fibres, more complex joints, technical coatings, etc). Scrap availability as well as cost and quality factors require implementing actions focused not only on the scrap market but also on optimal charge preparation and improved process control. For reliable performance, it is necessary to assess the influence of scrap quality on the final product.
Dynamic and robust charge mix optimization tools have been applied to determine the optimal charge mix for each individual steel grade with respect to quality restrictions of the crude steel as well as the availability, purchasing costs, yield, and meltdown energy demands of the individual scrap types.
Today, digital systems and tools can be applied to support scrap yard management activities including scrap yard inventory
systems, which track the availability of charge materials in the scrap yard. The inventory database must be automatically updated every time a scrap delivery is entering the plant, and every time a scrap material has been loaded and charged to the furnace.
Some examples of RFCS projects seeking the best and most economic use of scrap in steel making operations are CONOPT SCRAP [7], FLEXCHARGE [8] , AdaptEAF [9], SUPERCHARGEEAF [10], and OptiScrapManage [11]
In addition, digitalization tools for supporting scrap yard management activities are under development, aiming to maximize the efficiency in the use of the available charge materials [12], [13], [14], [15]
II. Ori Martin steel plant
The Ori Martin steel plant produces wire rods and round bars by recycling metal scrap material. Every day around 100 trucks containing various types of steel scrap enter the plant, each truck carrying approximately 15 to 30 tons of metal scrap. This scrap is used to feed an EAF, which melts it into liquid steel up to a temperature of approximately 1660 to 1680 C°, about 25 to 30 times a day (each operation is called a heat), producing approximately 90 tons of steel per heat.
The shop uses several types of scrap materials, which are combined into recipes to create various types of products, mainly for the automotive sector, mechanics, energy, and construction. It is important that the scrap material is classified and tracked accurately from the time it enters the plant to the time it exits the EAF as molten steel, to control the characteristics and quality of the product.
The solutions presented in this article aim to improve the classification, tracking, and mixing of the steel scrap, from truck entrance to liquid steel tapping. The use of this information improves the profitability of the plant and the quality of the final products.
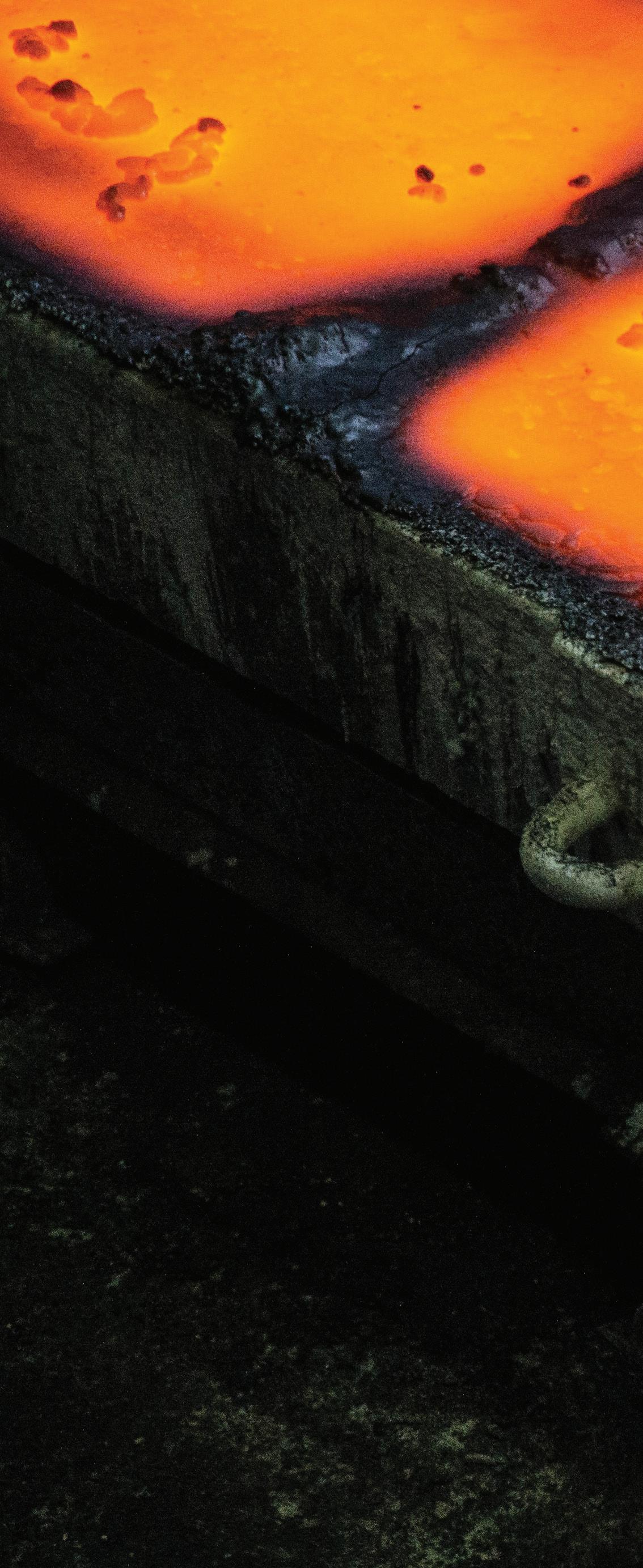
III. IOT platform and Tenova Edge Machine learning models need data. To gather data from customer plants worldwide, Tenova developed an IoT platform based on Microsoft Azure. This cloud platform can receive data from multiple plants, process it, store it in appropriate locations, and make it available for machine learning models as well as for interactive dashboards at Tenova offices and/or at customer sites to visualize KPIs indicating the performance, health, and maintenance status of the equipment.
Tenova’s customers in the steel industry are not all willing to open an internet connection
Building a smart factory
on the Tenova EDGE device in the plant. The communication with plant automation is based on RabbitMQ, an open-source message broker.
IV. Automatic metal scrap classification
When a truck enters the plant, it is weighed, scanned for radioactivity, and a picture is taken of its load. Then the load is discharged in the scrap yard and inspected to confirm its type and quality, making sure it matches what was expected from the purchase order. This is a manual operation and involves skilled technicians.
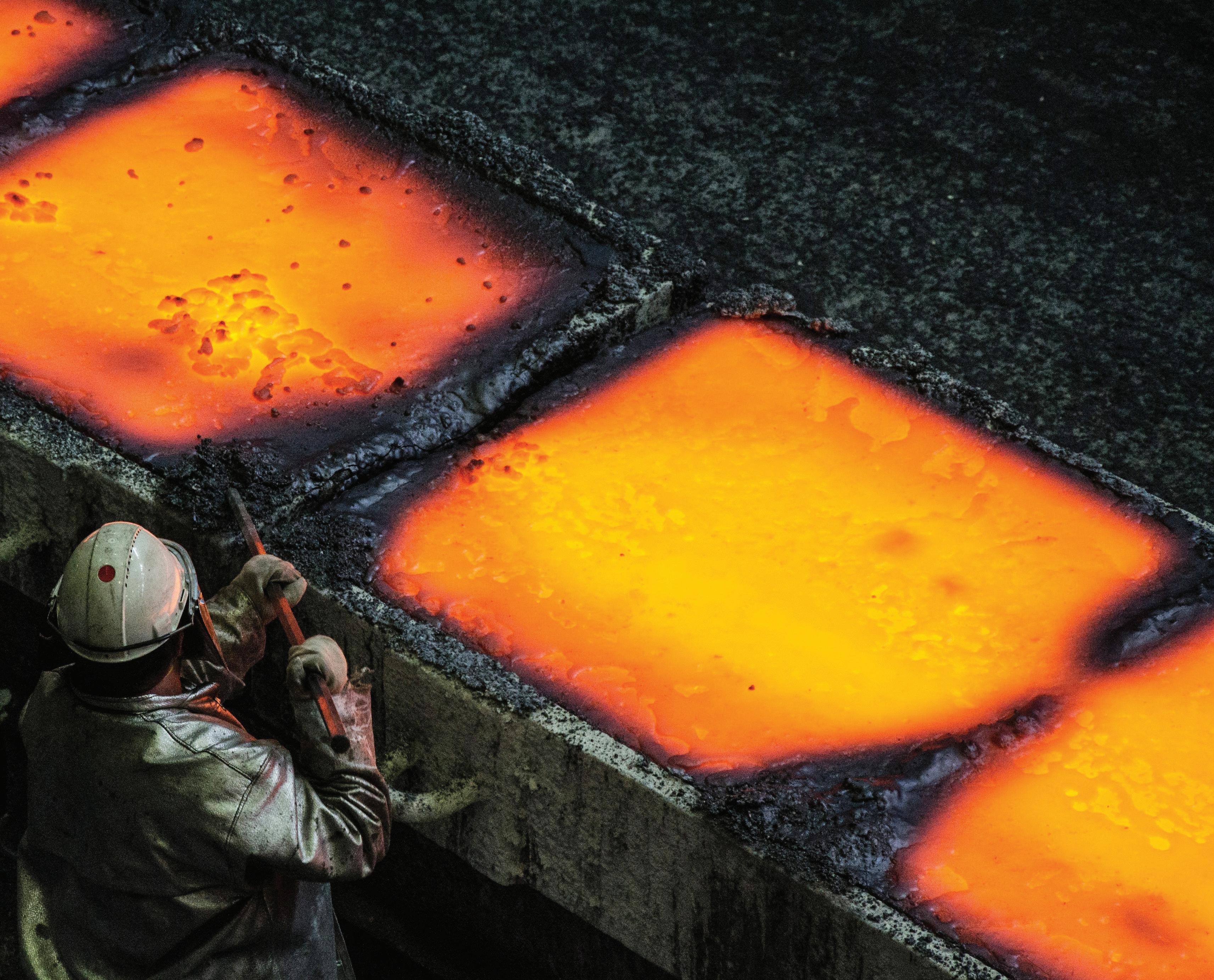
from their plants to the cloud, and they would not accept the correct operation of their plants to depend on a functioning internet connection. Moreover, the models would need to respond in near real-time. Therefore, even where an internet connection is made available, as at Ori Martin, the models must be deployed on-premises.
To this end, Tenova developed an edge
device, called Tenova EDGE, which is a local PC implementing a microservices architecture based on Microsoft IoT Edge, with dedicated software developed by Tenova. This device is used to read data from plants and send it to the cloud as well as an environment where to deploy models built and trained in the cloud and run them on-premises. The models are deployed inside Docker containers
The shop typically operates using nine types of scrap material, as shown in Fig.2
Over the course of the project, more than 50,000 images of trucks entering the plant were gathered and stored on the cloud. Some of these images were used to train machine learning models, using Convolutional Neural Networks (CNN), to automate the classification of the scrap
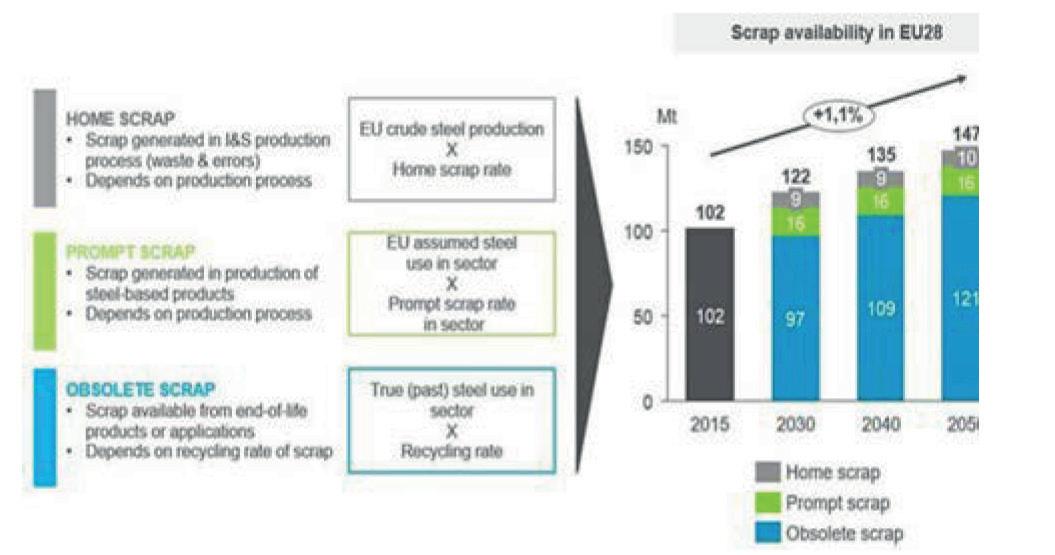
material, as an aid to technicians by highlighting possible mismatches.
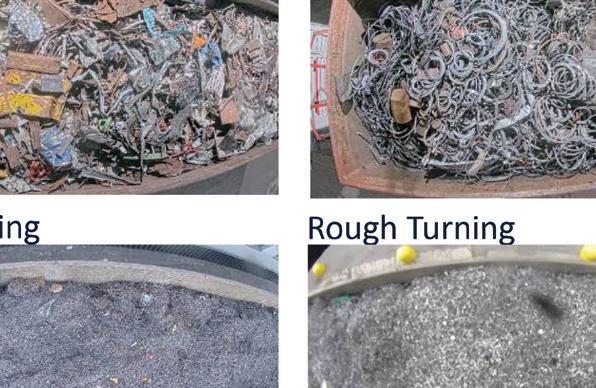
The first models were simple convolutional neural networks designed from scratch, training on a few thousand images. The models were written using Python, Keras and TensorFlow [3]. The image ‘labels’ (i.e., their target classes) were taken from a database already present at the plant, as each purchase order had already been checked and tracked.
The initial results highlighted a few problems. In the afternoon, especially in winter, the images were often too dark, making classification difficult. This was easily solved by installing an LED light. A somewhat harder problem was that sometimes the trucks were positioned incorrectly under the camera, or they were covered, so the algorithms could not learn to classify the load, and risked learning to classify the truck, the cover, or issue random guesses. This was harder to avoid because it would have involved changing procedures in the plant. It was addressed by creating two new categories for ‘misplaced’
truck and ‘covered’ truck, as shown in Fig. 3, separating their images, and training the models so that they could identify such cases instead of trying to guess their load.
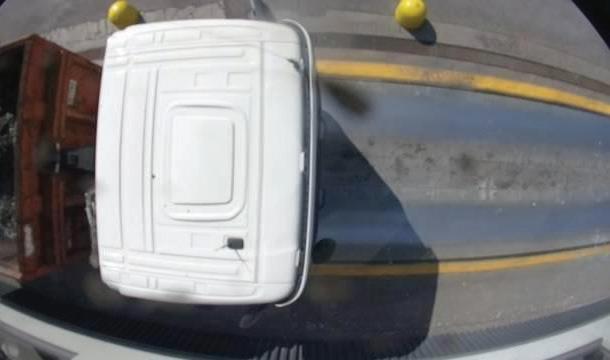
Separating the images of misplaced trucks and covered trucks
Trucks were easy with a few thousand images at the beginning of the project, as a single person could do it in a reasonable amount of time. It turned out to be more difficult with tens of thousands of images, due to the manual work of identifying them, and the different standards that could be used to identify them, especially if more than one person had to do it. For instance, should an image like the one in Fig. 4 be classified as ‘misplaced’ or as ‘pantograph’? These types of decisions should be taken consistently, not to confuse the learning algorithms.
When the number of truck images grew to tens of thousands, as it became more difficult to separate misplaced and covered trucks, we adopted an image search algorithm to find them [6]. We used an open-source pretrained
MACHINE LEARNING
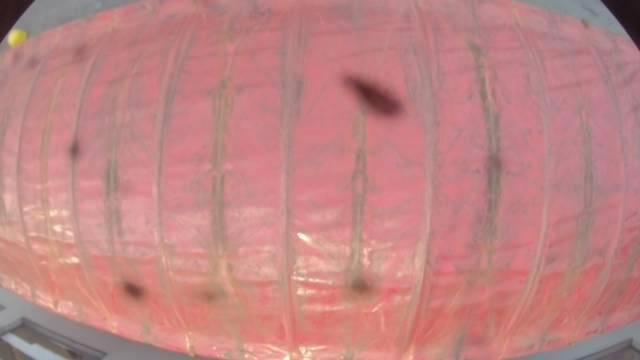
convolutional neural network to extract features from all the images in the dataset, then used a few sample images of misplaced trucks (or, separately, covered trucks), which sorted all the images in order of their growing difference from the sample images, and visually selected the images from the top of this resulting list. This allowed us to quickly see many images that were likely to be of the types we were looking for, without having to scan through thousands of images of everything else. This approach was inspired by the way google image search works.
Another problem was that some images were very poor quality, due to bad image focus, wet lenses, movements of the camera, or other events that cannot be controlled. These images were often classified incorrectly, but the real problem was that they confused the learning algorithms, lowering its quality. We decided to reduce the number of images to around 1,000 for each scrap category, so that it was easier to visually select good images, making sure the dataset was representative and clean.
Once we had a good dataset, consisting of 11 categories, around 1,000 good images per category (except for the covered trucks, of which we had much fewer images), we separated the images into training set, validation set and test set. We trained several different convolutional neural networks, with different hyperparameters, to classify these images, and selected the one yielding the best accuracy on the validation set, then tested its accuracy on the test set, as is standard practice in image classification projects.

Among the different convolutional neural networks tried, some were simple deep learning networks designed from scratch, and some were designed on top of state-of-theart open-source pretrained neural networks, using a technique called transfer learning [3]. As anticipated, the latter technique gave better results.
The accuracy achieved on the 11 categories was around 85%. The main problem was that it was difficult for the algorithm to differentiate between the three types of metal sheet, and between the two types of turning.
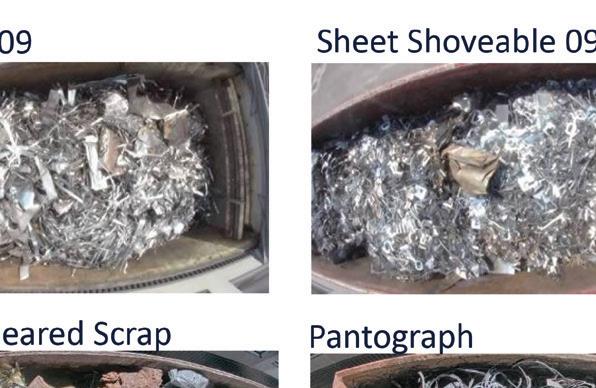
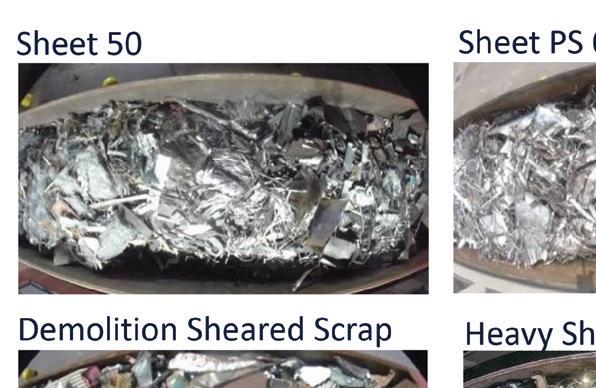
We analyzed the images that were classified incorrectly by the classification algorithm, sent them to Ori Martin‘s
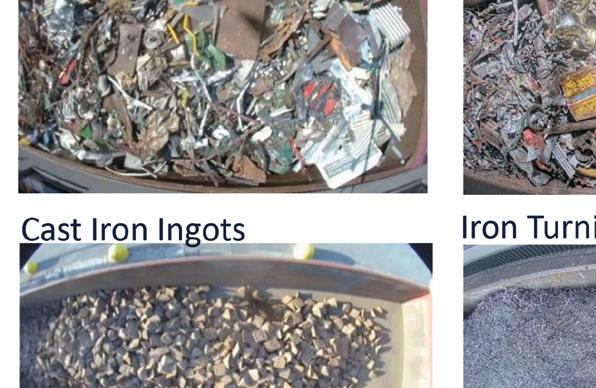
technicians, asked them to classify them manually, and compared the results. We realized that the technicians made similar mistakes in classifying the images, and this could be due to several reasons. One is that it is an objectively difficult task to differentiate between these images, as some of the scrap materials are very similar to each other. Another reason is that operators are used to classifying material on the ground, not from images, and it is easier to make mistakes by only seeing the image, as the algorithm does. This problem turned out not to be too serious as two of the metal sheet categories were treated the same in production (i.e., it was not necessary to differentiate between them) and the same was true for the two types of turning. Considering the three metal sheet categories as one and the two turnings as one, the accuracy on the resulting eight categories went up to approximately 95%.The model was deployed inside the Tenova EDGE device in the Ori Martin plant. When a truck enters the plant, a picture is taken, sent to the model, classified, and the response is sent to the technician’s tablet, so the technician is advised about the type of material expected in the purchase order and the type classified by the model. He can then make his decision.
The model has been in production for over a year. As it is used and its accuracy is evaluated in production, we will consider different possible uses of the model in the future.
There are also aspects that this model does not address, like the fact that it is based on an image of the load from the top, when there could be different material below the surface that cannot be seen until the material is unloaded. This is not critical now as the model is only used to advise operators, who also observe the material once it is unloaded on the ground.
V. Identification of bulky material on the Consteel®
The Ori Martin plant uses Tenova’s Continuous Charging System (Consteel®) to load the EAF. The Consteel® system continuously feeds and pre-heats the metallic charge (scrap, pig iron, etc.) to the EAF, while simultaneously controlling gaseous emissions.
The charge is loaded directly from the scrapyard to the charge conveyor, where it is automatically and continuously preheated
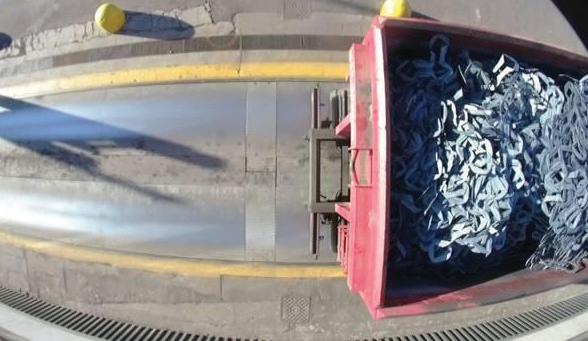
and conveyed to the EAF. Once preheated, the charge falls into the EAF where it is continuously melted by the liquid steel. This permits constant flat bath operation, a key advantage over conventional batch processes where scrap is melted directly by the electric arc. If the scrap material on the conveyor is bulky and heavy, it might risk touching and damaging the electrodes when falling into the furnace. To prevent this, Tenova developed a machine learning solution aimed at identifying such material on the Consteel® conveyor, giving operators a chance to lift the electrodes before the material enters the furnace.
The first task was deciding what type of materials are to be considered as bulky and dangerous and, therefore, should be
identified in advance. After discussion with plant technicians, we concluded that the two most common types of dangerous materials that could end up on the Consteel® were wire rods and large metal sheets, examples of which are in Fig. 5 and Fig. 6
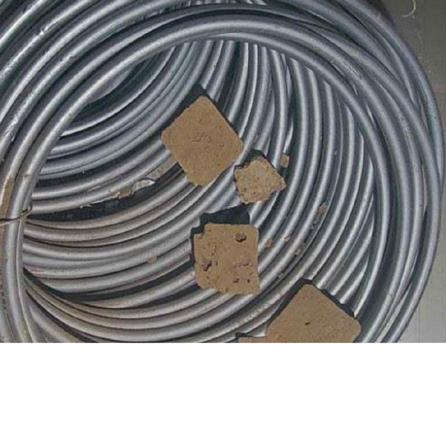

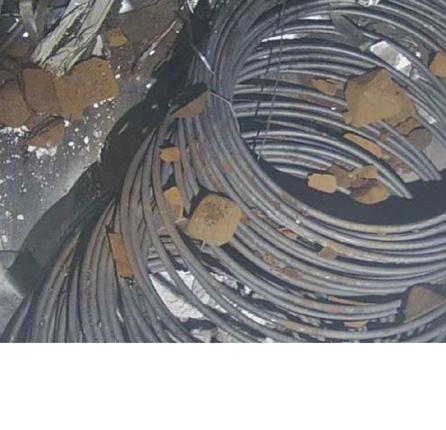
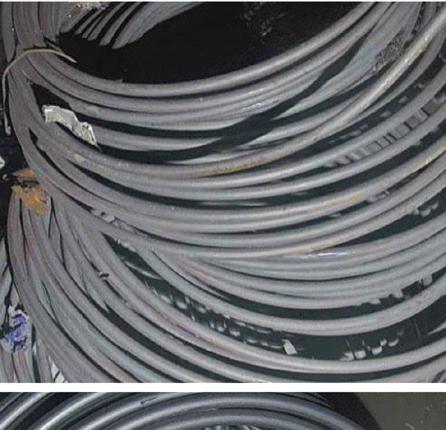
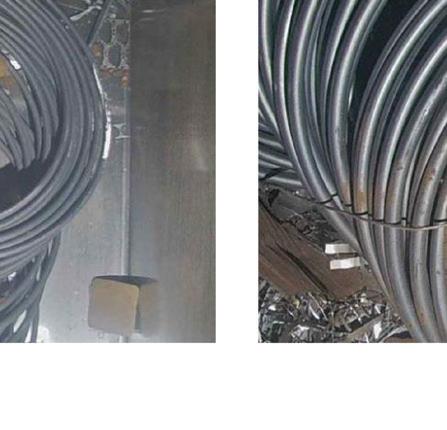
We had placed a camera above the Consteel® conveyor at the start of the project. The camera quickly gathered hundreds of thousands of images. The problem was that none of these images were labeled i.e., we did not know which images contained wire rods or metal sheets, and such materials were relatively rare. A human scanning one hundred thousand images looking for wire rods or metal sheet is a frustrating, time consuming and error-prone job. Instead, we applied the same method we had used to find
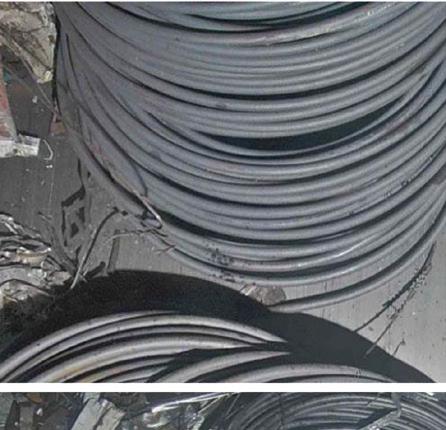
images of misplaced and covered trucks in the previous project [6]
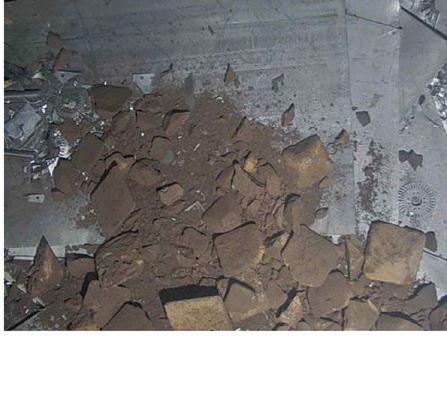


We downloaded more than 100,000 images, used an open- source pre-trained convolutional neural network to extract features from all images, then used a sample image of a wire rod and sorted all images in order of their difference from the sample image. From just one image of a wire rod, we were quickly able to find hundreds of images of wire rods and repeating the process with different sample images we found even more.
We used the same process for metal sheet images, ending up with about 1,000 images of wire rods, 1,000 images of metal sheet, and over 100,000 images which were considered ‘other’. In theory these ‘other’ images should not have contained
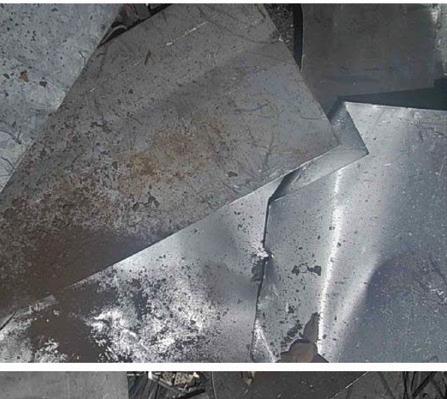
bulky materials, but we could not be sure unless we looked at all of them, which was not something we wanted to do. This was also a very imbalanced dataset, not ideal for training a supervised machine learning model. We had to reduce the number of ‘other’ images, to balance the dataset and to be able to analyze them to make sure they were good images. Selecting a random sample of them would have been far from ideal. We used an unsupervised learning technique instead, clustering [3], to divide the hundred thousand images into 1,000 clusters, based on the same features extracted for searching wire rods and metal sheets. Fitting this model, we separated the ‘other’ images into 1,000 clusters, and then selected a good representative image for each cluster.
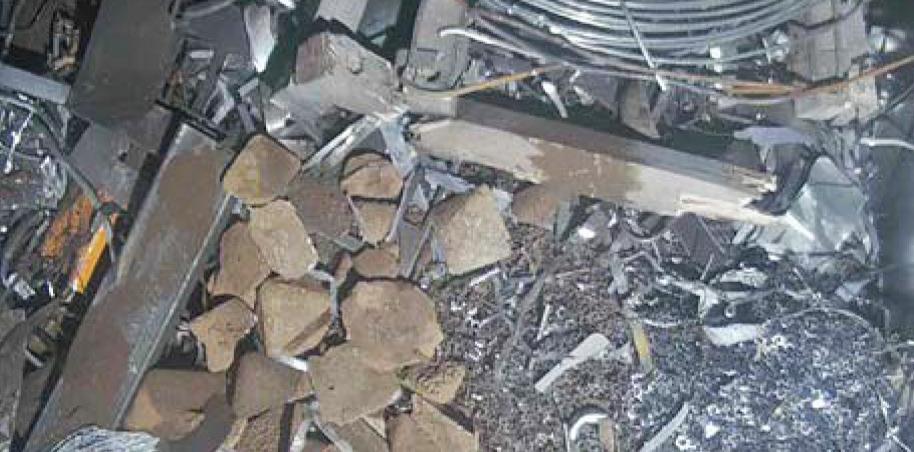
MACHINE LEARNING
Some of these images ended up containing more wire rods and metal sheets, and these were moved with the rest of them, while the remaining ones created the ‘other’ category of images.

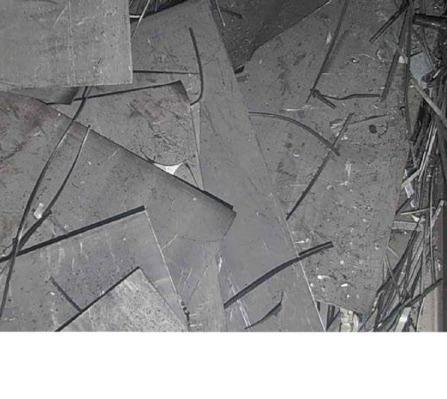
At that point we had a reasonably sized, balanced dataset of three categories of images: wire rods, metal sheet, and everything else. We divided them into training, validation, and test sets, and trained several convolutional neural networks with different hyperparameters, selecting the best performing ones on the validation set, and measuring their accuracy on the test set. We only used transfer learning, designing our networks on top of pretrained open-source networks as we had seen it worked better in the classification of scrap material on trucks. The results were reasonably good. We then looked at the images that were misclassified, one by one, which is always a useful exercise when evaluating the performance of an image classification algorithm, more so than just looking at a percentage of accuracy. We saw that in many cases, for instance, the wire rods were at the border of the image, like shown in Fig. 7
Such images could be classified as ‘wire rod’ or as ‘other’ without major problems (the previous or subsequent image would probably contain more of the wire rod and thus could be classified more accurately), but it should not be included in the training set, as it might be confusing for the algorithm. It does contain a wire rod at the top of the image, but most of the image is not, so how do we label it? We concluded it was better if such images were excluded from the training set, so that training images were clean and represented good samples of each type. On the other hand, if included in the test set, such an image could increase the number of misclassifications, if it is labeled as ‘wire rod’ instead of ‘other’ or vice versa, whereas in such conditions we did not really care either way. We looked at all the images in the three sets and removed the ones that were somewhat ambiguous. Working on the dataset, making sure it was clean and contained good representative images, improved the predictions more than trying different models and fiddling with their hyperparameters.
At that point we had close to 1,000
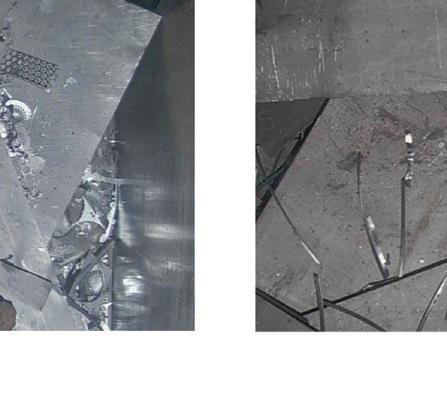
MACHINE
LEARNING
images of each of the three categories, these were good and representative images, and we trained the models again measuring their performance, which was above 95% on the test set.
We integrated the model with the furnace control system and deployed it into production on the Tenova EDGE device, monitoring its performance, and it worked well for a while. It warned operators when bulky material was on the Consteel®, giving them an opportunity to observe them and decide if the electrodes should be raised to avoid damage. Then one day the operators told us that our system kept warning of bulky material on the Consteel® while the Consteel® was empty!
This was a serious ‘false positive’ error, as it is normal for the Consteel® to be empty at times, and this is certainly not a dangerous event. The problem was that the bottom of the Consteel® is made of metal sheet, as you can see in Fig. 8, therefore the image was classified as metal sheet, and metal sheet is considered bulky and possibly dangerous.
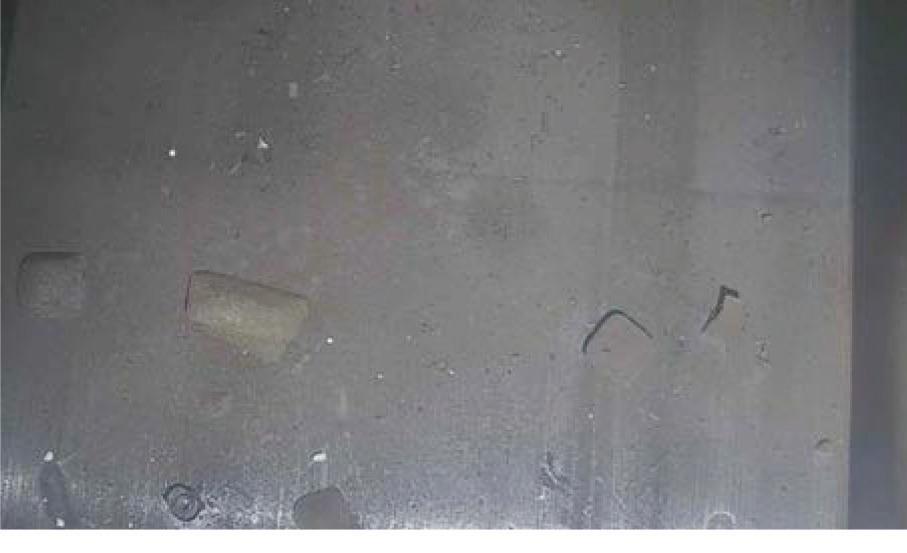
To solve this problem, we created a new category of images, representing “Empty” Consteel®. We found one image of it, and with the image search method described above, we looked for many more, selected the best images one by one, and retrained the model with the four categories.
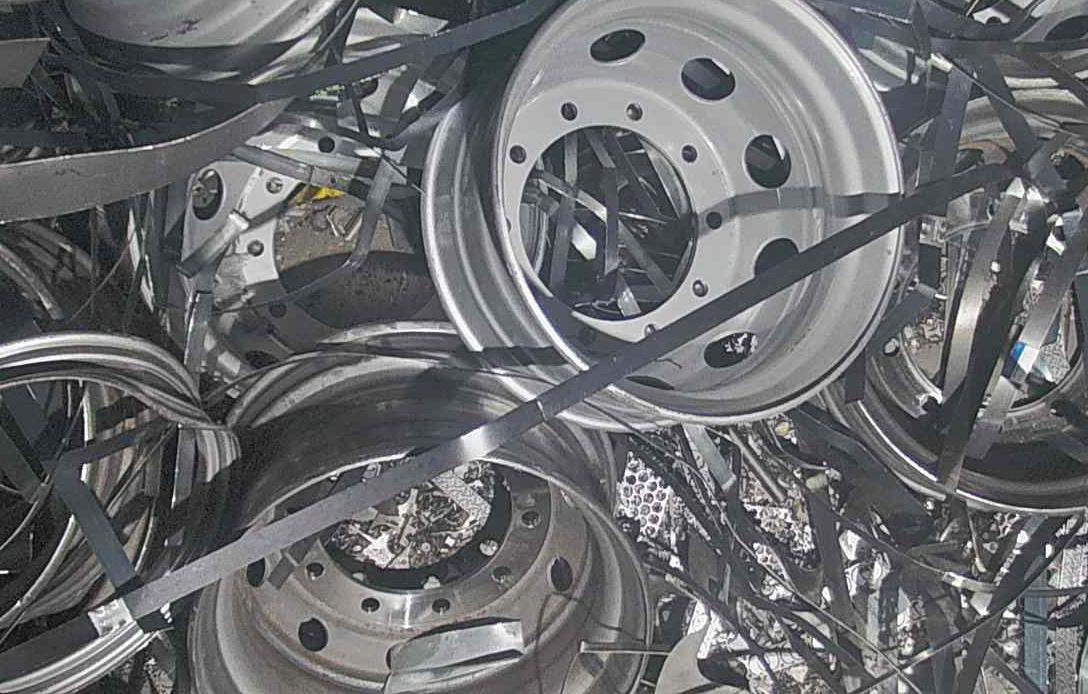
Observing the model performance in the following months, we noted that sometimes wheel rims were classified as wire rods, probably due to their similar round curves, as you can see in Fig 9. Since wheel rims are not bulky nor dangerous, these would turn out as false positives too. Once again, to improve the performance of the classification algorithm in the case of wheel rims, we searched for other wheel rims in the dataset of images, and found over 100 of them, we created a new class : ‘wheel rim’, and trained the model on the resulting five categories.
This model has been in production for several months. The accuracy of the predictions is above 95%. The confusion matrix of predictions on a small test set is shown in Fig. 10. There are few images in the ‘Empty’ and ‘Wheel rim’ categories, but the accuracy seems reasonable. The model is integrated with the furnace control system and warns operators when bulky material is present.
VI. Finding correlation between loaded scrap material and tramp elements in liquid steel
To be able to control the quality of an EAF’s production, it is very important to track the scrap material going into each heat and relate it to the quality of the liquid steel produced. In other words, we need to know accurately what scrap material was used and relate it to the chemical analysis of the liquid steel. To do this, Tenova developed an application using a laser scanner to track the volumes of scrap material stocked in each area of the scrap yard, and adopted a new weighing algorithm on the crane, able to weigh the load with a maximum error of
2% while the crane is moving. Accurately weighing the load while the crane is moving is a complex problem that requires advanced mathematical modeling. Once the scrap load is melted in the furnace, the next necessary step is measuring the quality of the final product. To this end, as part of the production process in Ori Martin, a chemical analysis of the liquid steel is always performed after each heat.
Some chemical elements are present in the scrap material but are considered ‘tramp’ elements (pollutants) and their levels cannot be removed from the liquid steel; therefore, to achieve the final steel composition required in the cast product, the quantity of such elements
Québec City, Canada
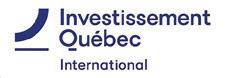
Future Aluminium Forum 2023: Meeting the Demand
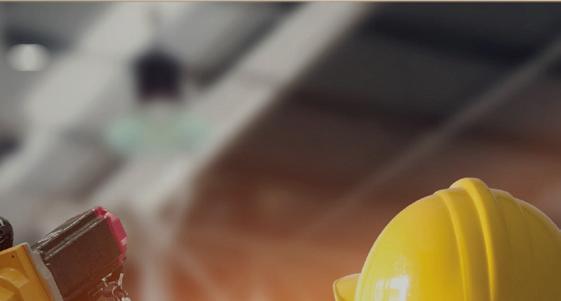
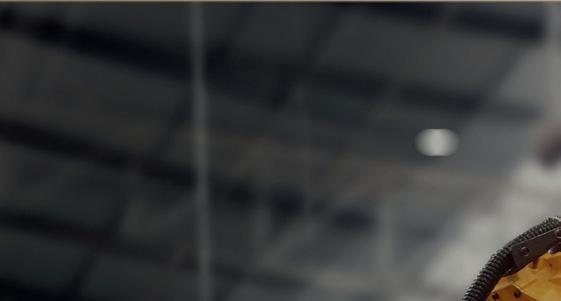
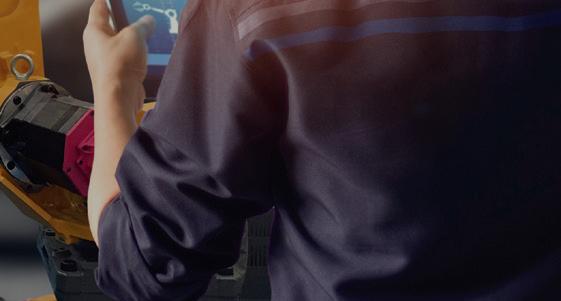
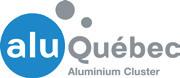
With global aluminium demand projected to increase 40 per cent by 2030, the aluminium sector will need to produce an additional 33.3 Mt – a reality only possible through the inception of streamlined technologies, digital manufacturing and sustainable solutions across the supply chain.

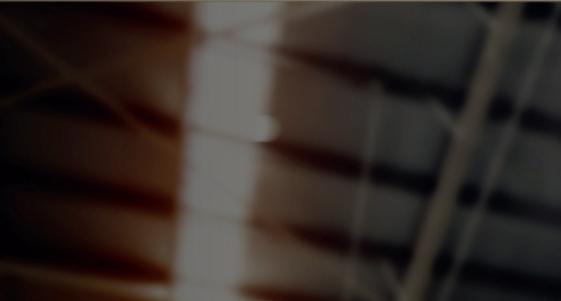
Join us at the Future Aluminium Forum to meet manufacturers rising to the challenge, focus on the opportunities that growth will bring, and prioritize time for your own learning and networking.
To find out more about speaking contact:
Nadine Bloxsome, Conference Director nadinebloxsome@quartzltd.com +44 1737 855115
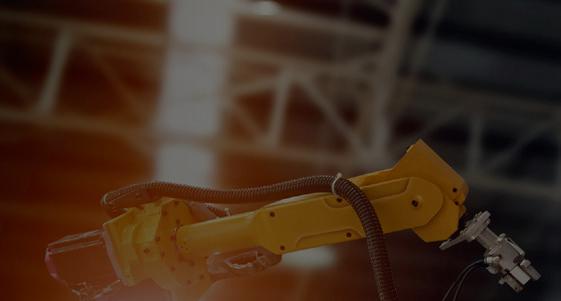
To find out more about stand and sponsorship opportunities contact: Nathan Jupp, Commercial Sales Director nathanjupp@quartzltd.com +44 1737 855027
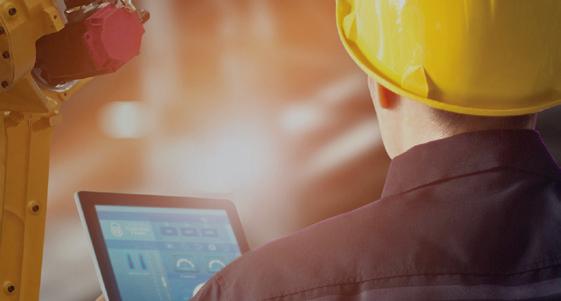
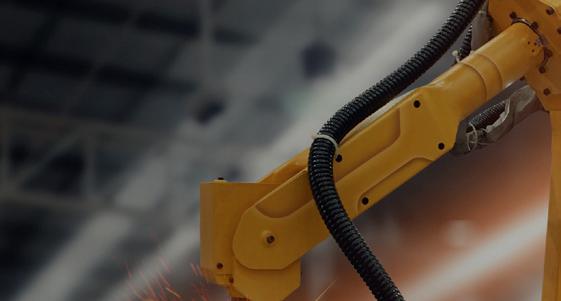
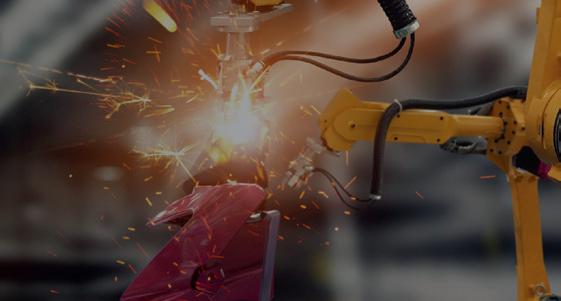
In 2023, the Forum will return in a live format to Québec City, a region where the aluminium industry has existed for more than a century and is now seeing rapid change and collaboration between manufacturers, processors, suppliers and OEMs. Register
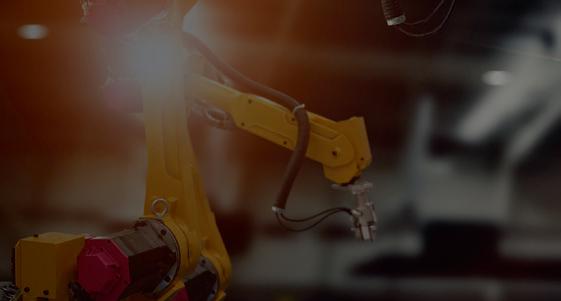
MACHINE LEARNING
needs to be controlled in the raw materials. Some of the key elements are copper (Cu), nickel (Ni), arsenic (As), tin (Sn) and molybdenum (Mo).
The project was to use machine learning to find the correlation between the combination of supplier and scrap material type and the percentage of each tramp element in the supplied scrap.
We had data relative to over 9,000 heats, and for each one we knew the total weight of each scrap material that went into the furnace, which we could calculate because we tracked every single loading operation by the crane, and the chemical analysis of the resulting liquid steel, which included the percentages of the five tramp elements mentioned above.
Since the tramp elements remained in the liquid steel, we could use a linear regression model to find the coefficients (percentages) of copper, nickel, arsenic, tin and molybdenum present in the scrap for each combination of supplier and material.
There were 11 different scrap materials and 33 different suppliers, for a total of 81 combinations of material and supplier. Not all combinations of material and suppliers were frequent enough to be used reliably in a regression model, so all heats containing such rare combinations were removed from the dataset.
A lot of work went into creating the dataset, introducing new features representing physical quantities important in the process, cleaning the data, identifying outliers, taking into consideration the liquid heel that remains in the furnace from each previous heat, and whose contribution to the tramp elements should be accurately calculated.
The result of applying the linear regression models was estimating the level of each tramp element present in each material supplied by each supplier. Naturally, this was a statistical value, not a measure of the content of the tramp element in the material, but this was good enough to allow an assessment of each supplier and to get a reasonable prediction of the level of each tramp element in a heat given the combination of scrap in the mix.
Having used linear regression to calculate the percentages, a good measure to evaluate the quality of the results is to calculate the coefficient of determination, R2, which indicates the proportion of the variation in
the percentages of tramp elements in the liquid steel that is predictable from the scrap material loaded in the furnace.
This coefficient of determination varied for the five tramp elements and is shown in Fig. 11
These were considered reasonably good results and were used in two ways.
The first was to evaluate the quality of the suppliers, by providing Ori Martin with a statistical value indicating the percentage of content of each tramp element for each material delivered by each supplier.
The second was to develop an application that tracks the loading operations of the crane for each heat and indicates the predicted level of tramp elements in the liquid steel that would result from the load while it is accumulated for the heat. This allows operators to see if the level of any tramp element risks exceeding a threshold and take corrective actions by choosing appropriate material to load.
The model is retrained automatically in the cloud every week on the data containing all heats in the previous six months, excluding the last 150 heats, which are used to verify if the new model is more accurate than the previous model. If the accuracy of the new model has improved with respect to the previous one, it is deployed automatically on the Tenova EDGE device in the plant, where it is called by the automation software.
VII. Conclusions
We have learned a lot while designing, training, and evaluating these machine learning models, and were often surprised by how quickly these models learned themselves, especially the ones involving image classification. In general, we have dedicated more time to cleaning up the dataset of images and selecting good ones than in trying different models and hyperparameters, in part because looking at thousands of images takes time, but also because we found that the quality of the images mattered, often more than their quantity. We had reasonably good results with a few thousand good quality images in both projects.
There are several advantages to using fewer images. The first one, already mentioned, is being able to see all of them, and thus improve the quality of the data. The second is that training is faster, therefore
it is possible to try more models and more combinations of hyperparameters in any given time. Of course, for some applications many images are necessary.
As we observe the models in production, we evaluate their classifications, and as we find more good images representing the various categories, especially if they were misclassified by the models, we add them to the datasets for future retraining.
We used image classification techniques and did not try object detection, where the goal is to identify the location of each object together with its type, because it would have been a very difficult and error-prone task to locate and label all the different types of materials inside each image, it could only have been done by Ori Martin’s technicians, whose availability was hard to get, and it would have added a lot of complexity without adding much value. It would also have required many more images, with possible degradation of image quality. Our goal was never to locate material, only to give an indication of its type or find out if any of it was present on the Consteel®
For the model using process and sensor data, we have implemented automatic retraining, validating of the model, and automatic deployment. This was possible because we could implement automatic feature engineering on the new data, and we could evaluate the performance of the model automatically. For the image classification models we have not implemented automatic retraining and deployment, mainly because of the manual work of verifying the quality of the images, classifying them, and evaluating the results.
Machine learning projects do not end once the models are deployed. We are still evaluating their performance, gathering more images and data, regularly retraining them, and updating them to adjust them to the everchanging world outside.
Acknowledgment
All these projects were team efforts. I would like to thank the whole Tenova Digital Team, of which I am part, for all the support, the competences, and the curiosity they continuously demonstrate, it is a pleasure to work with them. I would also like to thank my colleagues in Tenova Upstream for providing us with interesting problems to solve and a lot
of help to understand them, and Ori Martin for giving us access to the plant, to the data and for answering all our questions.
A particular thanks to Enrico Malfa, for writing the introduction, and to Marco Corbella, Franco Amadei and Nicolò Giso for reviewing this paper and suggesting improvements.
References
[1] Valentina Colla, Costanzo Pietrosanti, Enrico Malfa, Klaus Peters, “Environment 4.0: How digitalization and machine learning can improve the environmental footprint of the steel production processes,” Matériaux & Techniques 108, 507 (2020)
[2] ESTEP, The Circular Economy Focus Group, “Improve the EAF scrap route for a sustainable value chain in the EU Circular Economy scenario,” Roadmap, June 2021
[3] Aurélien Géron, “Hands-On Machine Learning with Scikit-Learn, Keras, and TensorFlow: Concepts, Tools, and Techniques to Build Intelligent Systems,” O’Reilly, 2019
[4] Francois Chollet, “Deep Learning With Python,” Manning, 2018
[5] Ismael Martino, Valentina Colla, Stefano Bragiola, Stefano Bardella, Uggero De Miranda et al., “Environmental impact evaluation and effective management of resources in the EAF steelmaking,” EIRES, RFSR-CT-2013-00030, July 2013 - Dec 2016
[6] Raghav Bali, “Supercharge your image search with transfer learning,”
Towards Data Science
[7] CONOPT SCRAP, Control and optimisation of scrap charging strategies and melting operations to increase steel recycling ratio, RFCS, 2005-2008.
[8] FLEXCHARGE, Cost and energy effective management of EAF with flexible charge material mix, RFCS, 2007-2010.
[9] AdaptEAF, Adaptive on-line control of the EAF based on innovative sensors and comprehensive models for improved yield and energy efficiency, RFCS, 2014-2017.
[10] SUPERCHARGEEAF, Supervision of Charge Material Properties in EAF steelmaking Utilising Advanced Statistical Methods, RFCS, 2017-2020.
[11] OptiScrapManage, Optimization of scrap charge management and related process adaptation for EAF performances improvement and cost reduction, RFCS, 2014-2017.
[12] ORI Martin, “Metal scrap classification and
Fig 10. Confusion matrix for identification of bulky material
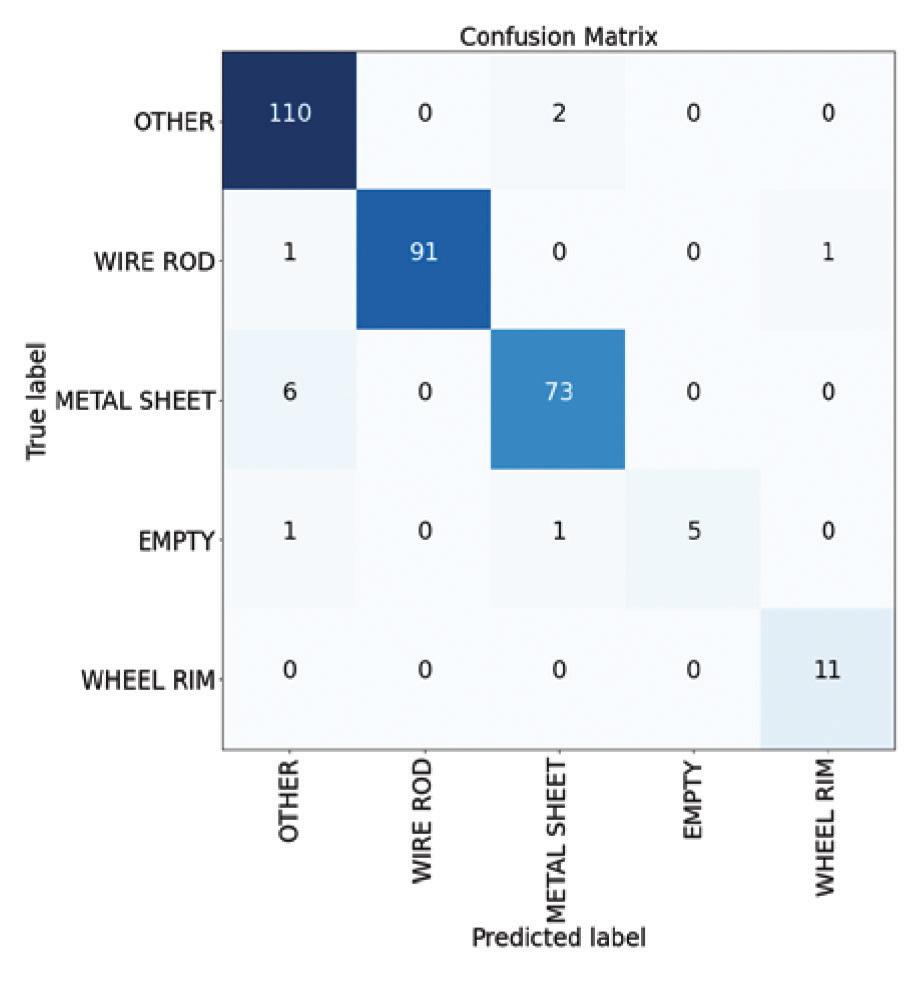
MACHINE LEARNING
Copper Nickel Arsenic Tin Molybdenum R2 81% 76% 75% 75% 69%
Fig 11. Coefficient of determination for the five tramp elements
tracking in ORI Martin,” in Green steel by EAF route: a sustainable value chain in the EU Circular Economy scenario, Bergamo, Italy, 2019.
[13] Arcelor Mittal, “Novel tools for scrap yard management in steelmaking facilities,” in Green steel by EAF route: a sustainable value chain in the EU Circular Economy scenario, Bergamo, Italy, 2019.
[14] BFI, “Intelligent scrap management and adapted EAF process control,” in Green steel by EAF route: a sustainable value chain in the EU Circular Economy scenario, Bergamo, Italy, 2019.
[15] ORI Martin & STIIMA CNR, “0 Lighthouse Plant ‘Acciaio 4.0’: an example of integration evnironmental aspect in the production process,” in Resi4Future - Residue valorization in iron and steel
industry - sustainable solutions for a cleaner and more competitive future Europe, 2020.
[16] EUROFER, “LOW CARBON ROADMAPPathways to a CO2neutral European steel industry
Further reading...
• Teresa Annunziata Branca, Barbara Fornai, Valentina Colla, Maria Maddalena Murri, Eliana Streppa, Antonius Johannes Schröder, “The Challenge of Digitalization in the Steel Sector”, Metals 2020.
• Bożena Gajdzik,Radosław Wolniak, “Transitioning of Steel Producers to the Steelworks 4.0, Literature Review with Case Studies”, Energies 2021.
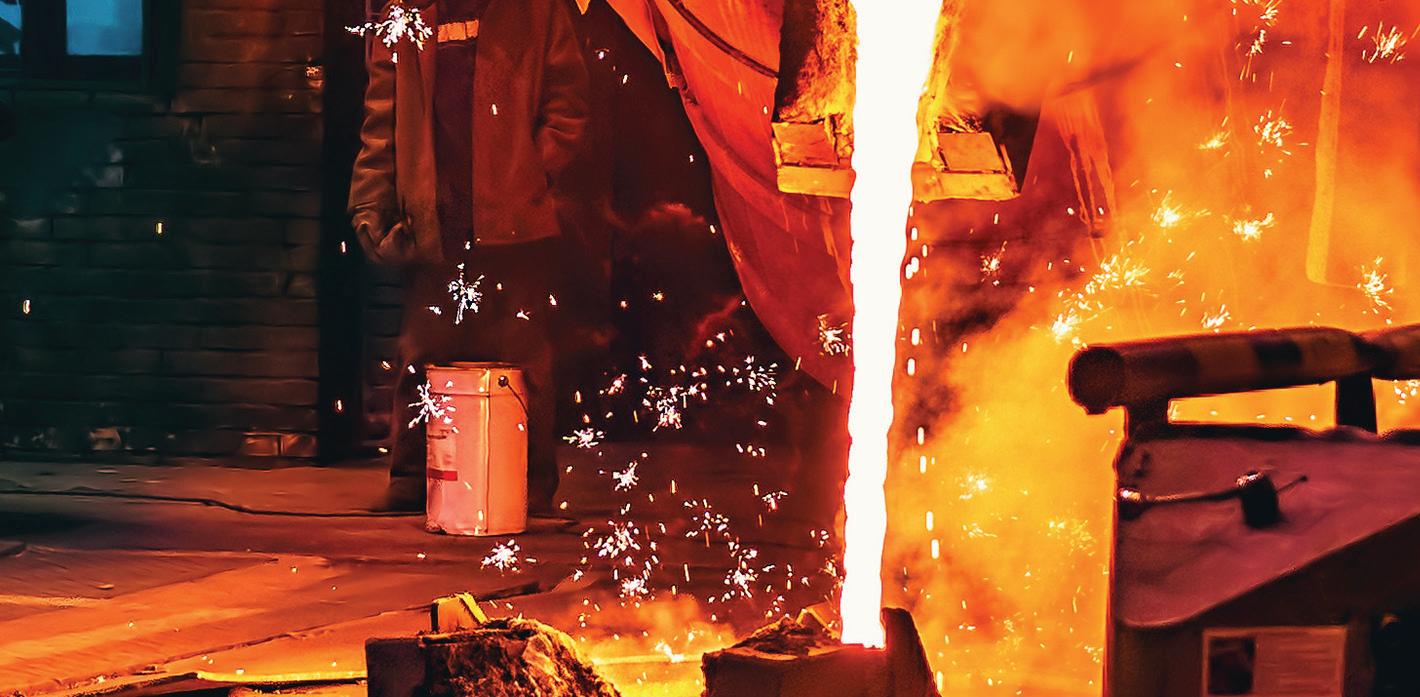
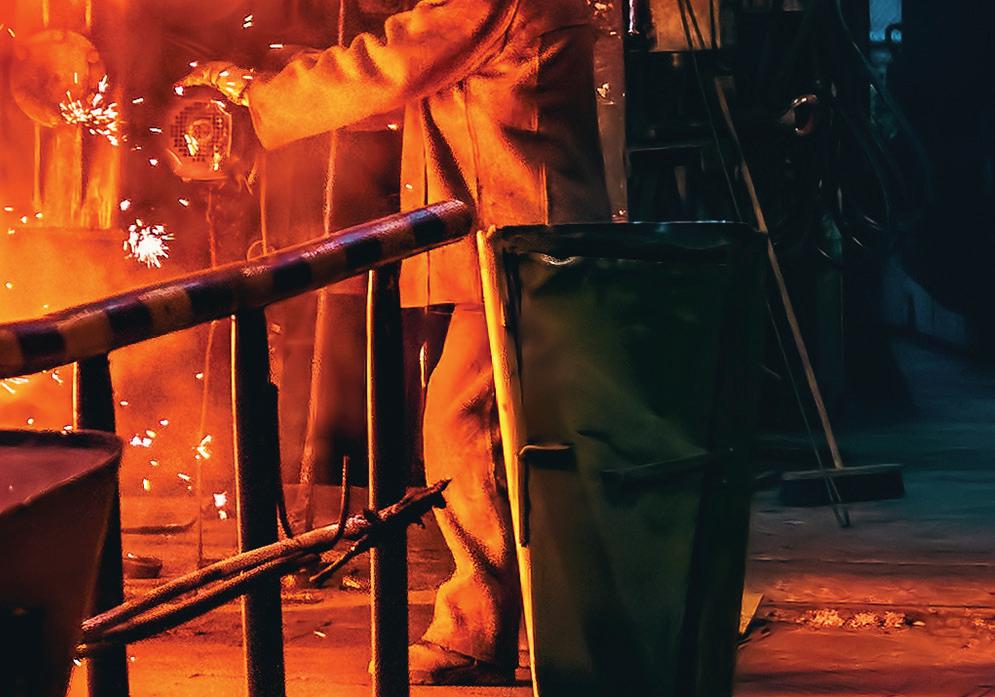
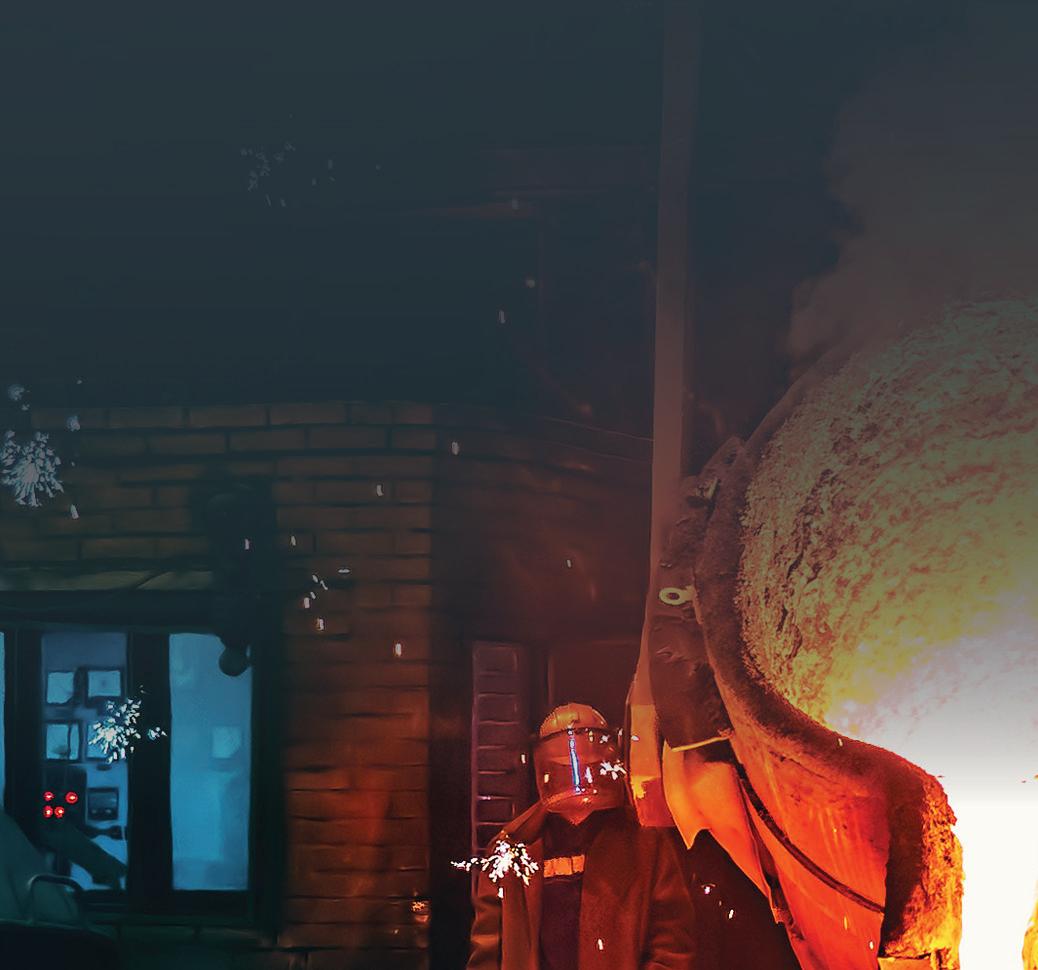
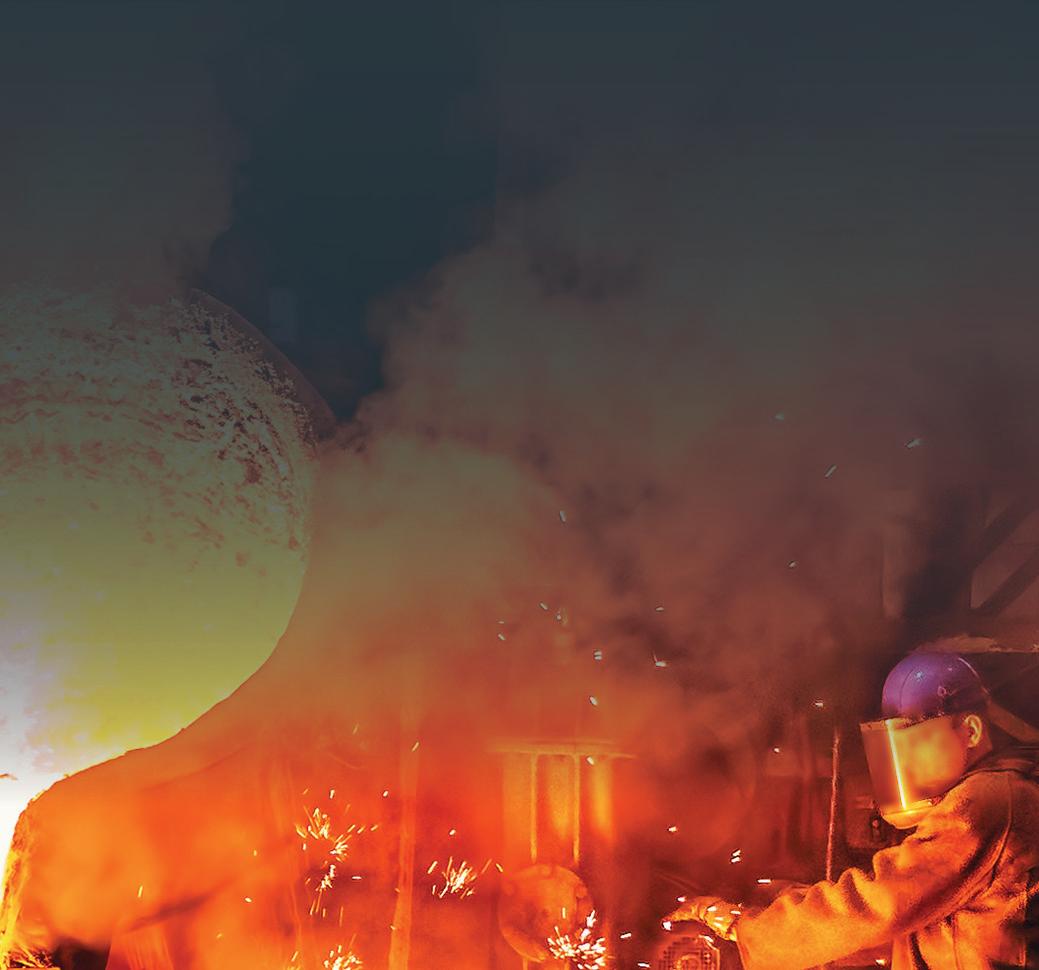
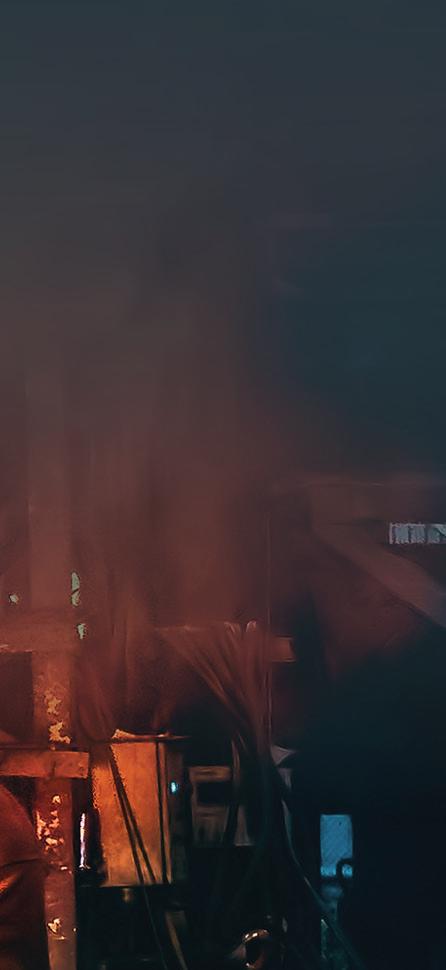
Neural networks for visual tracking
Neural networks for visual tracking
In the steel industry it’s almost impossible to apply adhesive labels, RFID, and other technologies, commonly used in other industries, to track the products. The current practice is to rely on sensors and logic to suppose the position of the products during the process. Machine Vision and Neural Network Technology can finally solve the common issues of the current tracking systems, the visual recognition of dimension, colour, shape, and other factors during the movements of the product dramatically increase the reliability and re duce potential mismatch and claims, benefitting quality and productivity.
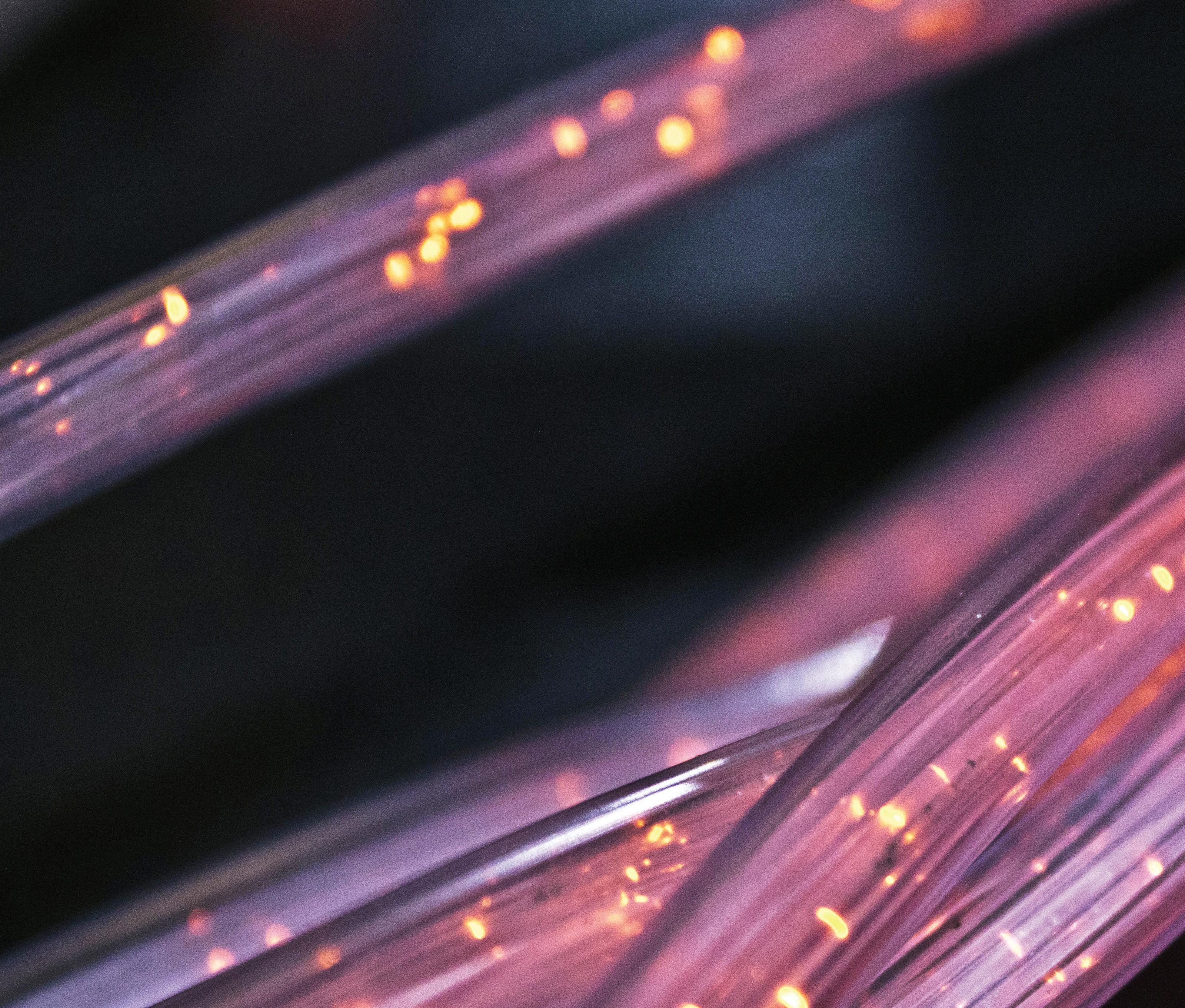
TRACKING SYSTEMS
is read by the PLC and communicated to the tracking system and L2 for the creation, inside of the production database, of a billet. Normally at this point other information, such as grade, work order, and batch numbers, are associated with the billet. When the billet is moved to the end of the run-out table, a sensor (which can be an hot metal detector, a laser, a photocell or just using the billet to close an electric circuit) moves the logical position of the billet in the tracking system to update the information.
This approach works properly only when all the information is precise and processed correctly, any kind of fault on a sensor, such as a manual movement from the operator, or an abnormal working condition, can affect the reliability of the information.
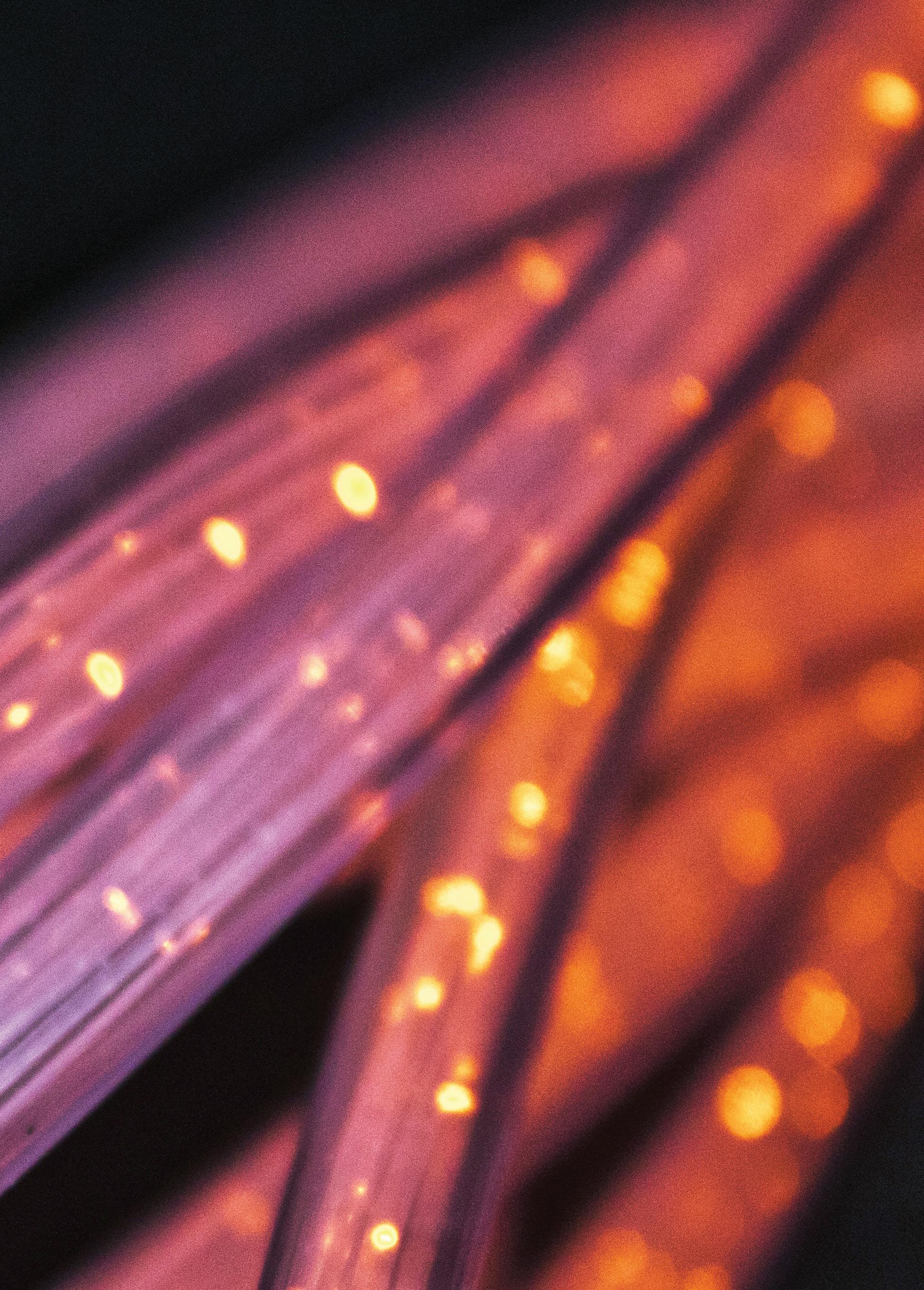
It’s easy to understand how tracking based on sensors could be problematic everywhere inside the mill, but this is especially apparent on the casting machine where any issue has to be solved quickly by the operator to prevent interruptions that have an effect on the amount of billets produced and consequent misalignment in the tracking. This paper illustrates how different approaches, that go from the already mentioned sensors to billet tagging robots, can greatly improve the tracking reliability, especially when a combination between vision systems and artificial intelligence algorithms is used (Fig.1).
In recent years, steel mills have evolved to be more customer-centric, with a wide range of steel grades produced and a high customization in terms of length and dimensions of the material. This results in a more complex management of the production that must keep in account all these variations between batches, that are often close both from a time-centric and geographical point of view of the process. Another matter that is of crucial importance is the reduction to the least amount possible of scrap and waste products that are usually a natural consequence of the process but can often be caused by human and/or machine mistakes during the traceability of the product that, at a given point, cannot be identified through the production steps and, therefore, have to be discarded or re-processed with consequent loss in production efficiency.
To manage such difficulties, plants often rely on several technologies to monitor and
track the material, such as:
� Weight measurement on the ladle support (car or turret)
� Slag detection during tapping
� Weight measurement on the tundish
� L2 to predict the quantity of mixed steel in the tundish
� Quality tracking on the casted products
All of this information is strictly related to the necessity to guarantee an error-proof tracking system so that any ‘catastrophic’ mistakes can be avoided. The current technologies used to track billets in the casting machines are related to the automation (signals from physical sensors and PLC logic that translates them into interpretable information).
One example is billet ID creation. In most cases, a signal from the torch cut or the shear,
Previous state of technology Level 1 sensors and manual tracking
The most obvious and obsolete way of tracking material, in any industry but especially in steel, that receives automation updates, the latest due to the critical environment, is by having a human operator placing information and moving it around the production line as the material (let it be billets, plates, blooms, coils, etc.) proceeds through the different phases. This is usually a direct cause of the material handling technique that is often almost fully manual, where operators are in charge of activating the moving mechanisms such rollers, cooling beds or cranes. This method is the most unreliable and least efficient for obvious reasons and is obsolete. The first evolution involves using it along with automatic sensing devices that can detect the material presence. The signals of these sensors can then be elaborated
by a PLC (level 1 automation) and used in a mapping logic to understand whenever a plate/billet has moved along the line. Some sensors/cameras needed for process monitoring are already placed close to the hot material [1] but are of no use for tracking in large facilities.
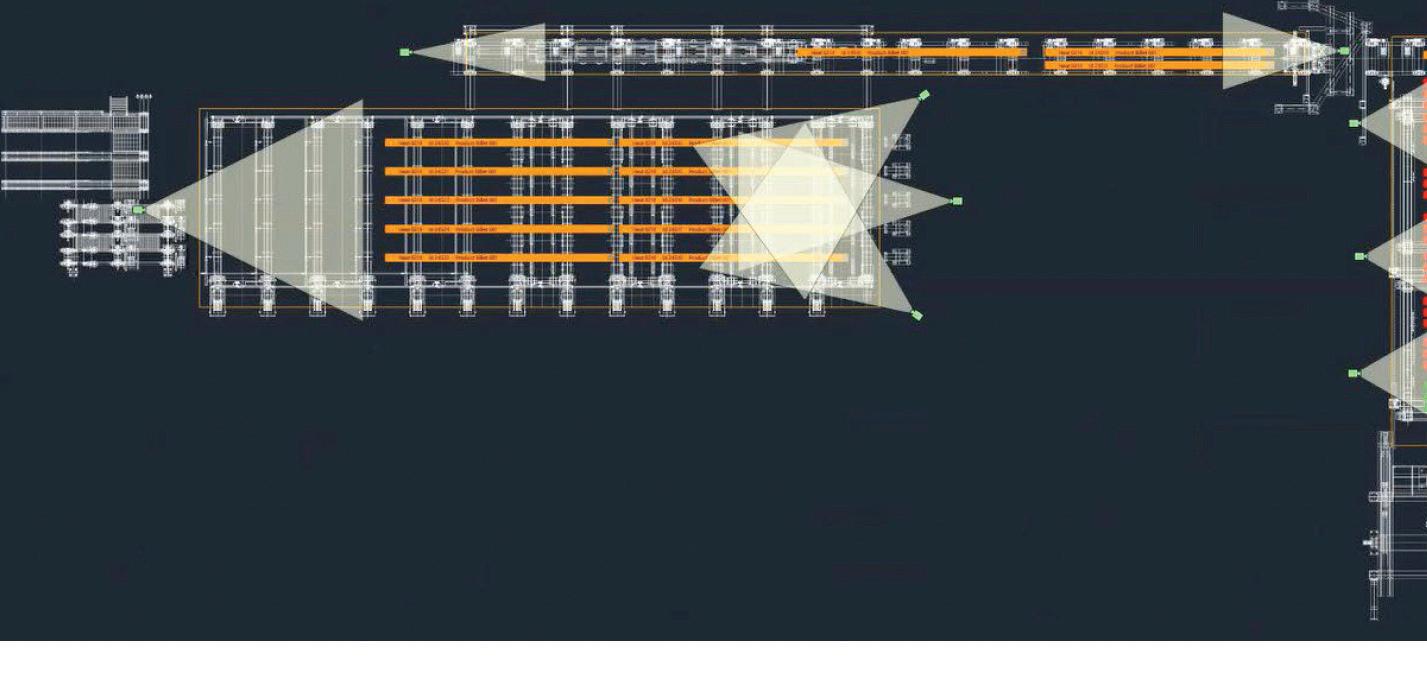
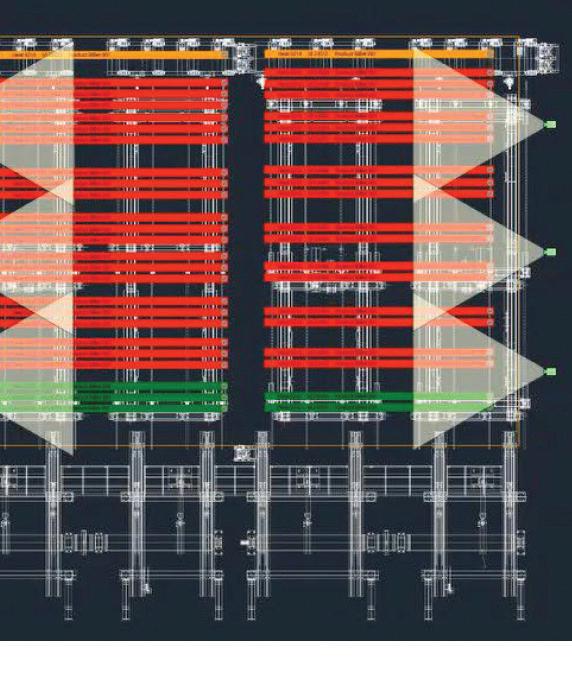
The approach of using material detecting sensors is already more commonly used but has several downsides:
� Sensor placing: often positioned close to the harsh environment around the hot metal that can cause malfunctioning
� Number: smaller/shorter products require sensors to be placed very close to one another to avoid ‘shadow zones’, with a consequent increase of devices needed in case of extended plants
� Maintenance: being close to the hot material means that maintenance can be done only during a production stop, both for ordinary and extraordinary maintenance like
calibration or substitution
� Integration: being connected directly to level 1 (PLC) means that tracking data has to go through several layers before reaching the production and supervision layer.
� Unusable areas: in some large transfer areas or cooling beds (example Fig.1) sensors cannot be used at all, with a high risk of information loss
Camera tracking

The sensors for presence detection are still a valid technology and keep having a use case in certain conditions, especially if their use is shared between tracking and automation (for automatic control of certain parts of the moving mechanisms for example). One evolution involves the implementation of cameras or 3D scanners for material tracing both as an alternative to the sensors, or in those places where sensors cannot be installed in the first place (like cooling beds).
TRACKING SYSTEMS
This first approach can solve several of the above-described problems of sensor tracking. Thanks to a proper selection of the lenses, cameras can be mounted further from the hot material, allowing them to be less sensitive to the harsh environment around the hot material. Remote placement means that maintenance on the device can be done during plant production since the cameras can be mounted in safe areas. The larger view area of the camera also means that one device can cover an area where many sensors would be needed.
(Fig. 2 shows the placement of cameras in a typical hot billet production line).
While the majority of the problems connected to the only usage of the sensors are solved with this system, it still has some flaws, mainly due to NiR cameras being able to distinguish only glowing objects (Fig.3). This translates first of all in the impossibility of distinguishing cold material, which is typically
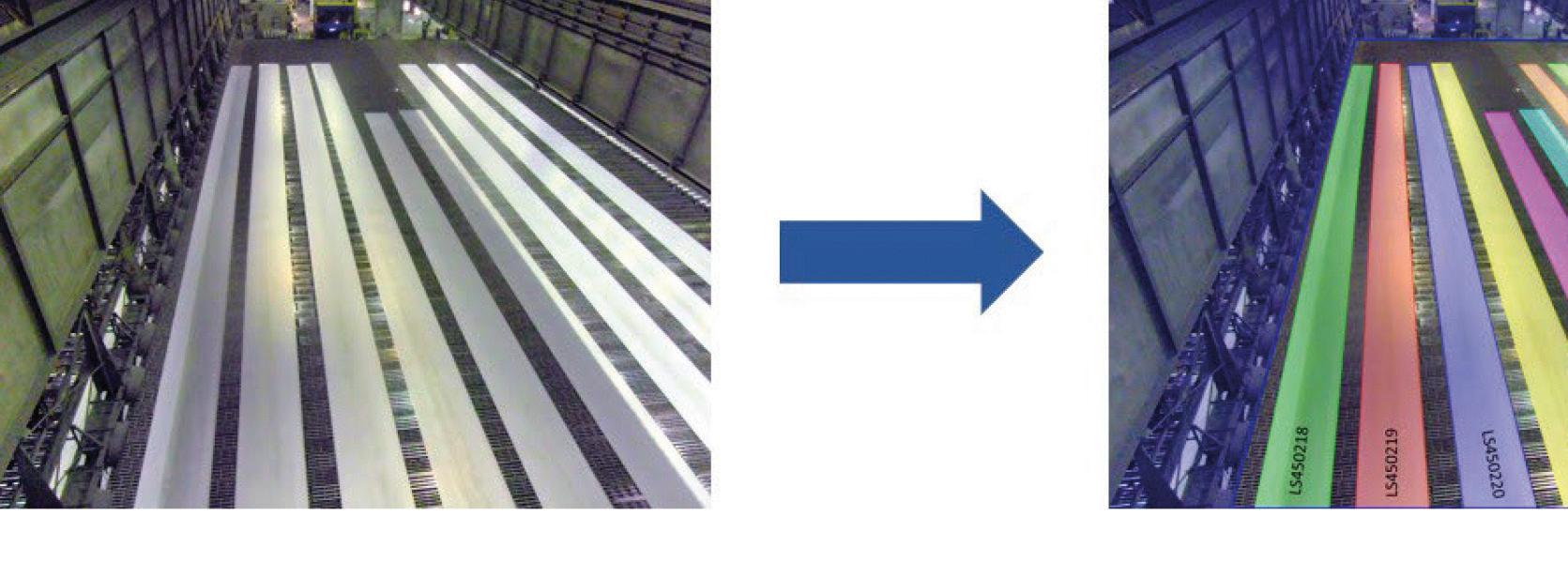
TRACKING SYSTEMS
a grey colour on a grey background. As a side defect, the image is interpretable only by the machine algorithm and is of no use for human analysis due to the high contrast (Fig.4). Another possible problem of 2D cameras can be the acquired precision which might not be optimal for certain products.
Artificial intelligence integration in camera tracking
The evolution of camera implementation helps enhance the acquired information by
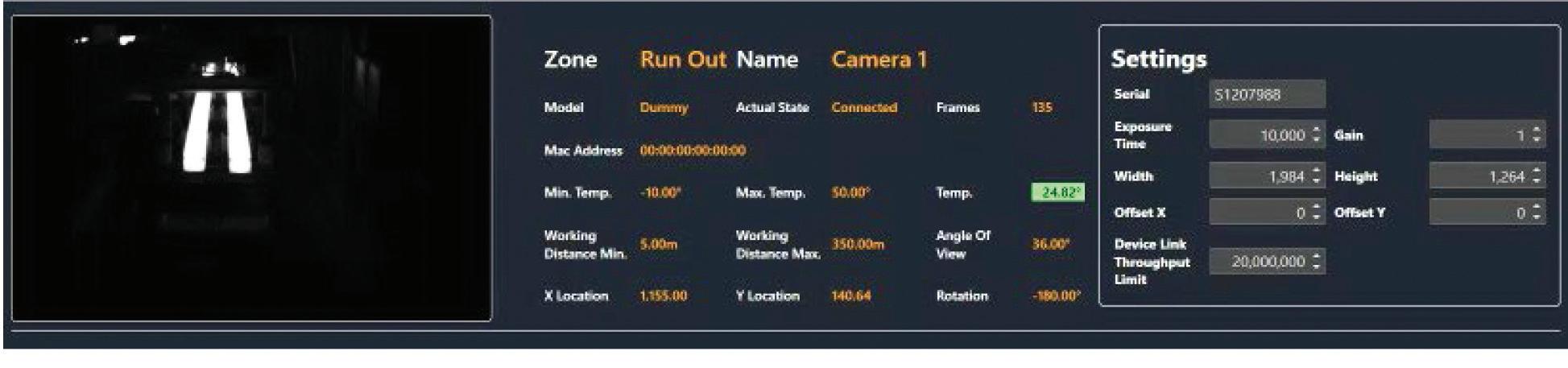
processing it with an artificial intelligence algorithm.
Artificial Intelligence is largely used in several fields and is a consolidated technology in production management applications such as supply chain management.
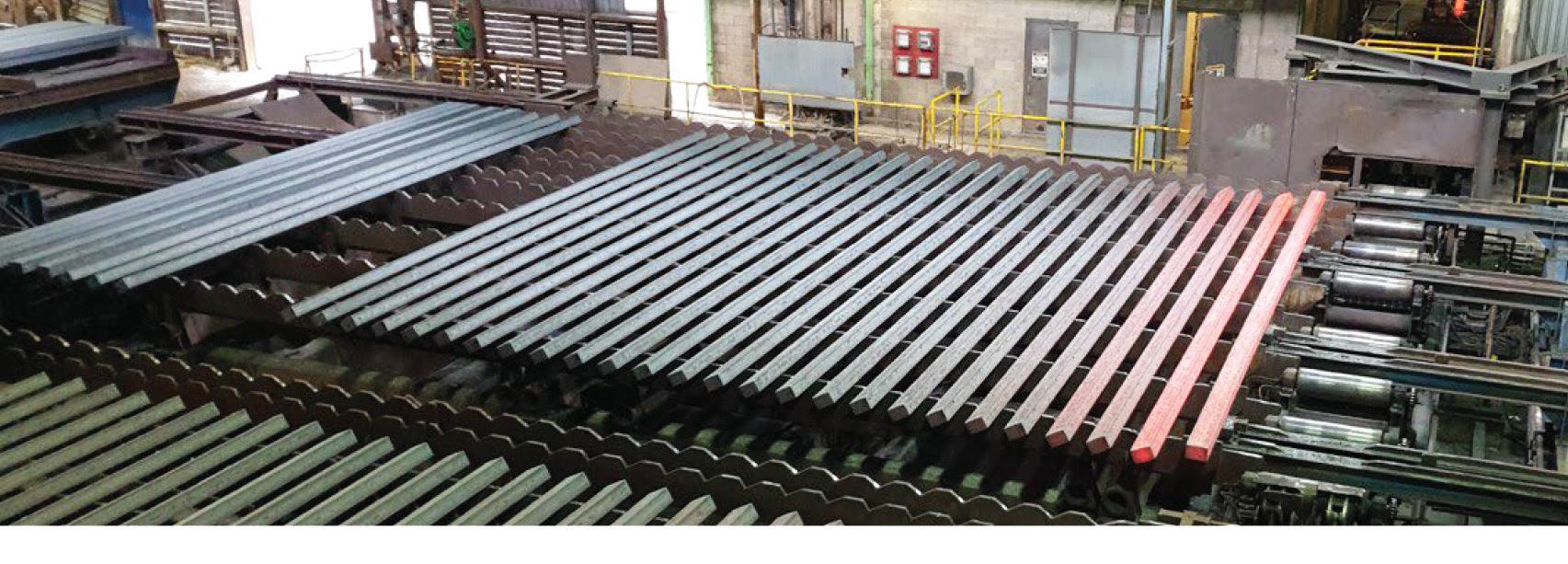
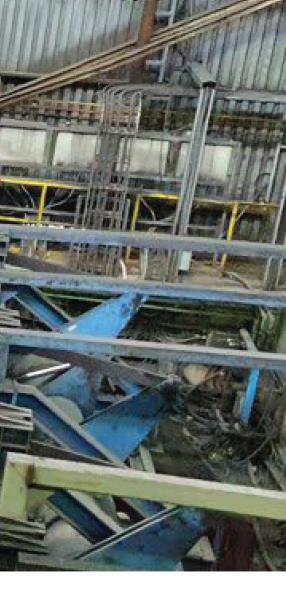
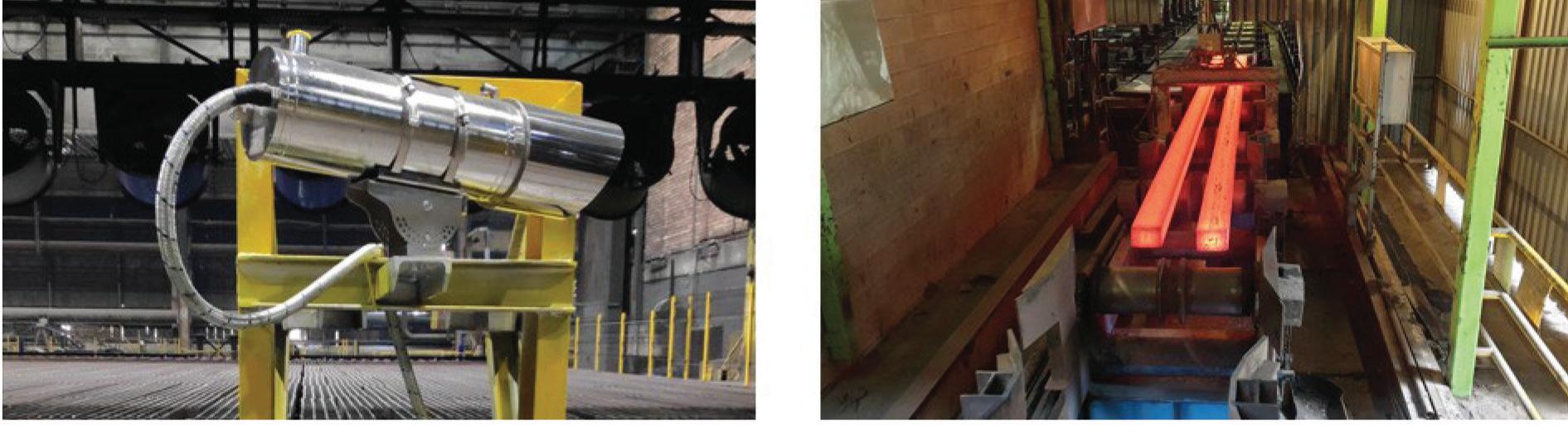
This approach was necessary for applications where the material is not glowing and NiR cameras are not enough anymore, for example after the material has cooled enough to be undetectable from the
background that has a similar colour (Fig.5).
The first step consists in creating a sufficiently large dataset for what is a material to be recognized and what is not. This procedure consists in acquiring about 1,000 pictures of billets in different conditions (temperature, length, light conditions and so on). After that the pictures are rectified and properly pre-processed before creating the dataset for the Deep Learning algorithm.
The dataset is then used by the software that analyzes with AI new acquisitions
Sustainable Industrial Manufacturing (SIM) is a global series of regional exhibitions and conferences supporting and facilitating the transition towards cleaner manufacturing around the world.
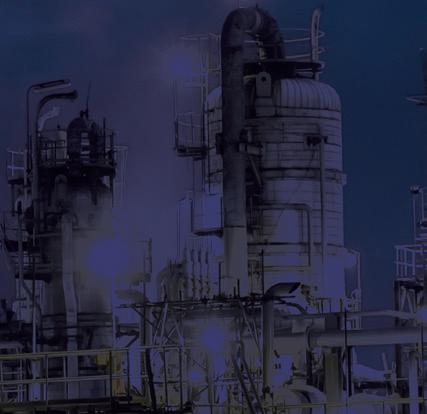
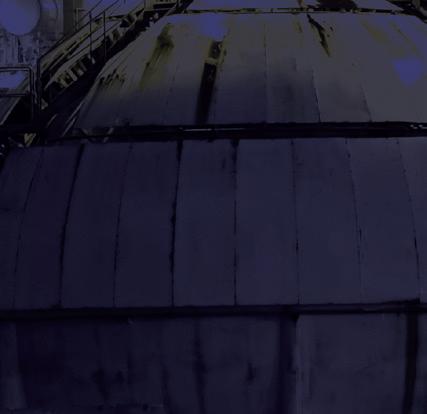
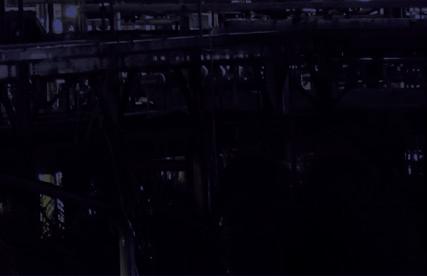
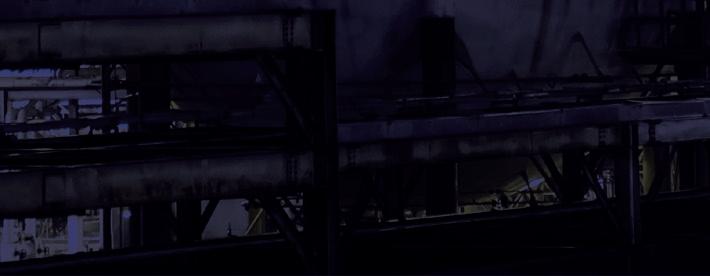
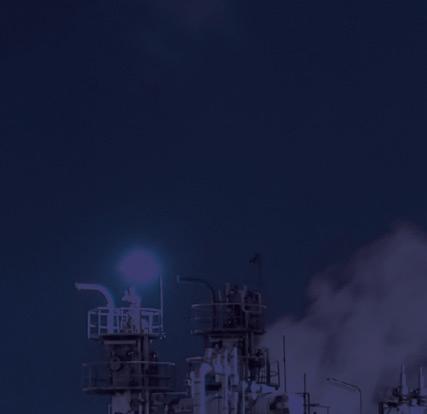
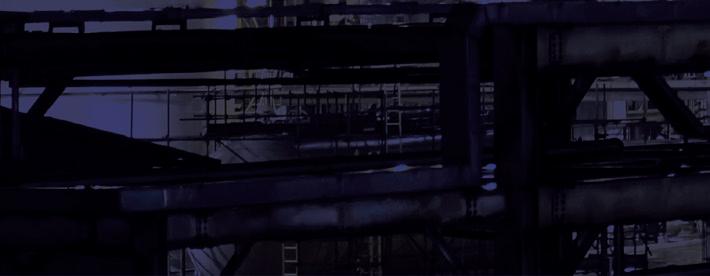
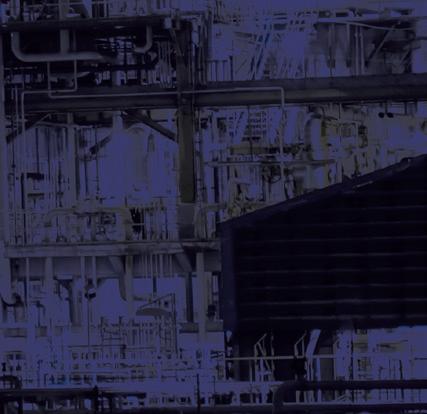
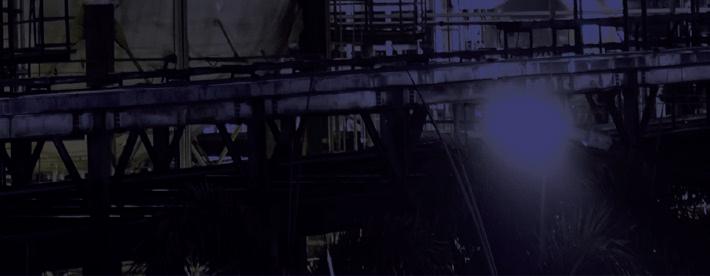
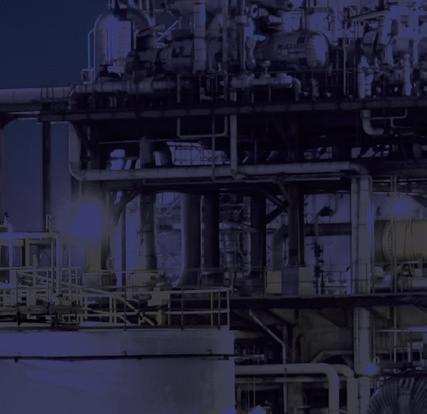
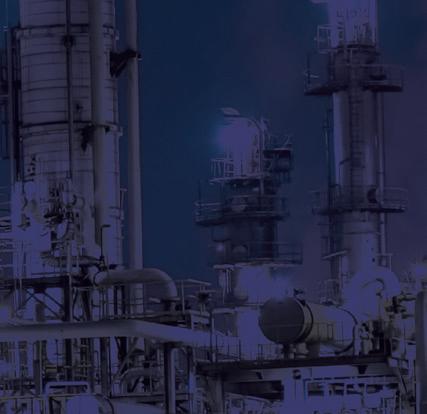
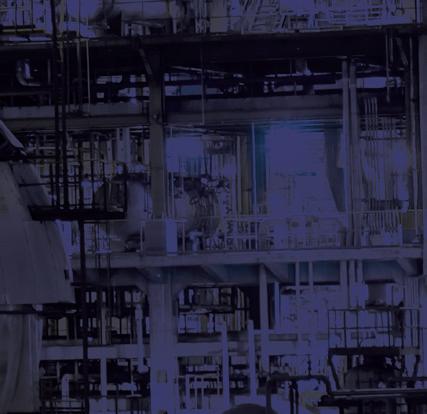
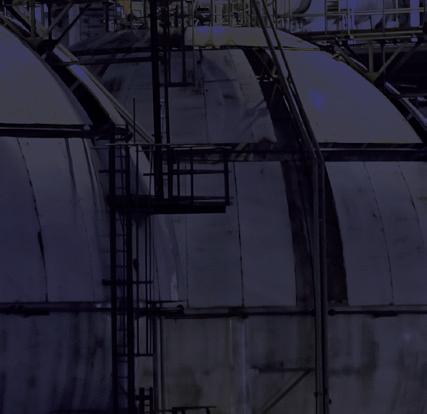
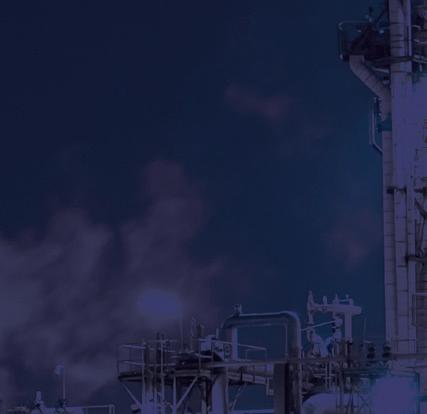
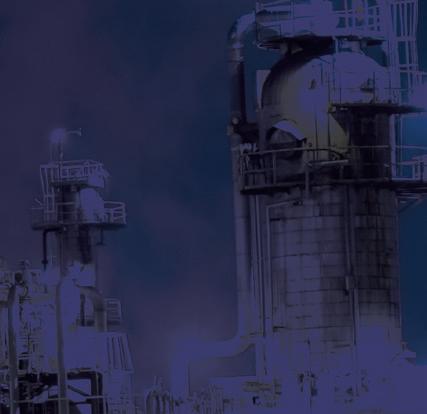
President Biden’s goal of a carbon-neutral economy by 2050 is dependent on the decarbonization of heavy industry. The 2021 Build Back Better Act, provides $4 billion for the deployment of technologies that can accelerate the emission reductions of industrial facilities.
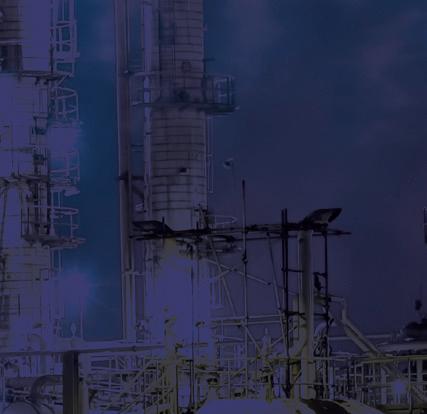
To support and facilitate the transition towards cleaner manufacturing in the USA, Sustainable Industrial Manufacturing will be staged in Cleveland, Ohio in December 2022.
Hosting leaders from industry, innovation, science, government and investment, SIM USA will bring together those responsible for driving sustainability across hard-toabate sectors.
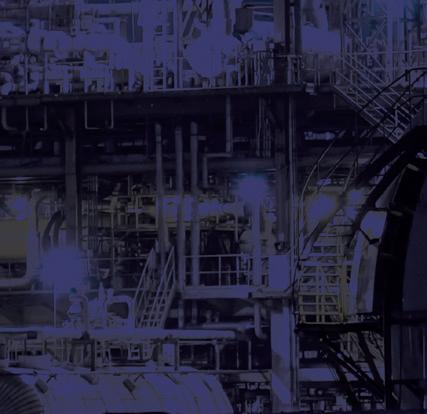
TRACKING SYSTEMS
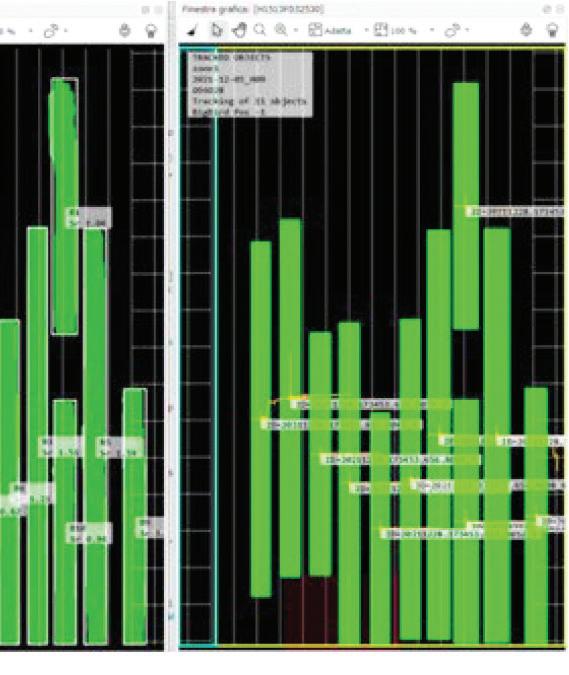
captured by the cameras and identifies the material to be traced. The software runs on a server connected to all the cameras and can be fully independent by the sensors being mounted on the plant field. The usage of such a technique also creates the possibility to merge views of different cameras, allowing for larger areas to be monitored. (Fig 6)
Each camera generates an image that is rectified, and the path recognition algorithm creates a shape.
The shapes from different cameras are merged into a single object. The algorithm then generates a persistent object, and this way if part of the billet is not visible (under the bridge for example), the system will still recognize it.
Fig 7. AI algorithm output and tracking
Furthermore, the algorithm classifies the object based on shape and dimension considering a real billet and calculating the position (from head and tail). The final result of the machine vision algorithm is the tracked object output (Fig. 7).
Conclusions
The evolution of hot billet tracing techniques culminates with the current state-of-the-art technique that involves the use of colour HDR cameras strategically positioned through the production line of a rolling mill. Thanks to an additional trained AI algorithm, the objects can be monitored even in difficult conditions such as cold billets or different light conditions.
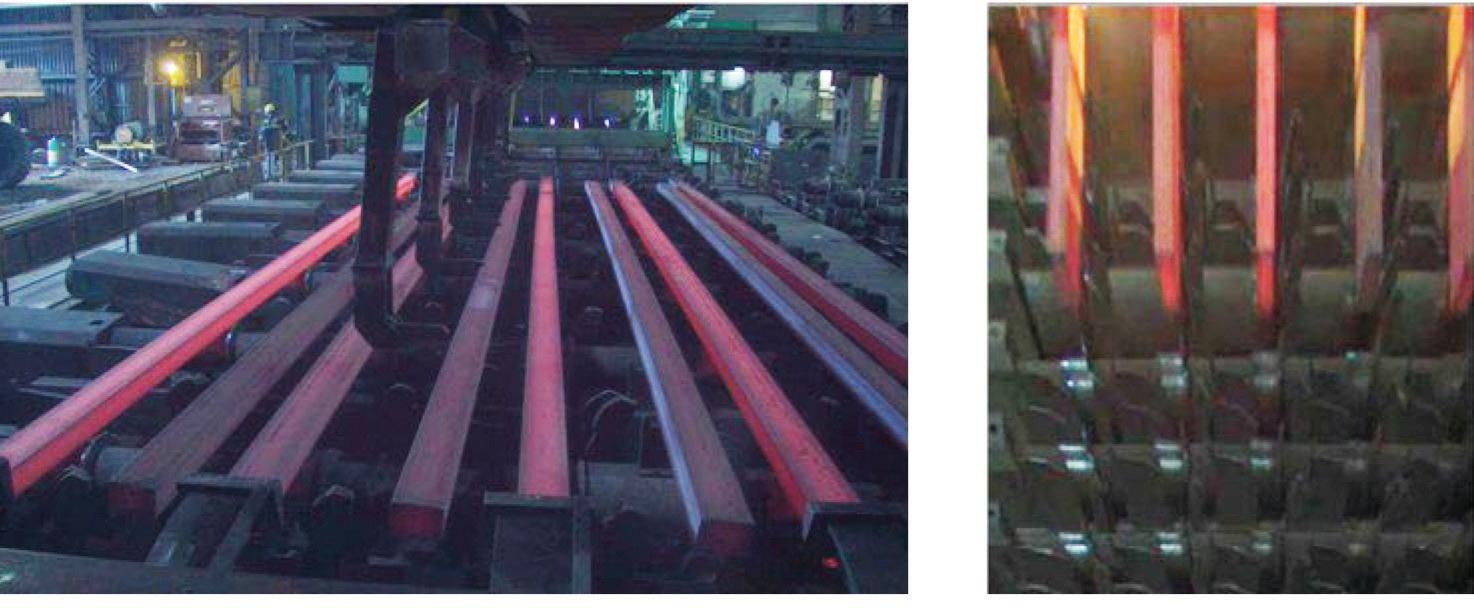
The cameras can be placed further
from the danger area, keeping them more protected than presence sensors, allowing for maintenance and adjustments with active production. Due to the high technology level of the identification software, it can be fully integrated directly with L2 and production monitoring applications while being independent from L1 and PLC software. The AI also allows complex image processing with the benefit of using several cameras that frame a large area that is not traceable with normal sensors.
Compared to traditional NiR 2D cameras, HDR colour cameras also act as perfect surveillance cameras, allowing the production to be constantly monitored with play back functions that allow process investigations. �
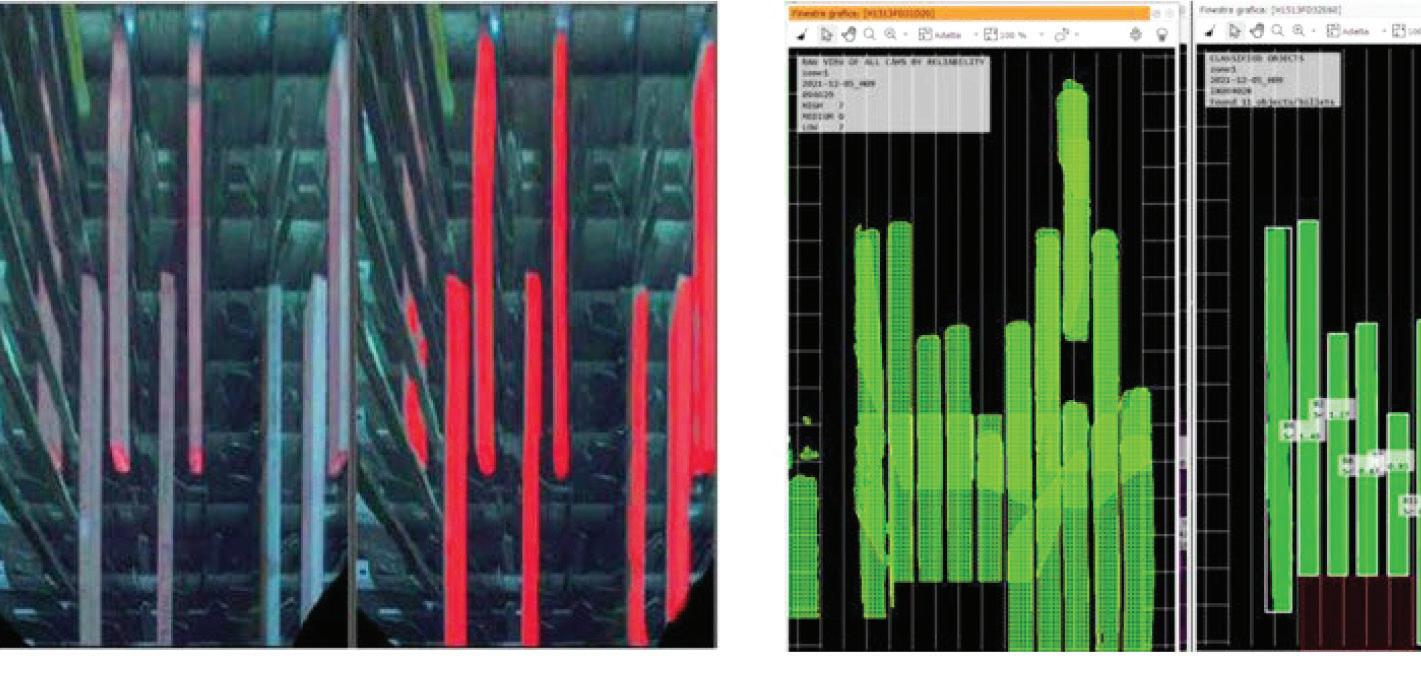
Since 1866
CONNECTING THE INTERNATIONAL STEEL INDUSTRY
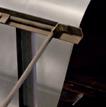

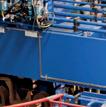
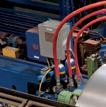
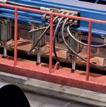
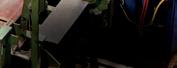
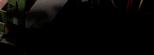
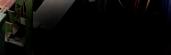
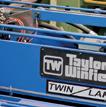
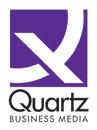




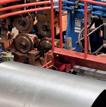


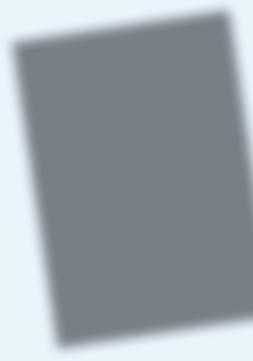


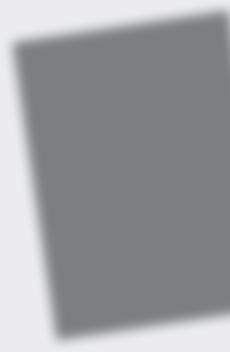
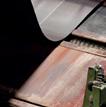

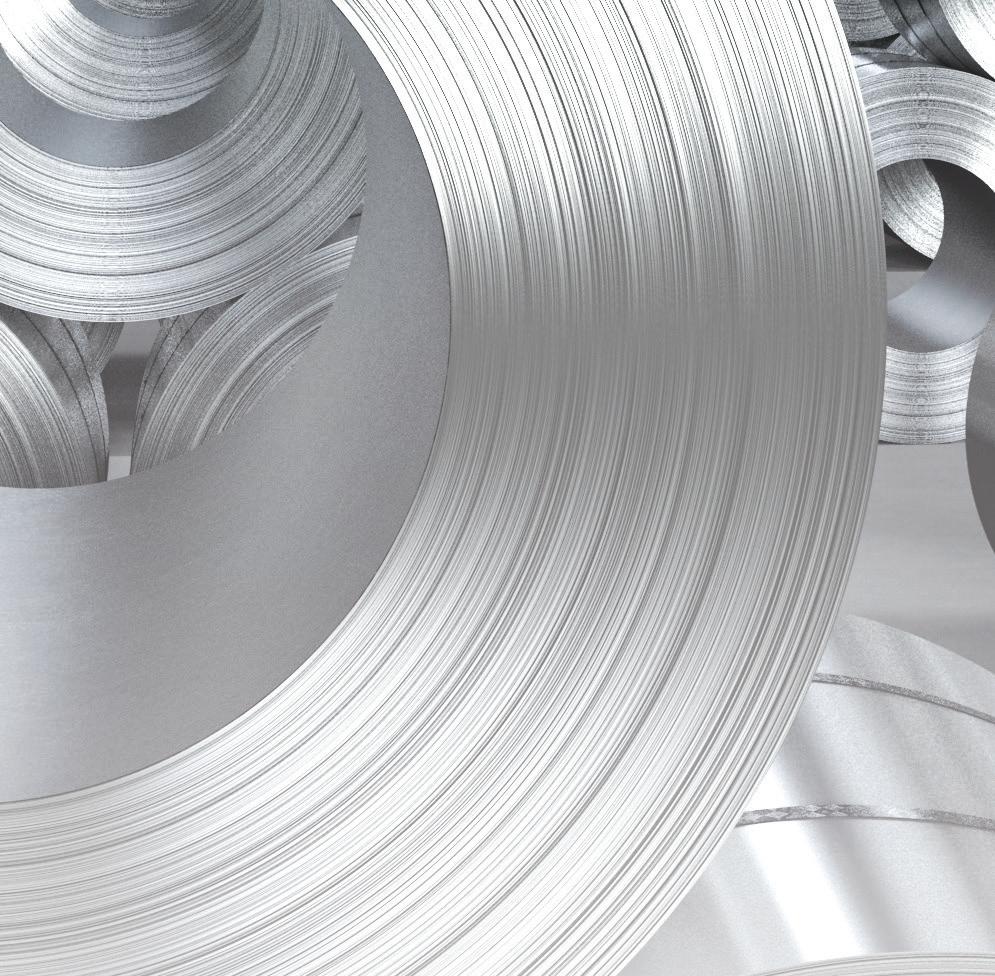
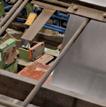
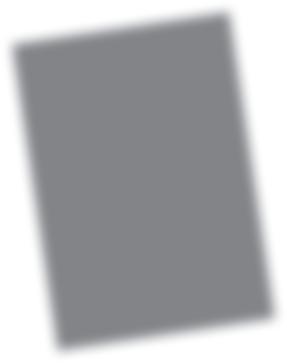

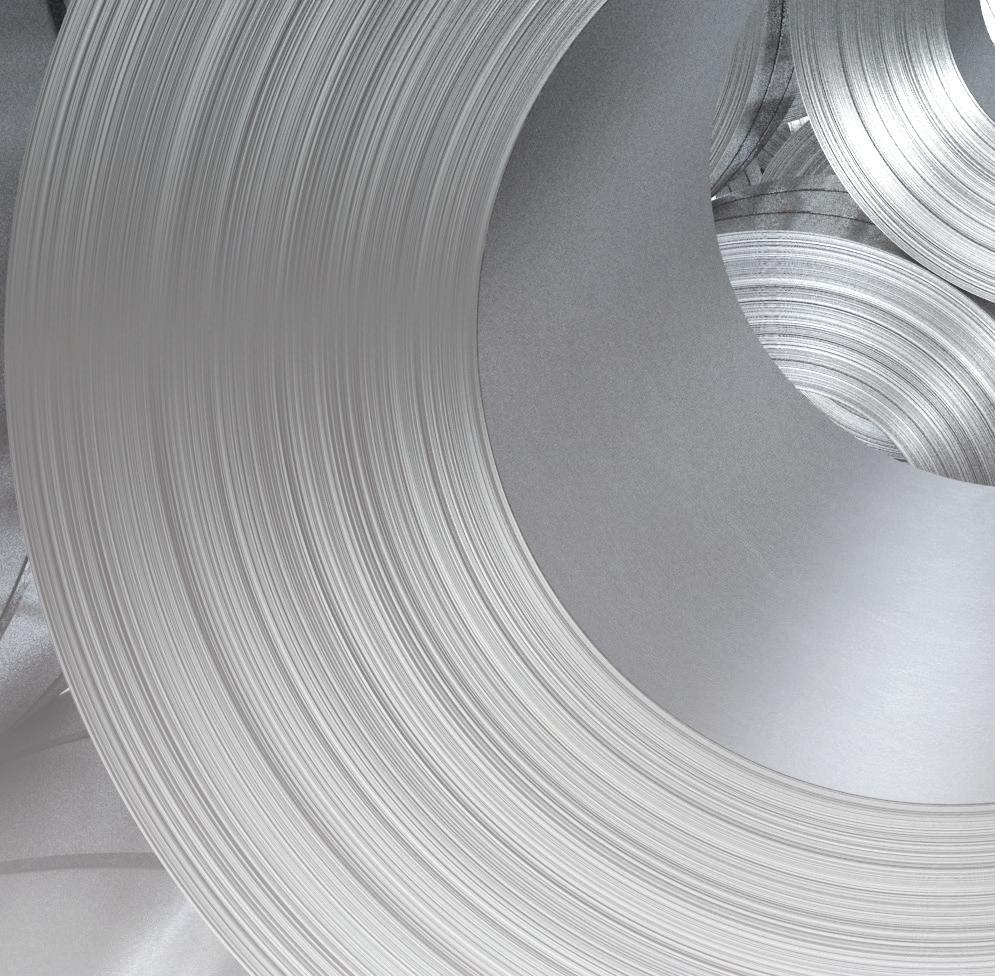
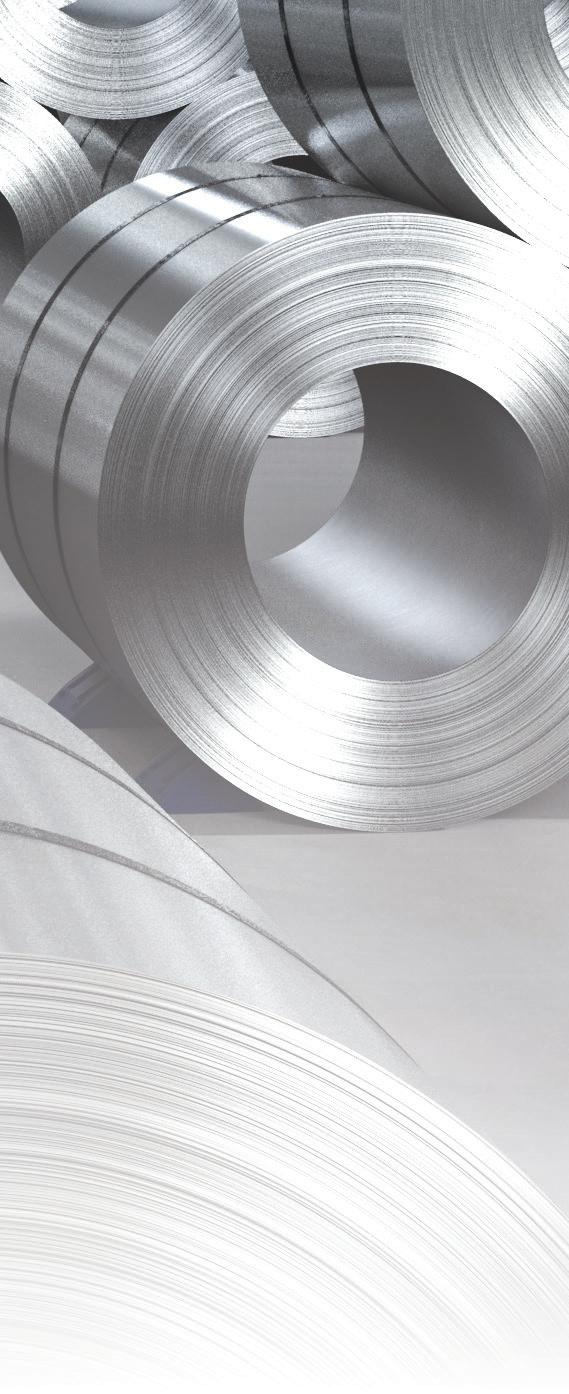
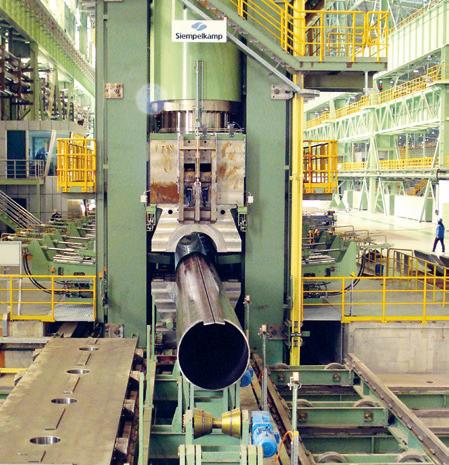
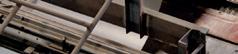
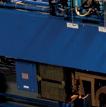
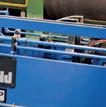
MAGAZINE DIRECTORY
Steel Times International is the key publication for the steel market, reporting on iron and steel making issues from all corners of the globe.
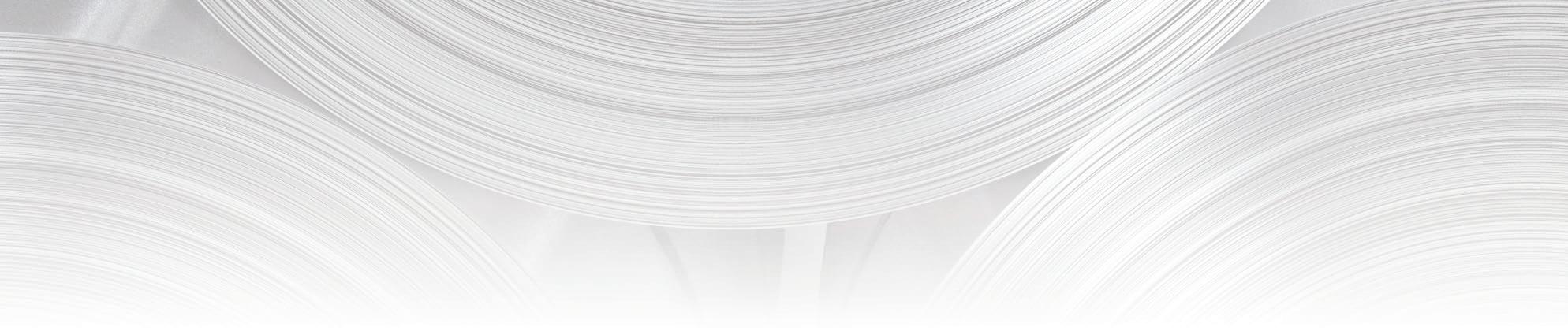
The Steel Times International Directory is the essential guide to steel manufacturers, producers, suppliers of plant equipment and services to the steel industry. Order your copy priced from £95 or FREE for paid subscribers
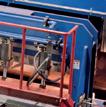
WEB WEEKLY E-NEWSLETTER
Packed with information on the steel industry and continually updated with news for steel professionals, steeltimesint.com also features special articles and interviews with leading industry figures.
A round-up of the top news stories is also sent to more than 8,500 industry professionals each week. You can register online to receive the weekly newsletter and keep up-to-date with the latest news from across the steel manufacturing industry. www.steeltimesint.com/e-newsletter
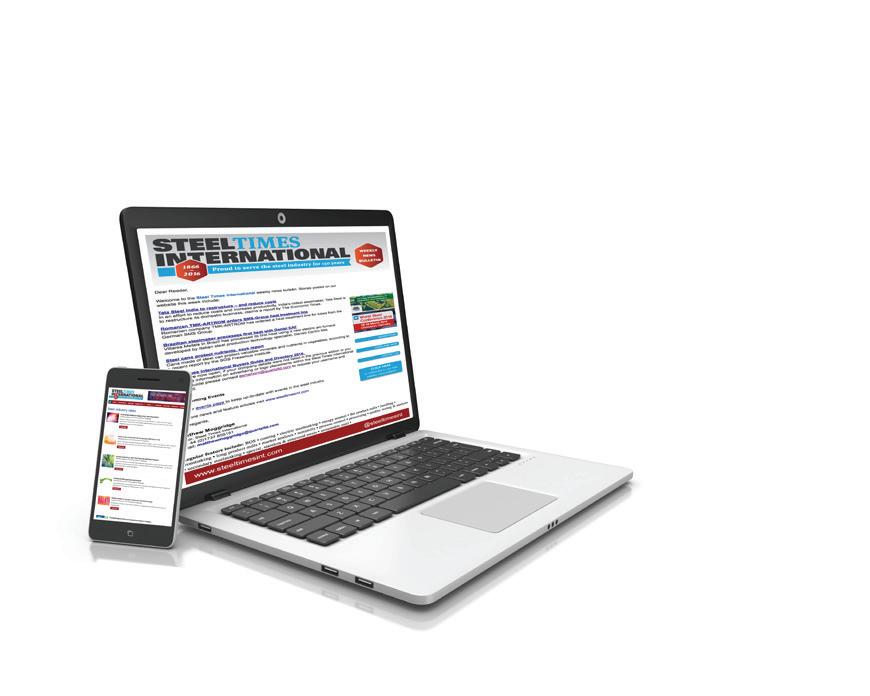