ECONOMIC PERSEPECTIVES
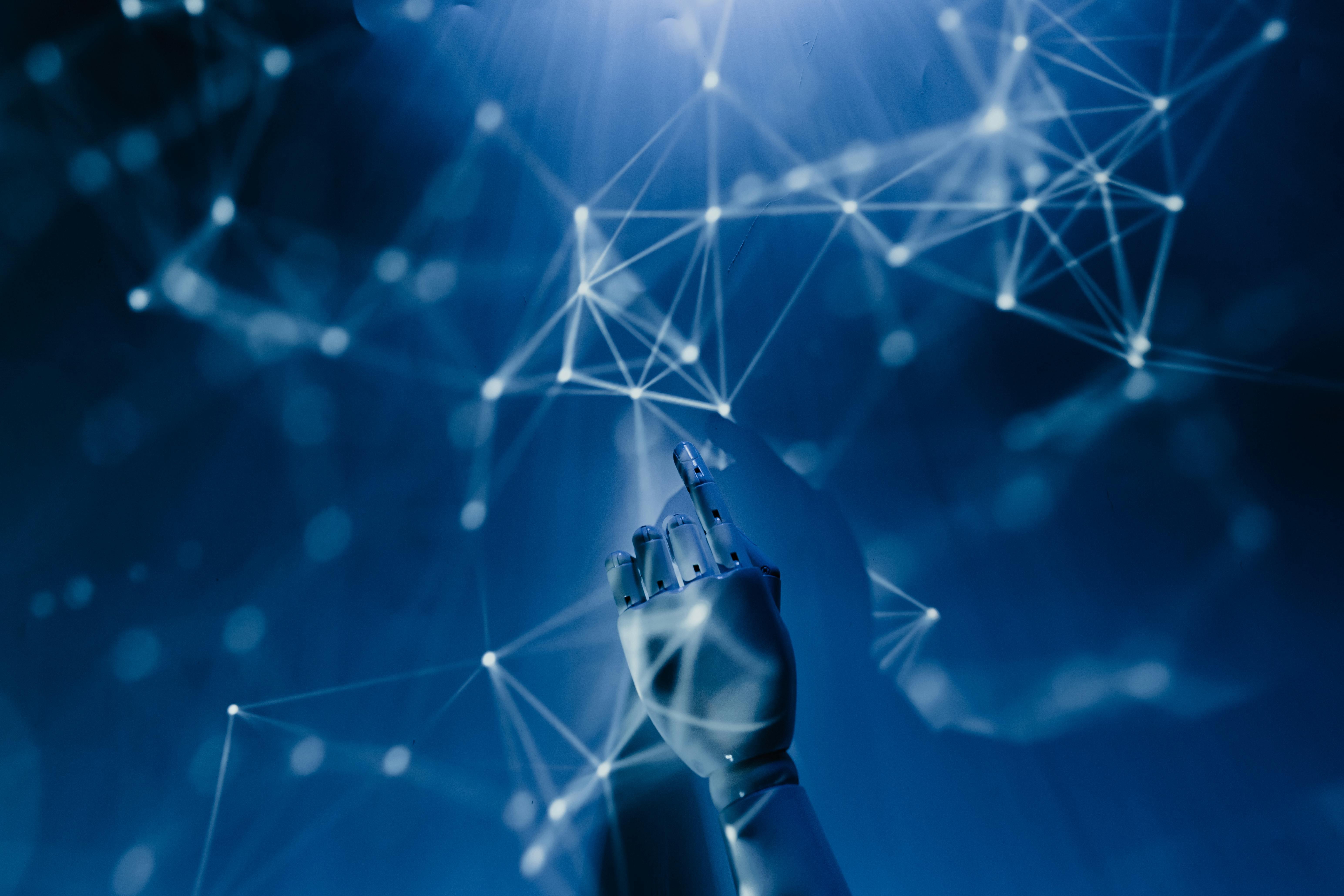
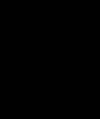
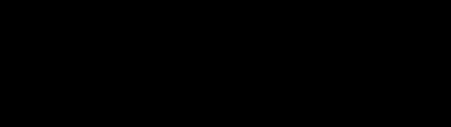
Artificial Intelligence: Friend or Foe?
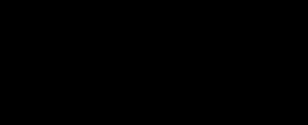
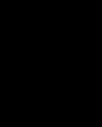
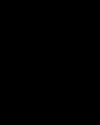
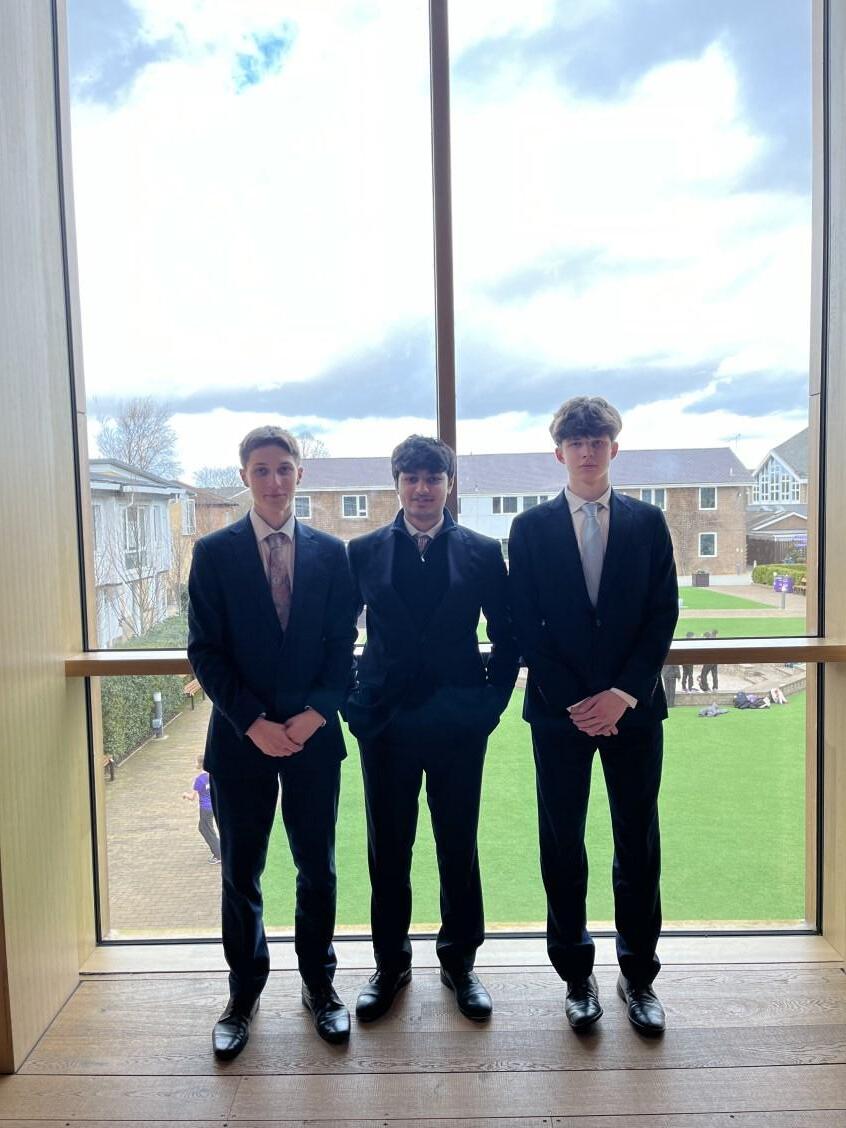
Artificial Intelligence: Friend or Foe?
EconomicPersepectivesis the new Perse Economics magazine, dedicated to students, run by students. Published termly, Economic Persepectivesseeks to explore the complex world of Economics on a theme-by-theme basis.
For the inaugural edition, there was no theme more relevant to 2024 than Artificial Intelligence. As AI becomes more prominent in everyday life, it is essential to assess its economic implications.
From AI’s impact in financial markets to its obstructive effects on the labour market, each article delves into the areas of Economics most affected by this rapidly developing tool. Every writer provides a fresh perspective on their chosen economic matter through indepth analysis and ideas on AI’s role in the future.
This edition includes eleven articles, one of which is written by OpenAI’s infamous chatbot ChatGPT, to suit the theme of Artificial Intelligence.
Thank you for joining us, and we hope you enjoy reading the first edition of EconomicPersepectives.
Before we explore the ins and outs of market efficiency, it’s important for us to understand what an efficient market really means. In 1970 Eugene Fama, an American Economist based at the University of Chicago, published his work, ‘Efficient Capital Markets’. Here, Fama introduced the Efficient Market Hypothesis, explaining how all new information that may influence the price of a stock is already reflected in its price. Therefore, he argues that it is impossible for one to outperform the market through the analysis of individual stocks, because everything that is there for you to find out about a stock has already been implemented into its pricing.
Fama identified three subsets to his hypothesis. Firstly, there is the weak form of the Efficient Market Hypothesis. This is where the price of the stock is believed to reflect all currently available historical market data. Therefore, technical analysis has no place, as it relies on predicting stock prices based off historical trends, but this has already been implemented into the pricing and so you cannot make further predictions. However, in this form, fundamental analysis could still be useful, as it implies that it is possible for one to have belief in companies based on their fundamentals, and so predict their future returns. Essentially, the weak form is the most lenient form of the Efficient Market Hypothesis, because although is shows how technical analysis of chart patterns and historical data will not help you to predict how a stock price will change, it does allow room for fundamental analysis to take place.
Secondly, there is a semi-strong form, where the stock price is quickly adjusted based off new, publicly available information. Here, fundamental analysis has no impact as the price of the stocks do match the true value of the company the stock represents. As fundamental analysis assumes the opposite (that the market price of a stock doesn’t always match the true value of the company that the stock represents), it cannot be used to predict
changes in the price of stocks. Technical analysis also has no place this form, as this publicly available data includes the historical data of the stock, and so it cannot be useful for the same reasons for which it cannot be used in the weak form. At this form of the Efficient Market hypothesis, insider trading can still occur, as only the publicly available information has been incorporated into the price, therefore those who know private information could still outperform the market illegally.
Thirdly, there is a strong form. This is when the stock price reflects all information both public and private, and that no investor can beat the broader stock market on a risk adjusted basis over the long run. The risk adjusted basis theory states that if an investor is willing to take more risk, they can achieve higher returns. It also states that these higher risk investors should be compensated for the additional risk that they took. Therefore, in the strong form, it is impossible for somebody to predict the movement of the price of a stock through analysing it in anyway, even with information that they shouldn’t possess.
The strong form of the Efficient Market Hypothesis implies that markets are fully efficient. There are many arguments for whether this is the case and not, and these will be explored further in this article. In short, the weak form of the Efficient Market Hypothesis is likely true, as in practice, it seems that investors cannot beat the market using only historical data to forecast future price movements. The semi-strong form does cause a debate; however, history has proven that value investing as determined by the P/E ratio of a stock has on average outperformed the market in the long run. This proves that fundamental analysis and spending time to calculate the fair value of a stock does lead to higher potential returns. There are arguments for which this is not the case, however they are mostly insignificant.
To begin with, I will explain why the Efficient Market Hypothesis was (and to a lesser extent, is) so widely accepted after Eugene Fama’s ‘Efficient Capital Markets’ publication. A well-known story tells of a finance professor and a student walking through New York, and they came across a $100 bill lying on the ground. As the student stops to pick it up, the professor says, “Don’t bother, if it were really a $100 bill, it wouldn’t be there.” This story well illustrates what financial economists usually mean when they say that markets are efficient.
Fluck, Malkiel and Quandt (1997) simulated a strategy of
the very strong statistical evidence of return reversals (a phenomenon where stocks with relatively high returns over the past month or week earn negative abnormal returns the following month or week, while stocks with relatively low returns earn positive abnormal returns). With this information, one can assume that markets are not efficient, as return reversals seem predictable, and so investors could be able to capitalise on low returning stocks assuming that return reversals will be apparent. However, they also found that returns in the next period were similar for both groups, so they could not confirm that a contrarian approach would yield higher-thanaverage returns. There was a statistically strong pattern
is widely considered to be a way in which predictions can be made. Fama and French (1988) and Campbell and Shiller (1988) conducted statistical tests on the ability of dividend yields to forecast future returns. They measured the dividend yield of the broad U.S. stock market Standard & Poor's 500 Stock Index each quarter since 1926, and then calculated the market's subsequent ten-year total return through the year 2001. The observations were then divided into deciles depending upon the level of the initial dividend yield. In general, the exhibit shows that investors have earned a higher rate of return from the stock market when they purchased a market basket of equities with an initial dividend yield that was relatively high, and relatively low future rates of return when stocks were purchased at low dividend yields. Again, this implies that the market is inefficient as future stock prices can be predicted by dividend yields. However, these findings are actually not inconsistent with efficiency. This is because dividend yields on stocks are higher when interest rates are high, and low when interest rates are low. Therefore, this prediction of returns may just reflect how the stock market reacts in certain economic conditions. Furthermore, since the mid-1980s, the use of dividend yields to predict future returns has been ineffective. Dividend yields have been at the 3 percent level or below continuously since the mid-1980s, indicating very low forecasted returns. In fact, for all ten-year periods from 1985 through 1992 that ended June 30, 2002, realized annual equity returns from the market index have averaged over 15 percent. This could be because dividend behaviour of US corporations has changed over time. Companies in the 21st century have been more likely to institute a share repurchase program than to increase their dividends. So, dividend yield may not be as meaningful as it was in the past to predict future returns, and it wasn’t even necessarily any means to predict returns then. However, for a long time before the
mid-1980s, strategies such as the ‘Dogs of the Dow’, buying 10 stocks from the Dow Jones industrial average with the highest dividend yields, consistently outpaced the overall average. Mutual funds were set up implementing this strategy, and such funds significantly underperformed the market averages between 1995 and 1999.
So far, we have seen reason for which markets could be considered efficient. However, the success of value investors such as Warren Buffet will always be difficult to explain for those who assume market efficiency. Several studies suggest that "value" stocks have higher returns than so-called "growth" stocks. The most common two methods of identifying value stocks have been price-earnings ratios and price-to-book-value ratios. Stocks with low Price/Earnings ratios appear to provide higher rates of returns than stocks with high P/E ratios. This finding is consistent with the views of behaviouralists that investors tend to be overconfident of their ability to project high earnings growth, and thus overpay for "growth" stocks (for example, Kahneman
and Riepe, 1998). Therefore, we can create bubbles which will inevitably ‘burst’ once we realise that the amount we’re paying for the stocks is absurd. Eugene Fama does not believe in bubbles because if markets were efficient, stock prices would constantly represent their true value and could never be inflated.
Similar results have been shown for price/cash flow multiples, where cash flow is defined as earnings plus depreciation and amortization (Hawawini and Keim, 1995), and so proving the predictability of markets.
Moreover, the ratio of stock price to book value, defined as the value of a firm's assets minus its liabilities divided by the number of shares outstanding, has also been found to be a useful predictor of future returns. Fama and French (1993) concluded that size and price-to-book -value together provide considerable explanatory power for future returns, and once they are accounted for, little additional influence can be attributed to P/E multiples. Such results raise questions about the efficiency of the market if one accepts the capital asset pricing model (a model that helps to determine appropriate rates of return for assets based on their relative risk compared to the overall market), as Lakonishok, Shleifer and Vishny (1994) point out.
To conclude, there are many reasons for which there is a belief that the markets are efficient. For example, it has been proven that we cannot really predict future returns based off the dividend yield of stocks, or their previous performance. However, the fact that returns can be predicted through investor irrationality creating the likes of bubbles, and how there are methods for valuing stocks that have been proven to be successful, shows that markets are not strongly efficient at this point in time.
With this having been said, the reason for which I concluded with, ‘at this point in time’, is that this could change in the future. With the rise of Artificial Intelligence, the way markets behave could shift. If Artificial Intelligence is used widely to invest in the stock market, or let’s say all investment is conducted through Artificial Intelligence, this could alter the argument. This is because Artificial Intelligence will not be as irrational as humans. Therefore, it shouldn’t make the same mistakes that we do. It could potentially eliminate the
possibility of bubbles, as it would realise when prices are becoming too high for the true value of the product much earlier. There also wouldn’t be issues such as ticker confusion (a stock ticker is the 1 to 4 letter code that represents a stock), where due to promise in one stock, others with similar tickers also grow, as humans invest in the wrong one.
Artificial Intelligence has already significantly transformed stock market investing by providing powerful tools and insights. AI is extremely beneficial for stock picking, allowing investors to sort through vast amounts of data to identify stocks that meet their criteria. So, this has already eliminated an irrationality of investors, as humans like to come to quick conclusions and often take the first ‘good’ option, rather than sifting through every other possible option to see if there is one that is even better. Stock screeners also use AI to filter stocks based on fundamental and technical data points, such as accounting ratios, market capitalisation, trading volume and moving averages.
Artificial intelligence also plays a crucial role in algorithmic trading, enhancing the speed, accuracy and adaptability of trading systems. AI algorithms are able to quickly analyse vast amounts of market data, news feeds and other relevant information in real time to help traders make informed decisions. These algorithms can continuously learn and adapt over time, meaning that they will only become more efficient. Many hedge funds have used these qualities of AI to revolutionise their investment strategies through optimising portfolios, more tactical asset allocation, and generating new trading ideas. There are many more examples as to how Artificial Intelligence is already being used in the stock market which are already eliminating inefficiencies, and there is room for escalation.
Therefore, and to conclude once again, it is more likely that markets are currently not efficient. In the future, it will be interesting to see how dominant Artificial Intelligence becomes over the influence of the stock market, with its potential to eliminate the current inefficiencies.
Myles O, Year 12Since the inauguration of the internet in the 1990s, subsequent studies have found variations of the impact of distance on international trade. According to a study by Etemad, Wilkinson and Dana, distance is found to have a diminishing role in international trade as the internet arose. Technological advancements and trade are intrinsically intertwined. Technology has played a key role in altering the way nations trade, from the development of the wheel and the railroads to the introduction of containerisation. The last 200 years have seen a remarkable list of key technological advancements, with AI being the latest and one of the most notable. I believe the integration of AI will likely cause distance to have an even more diminishing role in international trade. AI will affect international trade in a plethora of ways. At the macroeconomic level, AI can increase output per unit of input, consequently increasing productivity growth. This leads to increased economic growth and provides new opportunities for international trade. Globally, rates of productivity growth are still low at the moment. Understanding the reasons behind this is crucial. AI doesn’t generate productivity gains immediately upon commissioning; one study suggests that the potential time lag in the productivity effect of AI may be attributed to adjustment costs and organisational changes. The rest of the article will try to shed more lights on this problem through exploration of specific AI applications in international trade, including supply chain management, translation systems and regulatory compliance.
AI facilitates the management and development of more proactive global supply chains. Data is vital to the success of supply chains from the very
beginning to the very end. This data encompasses information sourced from: online purchases (customer information, shipping addresses, payment methods), warehouse packing slips (item shipped, quantity, package dimensions) and shipment station goods scanning (tracks movement of goods). These datasets serve as inputs into an AI-driven logistics platform, enabling advanced decision-making that helps foresee problems in the supply chain and prepare solutions accordingly. The algorithm aims to account for changes in the nature of supply chain problems by continuously learning from new data inputs and adjusting their algorithms accordingly. In this way, the algorithm’s ability to adapt to evolving supply chains changes enable firms to anticipate consumer habits and adjust inventory levels, accordingly, thereby avoiding both under- and over-ordering. Consequently, by generating more accurate demand predictions, firms can optimise production and utilise the most cost-effective logistics solutions. This is evidenced in a 2022 McKinsey survey, where respondents identified that the highest cost
savings from AI are in supply chain management. AI could also revolutionise international commerce, a fact that is already becoming evident. International trade is significantly hindered by language barriers and AI has already begun to improve economic efficiency in this domain. Compared to human translators, machine translation systems can process large volumes of input text considerably faster and more cost-effectively. A study by Brynjolfsson examined the effects of implementing eBay’s Machine Translation (eMT) to facilitate online sales in foreign markets. The foundational rationale of eMT is to increase accessibility for consumers to search for and understand the details of items that are not listed in their language. The study’s findings revealed US exports to Spanish-speaking Latin American countries increased by 17.5% in terms of quantity and 13.1% in terms of revenue, attributable to eMT. To put this growth into context, a 10% reduction in distance between countries is correlated with a 3.51% increase in trade revenue of eBay. This implies a 13.1% increase in revenue due to eMT is equivalent to reducing the distance between countries by 37.3%, illustrating its diminishing role of distance in international trade. However, the level of adoption of AI-driven solutions like eMT may vary across different markets. In some countries, firms and consumers may be less receptive to using technology, due to cultural factors and regulation. Currently, 35% of all global companies use AI in their business. As this adoption rate increases, more
companies will experience its benefits, potentially mirroring the success seen with eMT. AI can additionally help regulatory compliance and anti-money laundering efforts. Historically, detecting trade non-compliance was more of a guessing game; it was impossible to identify which items required the allocation of valuable time and human resources for investigation. Additionally, firms must continuously investigate customers, vendors, or partners who may be violating trade regulations. Keeping on top with the ever-changing nature of regulatory and compliance requirements may be a significant time drain for firms. A raft of new sanctions, export controls and regulatory updates were introduced last year, including more than 740,000 updates in the first eight months of 2023 alone. With AI, firms can automate these numerous tasks, reducing the time and resources required for compliance checks, in addition to antimoney laundering activities. However, limitations and challenges still exist; because of its currently high rate of false detection and false classification, it often needs human inspection. Nevertheless, AI still enables smoother international trade, irrespective of geographical distance.
In summary, these three specific AI applications to international trade, described above, contribute to minimising the role of distance. I would further draw to
the reader’s attention that distance is not some arbitrary unit of measurement, but the real measure of how long it takes to get from A to B. In the context of international trade, the time it takes for goods and information to move between locations is more relevant than just physical distance and this time taken can be substantially diminished by AI. In practice, however, there is a question whether this potential can indeed be realised. This is because national regulatory frameworks surrounding AI are major determinants of how AI-based products are traded. This is already evident as some of the largest US firms by market
capitalisation (Google, Facebook and Amazon) do not have access to the Chinese market due to regulation constraints in China. Likewise, some of the largest Chinese firms by market capitalisation (Tencent and Alibaba) could also be excluded from the US market based on national security concerns.
AI, its Rapid Progression, and it’s Current Unequal Impact on the Labour Market
The rapid evolution of AI over the past couple of years has had many effects on an already volatile labour market due to complications with COVID 19. Whilst AI’s current capabilities may be somewhat limited, it already possesses the attributes necessary to complete simple tasks, such as quality control, documentation classification, and data logging. Companies which require these tasks to be carried out have already reportedly begun using AI. According to a study by Tech.co, “AI tools have extensively removed the need for numerous job rules” in numerous organizations engaging in the aforementioned tasks.
AI is primarily used in these organizations due to one of two attributes; the increased efficiency AI could provide (it can work more hours, and in most cases, more accurately and faster than human workers), and the lower cost of using AI. The primary goal of many businesses is the maximisation of profit, therefore decreasing the costs of creating a good or providing a service via paying for AI instead of hiring a worker would be within the best interests of any profit-driven business.
According to an article by techradarTM, the baseline compute cost to run a single NVIDIA A100 GPU (a device used to train AI) is only $1.50 per hour. Companies can then use the AI, trained specifically to be efficient at its task, to carry out the task required of it, costing them
only the figure used to power the computers on which this AI is ran. For human workers, however, the companies would have to hire someone to train them, which would cost at least the minimum wage (£10.42 in the UK for workers 23 and over as of 02/03/2024, although this figure is likely to encompass workers of age 21 and over as of 01/04/2024). These workers are then hired for the same hourly wage, which is much greater than the costs of using AI.
Greater efficiency for a lower cost would be appealing to almost all companies, so you might wonder how AI hasn’t completely overrun the labour market already. Despite AI massive upsides, it has one very limiting factor – its current diminished range of capabilities. Whilst AI would be very prolific at performing certain tasks, it could not perform others, such as the role of a doctor. Currently in fact, AI is limited to menial tasks such as data entry, and perhaps with access to a physical form (i.e. robots or machinery) it can perform some manual labour (such as in China, where 10% of the manufacturing industry reportedly consists of robot workers).
As we can see, AI already has an unequal effect on the labour market – whilst lower-paid manufacturing jobs and menial tasks are being replaced by cheaper AI workers, higher skilled and higher paid workers are much harder to replace, due to AIs current lack of higher
skilled capabilities. This means lower-skilled workers with a lower salary than average is now being contested by AI for their jobs, meaning they could be at risk from unemployment. This exacerbates the already large gap between the higher and lower skilled sectors of work, and results in it being harder for lower-skilled workers to find a job. Unfortunately, this consequence of the evolution of AI will only worsen in the foreseeable future, as AI will inevitably become the focal point for technological development, resulting in it evolving and increasing in efficiency at a greater rate. Unfortunately, the human workers are unlikely to contend with the increased proficiency of AI in the manufacturing industry, and would be let off, whilst the richer business owners enjoy a more profitable business due to more efficient workers and lower costs of production. This would only serve to worsen inequality, as we see the richer getting rich, whilst workers on a lower salary could be fired.
Whilst it would be impossible to predict the exact course of evolution AI will undergo, large investments into the new technology from massive companies such as Meta and NVIDIA are very likely to further its growth. Unfortunately for most workers, this means that their jobs could be in jeopardy very soon. Jobs involved in the
manufacturing industry are most likely to undergo this cleansing process first. Reportedly, the manufacturing industry in China is predicted to consist of 20% robot workers by 2025. Samsung have been paid $16.75 million by the South Korean Government to create robots for their manufacturing industry, and this concept is projected to be commonplace amongst governments in the future.
All this investment into AI and greater technology to perform labour would only worsen the inequality in the labour market. As lower-skilled workers lose their jobs to the more efficient and cheaper AI workers, inequality is only exacerbated, as the higher-paid workers are less likely to lose their jobs, due to the higher-skilled nature of their work, which would be tricky for AI to replicate, at least soon. As AI progresses, more jobs in the tech industry would be created, more specifically in the AI industry, due to the expansion in the market for AI (as more firms would incorporate AI into their company to further their profit margins). These new jobs would likely be within the bounds of creation and monitoring of AI, which would undoubtedly be high-skilled and well paid. Therefore, AI is likely to create more high-skilled jobs and reduce the amount of low-skilled labour on the market, increasing inequality in the labour market. This could also have effects on the unemployment rate, which would undoubtedly consist primarily of these low-skilled primary and secondary sector labourers, who cannot get a higher-skilled job as they lack the skills. This form of unemployment is called structural unemployment, and is commonplace during times of great technological advancement, such as this period.
some aspects of work, and as it evolves, it will come to be more efficient, and will extend its grasp on the labour market to other areas. Large tech firms are investing in the development of AI, and it is possible that we see a world where AI controls the entire labour market. It is already very proficient in manual labour, as seen by its domination in the Chinese manufacturing industry, and it
can be used to carry out simple tasks. As AI’s capabilities progress through investment and advances in technology, it will be able to take on more complicated tasks and will eventually be capable of doing anything a human can, therefore in the longer term, it is likely that markets will be completely overran by AI. This would help to reduce inequality, as AI would not discriminate based on the jobs it takes, as higher and lower earners see themselves put out of a job. However, this is theoretical, based mainly on the current trajectory of AI evolution, and so it is only one of many possibilities.
Therefore, in the future, AI is likely to widen the gap in the labour market between higher and lower skilled workers, increasing the inequality in this market.
In the long term, AI is very likely to dominate the labour market. It has already proven itself as a useful tool in
Rory W, Year 12Artificial Intelligence is an ever-growing industry paving the way for technological innovation, digital assistance, cybersecurity and more. This industry has undeniably found usage in all areas of society. The US international trade administration currently estimates the value of the industry at £16.9 billion and projects it to grow to £803.7 billion by 2035. As AI’s utility and usage increases, it is important we don't forget the often unnoticed environmental impacts of AI development and consumption.
The production of AI hardware is a costly process to the environment: it requires the extraction of rare earth metals alongside its assembly and distribution. Despite this, the production of AI hardware is generally considered to be relatively insignificant in terms of environmental impacts as extraction processes can be extremely efficient. However, there is still a negative externality of production as the extraction of resources
could lead to the destruction of widespread ecological habitats.
Energy usage by data centres has remained at a steady percentage of total energy consumption. However, the introduction of AI servers has rapidly increased their energy usage. Scientific American estimates that NVIDIA's planned shipping of over 1.5 million AI servers could require at least 85.4 terawatt-hours of electricity annually. Using government estimates for CO2 emissions when generating power, this would produce around 44,500 tonnes of CO2. This is only one projection of company energy consumption, and there will likely be much larger and more demanding projects in the future.
AI can also require substantial amounts of water for cooling. Google is currently planning a new data centre in Uruguay which would require 7.6 million litres of water each day, potentially diminishing water supplies for locals. However, this water doesn’t simply evaporate after usage for cooling and can still be used by others. Additionally, AI can play a crucial role in optimising
water distribution systems, ensuring that water is allocated as efficiently as possible. This is achieved through advanced algorithms which use predictive analytics, using a wide array of data from sensors and metres to identify leaks, detect anomalies and optimise the water flow. By reducing water losses, these predictive analysis models can easily make up for the water it uses and save far greater amounts.
AI seemingly has a negative externality of consumption and production. However, there are many uses for AI which can positively impact the environment. AI can provide many insights surrounding increasing efficiency, where companies like Intel and GE renewables are already utilising AI for significant CO2 savings. Analysis done by Fortune states that 'AI-enhanced manufacturing could reduce greenhouse gas emissions by 10-20%.'
Energy usage by AI is a controversial topic. It can be perceived that AI uses large amounts of energy for seemingly zero benefit. Contrarily, many others justify the energy consumption through the utility AI can provide. For example, machine learning algorithms play a pivotal role in optimisation, like the AI used for water systems. Furthermore, AI is used in a variety of energy sectors, including power generation, transmission and the usage of the servers. Using historical data and weather forecasts these models can predict when
renewable energy will be generating the most, thus minimising the necessity for fossil fuel-based power generation. This is essential for reducing the CO2 emitted via power generation whilst maintaining the needs of the population, clearly demonstrating one of the positive externalities of AI.
As the use of AI becomes increasingly prevalent in every aspect of daily life, the negative externalities of AI will consequently increase. To mitigate the harmful consequences created, the Government could introduce policies to regulate AI, ensuring a sustainable and safer future. For example, the Government could create policies encouraging the usage of renewable power generation to meet AI’s increased energy demand as well as passing legislation to limit AI’s use. Not only can the Government help in reducing the negative externalities, but we can also limit our own personal use of AI to reduce strain on the often-crowded servers. Establishing regulation will create a place for both technological innovation and environmental sustainability, leading to a superior, more productive and prosperous economy.
Elliot M, Year 12Personal finances is a broad term that covers money management and how people choose to spend their own money. Essentially, personal finances are defined by every financial action that an individual takes, from budgeting and spending with credit to mortgage and retirement planning.
Personal financing is paramount in living a sustainable lifestyle, however not enough people do it. According to the Financial Conduct Authority’s Financial Lives survey, 12.8 million adults in the UK are falling behind on bills
or finding their repayments a heavy burden. This statistic shows the worrying fact that personal finances are likely being ignored and people do not know how to control them. Furthermore, an OppLoans survey showed that 73% of Americans don’t have a budget that they regularly follow.
One reason explaining this behaviour is that some people act irresponsibly, making emotionally driven decisions with their money rather than spending responsibly. This is all summed up in one concept - animal spirits. John Maynard Keynes created this idea in his 1936 book “The General Theory of Employment, Interests and Money”,
using the concept to describe how people’s decisions, especially in times of volatility, are irrational and driven by emotions. For instance, impulse buying, overconfidence and taking up too much risk are behaviours driven by irrational and emotion driven decisions which negatively impact personal finances. Another reason explaining the absence of personal finances could be a lack of education. Personal finances can be difficult, especially when people already living busy lives have to count their spending, savings and create a plan for themselves. Many people simply do not know how to measure their money or don’t know where to start. A joint study from Compare the Market and MyBnk found that 61% of young adults did not remember having received financial education in school whilst only 41% of adults were deemed financially literate. A trend can be seen here - money management is an issue for the majority of people.
Whilst there are various solutions to helping people with their personal finances, for instance through education, there is one clear solution which stands out above all others - Artificial Intelligence. AI has the capabilities to provide advice, automation and security to ensure people manage their money in the best ways possible. As AI improves, its capabilities will only get better,
helping people further with their personal finances.
Automation can be a huge help when it comes to expense tracking and budgeting. AI-powered budgeting apps already help individuals track their spending. AI would analyse people’s expenses and do all the hard work counting, ensuring people are well informed of their spending. AI can help through providing advice and creating budget plans for the future. For instance, AI could track how much people have spent whilst keeping track of their income, taxes and expenses, providing people with a plan to follow so they can use their money sustainably without finding themselves in financial difficulty. Automation would also be able to create personalised plans, based on previous spending habits allowing people to carry on living the same lifestyle but without risky financial decisions.
AI also shines in automating the investment and wealth management process. Robo-advisors and automated investments use complex metrics and mathematical models to trade on behalf of a client. Automated investing is especially helpful as it takes the guesswork out of investing. Put simply, it is very difficult to tell if
the company is going to grow or not without in-depth analysis. People are able to select their ethical preferences, risk levels and desired return and then just let AI trade for them without having to worry about choosing the right stock. Automated investing is able to quickly analyse all options and buy using the investors’ money whilst robo-advisors advise investors on what to purchase, not necessarily buying it for them. Automated investment has already made returns of 2-5% in five years according to the Condor Capital Wealth Management Robo Report from 2023, indicating AI’s profound success despite the pandemic and its very early state. AI is improving rapidly and automated investing will only become more and more profitable with time.
AI can be a really useful tool in detecting frauds and keeping people’s money secure. AI can track personal behaviour and spending habits and is able to detect
when abnormal activities occur, shutting down the account or preventing any more spending. Having AI detect suspicious activity is relieving for people, allowing them to feel safer with their spending and futures. Personal finances are pivotal to live a sustainable life. Without good personal finances, it is nearly impossible to follow healthy spending routines and not find yourself in financial difficulty. However, many people find it challenging to control their personal finances due to emotionally driven choices and a lack of financial literacy. AI could be a useful tool to those who do not have the time for personal finances or the relevant knowledge. AI can achieve this by counting spending, providing advice and creating plans for the future, using wealth management and automated investments, all whilst keeping people’s money safe through fraud detection and AI-powered security.
Octavian M, Year 12In this new age of technological advancement AI has transformed the way we live, work and interact with the world around us. From self-driving cars to personalised systems, AI has permeated into nearly every aspect of our lives to improve productivity and efficiency. However, as AI continues to grow there are various ethical considerations and challenges that demand careful attention, so this promise for a more efficient future doesn’t cause societal harm.
At the heart of the discussion around AI is the balance between innovation and responsibility to make sure people benefit from this new technology and don’t end up getting left behind. However, AI brings with it many concerns such as its bias and fairness, privacy and accountability and lack of human autonomy for the future which must be weighed up with the economic growth, international competitiveness and increased productivity.
One of the most pressing ethical considerations of the adoption of AI is the issue of bias and fairness. AI systems are only as good as the data they are trained with, and this data has been known to be too narrow which can lead to bias. This can lead to unfair outcomes and system inequalities. An example of this is when AI is used in the hiring process to screen people for jobs with world leading firms such as Amazon even facing these challenges. The Amazon AI screening tool was found to discriminate against women and the system even started to penalise CVs which included the word ‘women’. This shows that AI can have huge ethical flaws as it will discriminate against certain demographics which can lead to unfairness and will continue to exacerbate disparities in firms.
Privacy and accountability are also critical ethical considerations that come with the adoption of AI. Many AI systems make it difficult to understand how they arrive to certain decisions. This complete lack of transparency makes it difficult to trust
AI systems, but it also imposes the question of who is accountable when AI makes an error. If a self-driving car crashes, who’s fault is it?
Privacy concerns also hide in the shadows of the adoption of AI, as AI requires widespread collection and analysis of personal data. This use of personal data raises concerns of potential unauthorised access and the misuse or exploitation of personal information. Therefore, protecting individuals’ rights is the only way of maintaining public trust and unlocking the full potential of AI technologies.
Moreover, the growing use of AI has large implications on human autonomy. As AI continues to permeate into every aspect of our lives it can start to take over in many decision-making processes. A good example of this this targeted advertisement and personalized recommendations used on platform like Netflix. These targeted images we see can lead to a narrowing of people’s view and can inadvertently restrict user’s autonomy. This therefore creates the risk of diminishing human control over certain aspects of our lives. It is paramount that we strike a balance between the efficiency and productivity gains of AI and the preservation of human autonomy in decision making which can affect many of the population.
Addressing these ethical issues will require a variety of different policies and regulations but it must be done to maintain a touch of reality in this fast-paced industry. Fostering a culture for responsible innovation, transparency and accountability is truly essential for the long-term success of AI and making sure that the collective interests of humanity are considered when making decisions about the adoption of AI into more industries.
Overall, ethics in the age of artificial intelligence is a real practical concern that demands attention and action from policy makers. By hitting that balance between growth and responsibility, we can harness the powers of AI for the benefit of all and transform the way we work. However, this idealistic future can only be achieved through collaboration and ethical reflection to navigate these issues for sustainable innovation that can benefit the whole of society.
The challenges that policymakers are often faced with are too little time and too much information. This was especially important during the COVID-19 pandemic which notoriously put government policymakers under significant time pressure. Could AI be the solution to this? Artificial intelligence is being integrated into every part of society, including government decision making and policymaking. This article will explore how AI can be used to enhance the policymaking process. Policymaking is a cyclical six-stage process of agenda setting, formulation, decision making, implementation and evaluation. At each stage, AI can help policymakers create more impactful and effective policies.
Agenda setting is the identification and recognition of a public problem. AI can rapidly analyse large datasets and crowdsourced data to identify critical issues. Machine Learning in particular can generate analysis in near real time so that leaders can take swift action. This allows policymakers to allocate resources to the most deprived causes, so the action has the largest social benefit. For example, in Australia, the Victoria State Government created a “syndromic surveillance” program which analyses public health data on patient symptoms, characteristics and triage, which is a procedure for prioritising assistance in the event of insufficient resources. This has identified public health risks and unusual patterns of illness. Within four months of usage, the program identified six public health concerns and has allowed public health officials to provide early warnings of potentially harmful illnesses. This will be especially useful for future epidemics and pandemics.
Formulation is the creation of strategies to address the problem at hand. AI can provide estimates of the impact, costs and benefits of different policy options and the impact on different geographical regions. The United Nations have developed models that predict the impact of trade policies on global trade flows. The United Nations Conference on Trade and Development research found that this model was superior to the dynamic factor model, a traditional econometric forecasting model, when predicting global merchandise and service
export values and volumes. A framework, named the “AI Economist” has been effective when assessing the impact of tax policy designs and formulating economic policies.
At this stage, policymakers formally adopt and legitimise a policy solution. This often involves commissioning studies and site visits, finalizing regulation and passing legislation. AI can improve the scheduling of hearings and votes, analyse bills, amendments and laws so that the process of legitimising policy is more efficient. A study by Deloitte’s AI institute found that 60% of investments in government AI will have a direct impact on real-time operational decisions by 2024.
Policy Implementation is the execution of the adopted policy as specified by the legislation. Through automation, real-time data processing and analysis, AI can improve the quality, speed and efficiency of policy implementation. In New Orleans, Louisiana, an emergency medical service agency utilised AI to maximise the placement of ambulances to where they were needed the most with particular focus on equitable access to services across all communities.
This is the assessment of how successful the policy is at addressing the issue and reaching objectives. With the use of extensive data, AI enables rapid analysis of the effects of the policy. For example, machine learning algorithms have been employed in the UK to assess the effectiveness of a carbon tax. Normally, this assessment is difficult due to the absence of a “counterfactual” which is the knowledge of what would’ve happened without the policy. The AI model is
able to predict outcomes with and without the carbon tax resulting in more accurate and impartial estimates of impacts which helps to continually optimize tax rates to reduce emissions. The applications of AI in policymaking are summarised in the diagram.
A key barrier to implementing AI in policymaking is building public trust. Concepts of fairness, justice and equity require in policymaking are inherently human, and therefore people are wary of the safety and fairness of AI. This is especially relevant in policymaking because it is difficult to avoid the impacts of policy whereas it is easier for consumers to opt out of commercial AI applications.
Another issue is AI’s inability to make sense of human reality – it struggles to understand unwritten ‘rules’ that an average person would be able to comprehend
because each ‘rule’ has exceptions that aren’t necessarily rational. The Cyc database project, which aims to give AI ‘common sense’, has coded close to 25 million nuances since 2017 and this wasn’t sufficient for AI to have the common sense of an average person.
Unsupervised AI can lead to unintended or even harmful consequences. For example, tax authorities in the Netherlands used a self-learning algorithm to identify fraudsters obtaining childcare benefits. Problems such as institutional biases, lack of transparency and inadequate checks caused the algorithm to mistake legitimate beneficiaries as potential fraudsters leading to expensive legal action, for those who could barely afford it. Amnesty International reported that having Turkish or Moroccan ethnicity led to a higher risk score and minor errors like a missing signature or late payments could result in misidentification as a potential fraudster. Cognitive biases and lack of understanding of human reality are a big problem in AI. The ‘fraudsters’ had their benefits suspended and had to repay previously used benefits immediately as a lump sum which caused tens of thousands of families to go into debt for years, pushing those who already required childcare benefits into poverty.
Another risk is found in data collection for AI because there are infrastructure and financial limitations. A significant capital investment is necessary for developers to obtain the proper resources to generate, collect, store and maintain data. This limits access to the data. This could be monopolised to firms with the financial capacity and resources. The State of AI report 2020 stated that only 15% of AI studies have shared their code and this leads to ethical concerns such as data collection methodologies which violate privacy.
Despite the many risks associated with AI usage, it will be an important tool in future economic policymaking to improve the efficiency of the process and the impact and quality of policy. This means that there will be more efficient resource allocation across nations. However, AI will not negate the need for human capital because it’s difficult for AI to make sense of human reality and societal nuances. To ensure the successful implementation of AI, human supervision and meticulous methodology are necessary.
What is risk and how does it affect financial markets?
Risk is a term in Economics that has many different meanings and applications - it is a crucial factor, especially in financial markets, that determines when people buy or sell securities.
The main issue is that we never know whether the value of a stock will go up or down; riskier stocks will usually be more volatile. The main type of risk that we will be looking at is endogenous risk- this is risk which has originated from inside of the financial sector and has spread throughout. This is the most dangerous type of risk as it usually is on a much larger scale and takes much longer to recover in contrast to exogenous risks (risk that started from outside financial sectors e.g. Covid19). Endogenous risk is what is measured when deciding the beta of a stock (the measure used for showing how volatile the stock is compared to the rest of the market).
If risk is too high this will deter people from buying shares in a company. This has negative consequences for the company as they have less money to reinvest making the company less competitive and consequently attracting less investment.
On the contrary, if risk seems incredibly low it can lead to lots of purchasing of the company’s stock which can greatly help the company expand and become more competitive compared to the rest of the market, stimulating more investment.
How has risk in financial markets been managed in the past?
Usually when banks and governments are measuring levels of risk in financial markets, they tend to focus in on recent events resulting in short term decisions. When they assume that the behaviour which they are taking part in is sustainable in the long run, there is a build-up of systemic risk.
An example of systemic risk is the 2008 Financial Crash. All the data which was used for forecasting was very recent only showing the good economic times, leading to the risk measurements in the financial markets being skewed. This meant that when the financial crash happened it was much worse than anyone could have expected, leaving people unprepared which worsened the impacts.
In addition, these financial decisions have also been driven by emotions. A major emotion which affects how we view risk in financial markets is fear. Fear controls whether we believe stock prices will go down, and this causes us to sell shares. Fear of missing out also leads us to invest irrationally in securities, in which we would not otherwise invest in. This can be much worse because the stock becomes very overvalued, forming a bubble. When people finally realise the true value (which can be seen with a P/E ratio), they become fearful of others also realising. This causes others to sell, leading to the price crashing and finally the bubble “pops”.
Firstly, AI is more likely to look at history as a whole, leaving the issue of looking at only the good economic times redundant. AI will also use complex algorithms,
enabling good financial decisions to be made without the bias a human would have.
AI can also be used to analyse substantial amounts of data in real-time, allowing for more accurate and timely risk assessments. This can help financial institutions to identify potential risks and take appropriate measures to mitigate them before they become a major issue. Additionally, AI can be used to develop predictive models that can forecast market trends and potential risks, allowing for more proactive risk management. Financial decisions would no longer be driven by fear and other emotions and would instead be driven by company value and opportunities in the market, a fairer way of allocating money and other resources.
Nevertheless, stock prices are still driven by human emotion, which are not accounted for by AI, although theoretically, it may be better if it will never be able to use human will and incentive in its decision making. In addition to this, if AI came across a new financial problem it would not know how to react, unlike a human.
While it can provide valuable insights and improve decision-making, it is still subject to the limitations of the data it is given and its learned algorithms. It is important that the data used by AI is accurate, unbiased, and that the algorithms are regularly reviewed to ensure efficiency.
In summary, AI will enhance risk management in financial markets, but will require human supervision or else the investment strategies will only be theoretical and not effective in the actual economy.
One of the ways that AI is being used to help manage risk in financial markets is by analysing large amounts of data from various sources, such as news, social media, market trends, and customer behaviour, helping to identify patterns, anomalies, and opportunities. AI can also use natural language processing and sentiment analysis to gauge the mood and expectations of investors and consumers, which can affect the demand and supply of financial products and services. However, as stated above, it cannot be fully efficient.
A 2019 survey by the Bank for International Settlements
revealed that 60% of banks were using AI and machine learning. The survey also showed that a majority of the time AI was being used for market oversight and supervision.
AI is also being used to help financial institutions to comply with regulations and prevent fraud, money laundering, and cyberattacks. By using AI to automate tasks such as data collection, reporting, and verification, financial firms can reduce the cost and time spent on these tasks and the accuracy which they are completed to. According to research done by Refinitiv in 2020,
74% of financial firms that use AI said that it had helped improve the accuracy and efficiency of their risk management processes.
The usage of AI continues to grow and so do its capabilities. Firms that do not make the most of it will fall behind, as they will not have the capability to be as efficient. Therefore, AI is and will continue to be an important part of firms managing risk in financial markets in the future.
Thomas N, Year 12Generative AI is evolving. More and more people are becoming increasingly dependent on the tool due to its time-saving genius with the additional benefit of less mental stimulation. However, as a result to its rapid growth, the cost of developing AI and training large
language models is rising stealthily because of its scalability.
Rising costs brings winners and losers. In this case, the big tech firms benefit from the costs increases due to raised barriers to entry into the AI market, enhancing
difficulty in forming and growing AI start-ups. In January this year, Microsoft announced the extending of their partnership with OpenAI, the creators of the chatbot ChatGPT which hosts over 175 million users. The partnership evidences the boost in funding required by OpenAI to 'advance cutting-edge AI research and democratize AI as a new technology platform' (Satya Nadella, Chairman and CEO of Microsoft).
Furthermore, rising costs leads makes it harder for universities and academic institutions to fund academic research into AI. This encourages scientists to move from academic research into more industry-based research. While leaving lower quality and quantity of AI research and teaching in academic institutions, AI research shifts towards primarily an industry job, changing the nature of the research to become more applied, investigative and conjectural. Research on the impact of academic research of research has shown that it has previously been a big contributor to many valuable innovations, and so closing this avenue with AI would cut off massive potentials for life-changing technology, given the value of AI services is at present.
Of course, there are many added bonuses that further incentivise professors to move into industry-based research. The most appealing is of course the greater wages available, but more importantly low funded universities may lack the resources and datasets available at big tech firms, which are needed for the necessary large language training for AI research. This flow of highly skilled and experienced AI talent paired with the wage and data incentives also disadvantages smaller firms in terms of keeping their current workers at their firms, also pushing job-seeking, inexperienced graduates towards industry rather than academia.
Several economists have coined the new term 'dataopolies' for the big tech firms that control huge amounts of data. They are oligopolies, but for data and information. An essay published on the AI Now Institute website mentioned that these 'data-opolies' may affect our 'privacy, autonomy, democracy and well-being', arguably making them more dangerous than traditional oligopolies (a market structure dominated by a few large firms, leading to interdependence and strategic interactions among competitors). They also mentioned that oligopolised AI may see its use more in 'public and private services, embedded systems and in key infrastructure'.
If we see oligopolised AI by big tech firms, then we may see a downward spiral in advertising and public mental health. Generative AI can be used to create ads that are appealing to the individual, tempting the user into clicking onto it, through the social media platforms owned by dominant tech firms. This generates ad revenue for firms through emotional and psychological appeal, which raises ethical considerations. As part of the personalised adverts, researchers are confident in the images generated may contain content that could stimulate or worsen mental health issues, whilst also drastically increasing consumer screen time. A recent study showed that 41% of the 180 health prompts used on 'popular AI tools' such as "OpenAI's ChatGPT, Google's Bard, and Snapchat's My AI" contained signs of harmful eating disorders.
In the political scope, the fact that most crucial commercial and communication services, including search and mapping engines, social media platforms, digital advertising systems, e-commerce platforms, email and operating systems, app stores and cloud computing has given the few corporations power in the decision making over the basis of inclusion of various consumers. Giving the tech firms free-roam control over AI infrastructure, arguably the most valuable of the services listed, would further intensify their position of control. This may extend to possible price discrimination and an increase in the terms and conditions of use of their sites, such as allowing the firms to use your data for their large language training models, as Zoom did in August 2023 for their users' voice and video data.
The AI landscape is changing once again, but not in the consumer direction. While generative AI begins to establish a prominent role in everyday life, it is coming at the expense of human health and power balances. This role is seeming to spill over to the structural changes in entire industries, steering the career paths of many aspiring and respected developers. What will really happen if AI is ruled by the few will not remain hypothetical for long.
Today, artificial intelligence is one of the fastest growing industries in the world; it has advanced to be one of the leading sectors in the world. As technology continues to advance, startups in this area represent a significant opportunity for entrepreneurs and can contribute to the economy if done well. The economic landscape of AI startups presents an abundance of challenges and risks for both the individual and the governments trying to support the growth of AI startups and its impact on the economy. This article will explore the key goals which must be met, when looking to bolster the growth of AI startups and the economy, in areas such as funding, labour markets, and international collaboration.
In supporting the growth of AI startups, the formation of dedicated investment funds and venture capital pools is essential for providing the funding and investment needed to boost startups into the competitive market. Large programmes by companies such as OpenAI and NVIDIA are notable examples of how this funding can be provided, with OpenAI providing 1M$ for every successful applicant, as well as both programmes providing opportunities for collaboration between successful applicants as well as the companies themselves. These programmes increase the competitiveness of AI startups in the market, allowing them to contribute to the economy. Furthermore, tax incentives and subsidies are a terrific way for the government to encourage R&D and growth in AI startups. The UK government’s R&D tax credit scheme, introduced as far back as 1981, aids new companies to spend on research and development. The increasing rate of tax relief given in this scheme in recent years, has seen a significant impact on new startups, with R&D rates increasing consistently by around 10% each year. However, it is important that both the government, and venture capital funds acknowledge the potential risks that these programmes hold. The diverse needs of AI startups makes it difficult for funding and investment to be offered effectively, and can result in an inefficient market, where money is being
used ineffectively. Additionally, fraud is a major problem for any tax scheme, and must be taken into consideration by the government, to prevent the R&D tax relief scheme from being taken advantage of.
The rapid growth of AI startups has contributed to the increase in highly skilled jobs demanded by employers in the labour market. To support the economics of AI startups, strategic investments in education and training in this sector are important. Allocating resources to educational programs with an AI focus, such as those already set up by various universities e.g. Stanford, MIT, Cambridge, and others, prepares graduates for entry into a highly skilled and competitive workforce. A program, supported by the OfS (UK), providing scholarships and opportunities for students across the UK to study Artificial Intelligence and Data Science at university, helps to tackle the enlarging skills gap present in the growing AI labour market. By enabling over 7400 students to enroll in university courses that provide students with the necessary skills to be competitive in the ever-changing labour market, the program is supporting the economic growth that AI startups contribute to and help in the nationwide transition to a more highly skilled workforce. Another issue that faces AI startups and their impact on the economy, is the focus on short-term prosperity over long-term sustainability. One way in which this can be visualized is through the turnover rate of employees. The period that employees spend at each company is quickly decreasing, getting as low as 6 months in the AI and Tech sector, as employees begin to take advantage of the plethora of job availabilities and leave at the slightest dissatisfaction. This may point to the fact that there should be a greater focus on talent retention, highlighting the need for the implementation of policies that not only attract but keep top job talent in the sector. Examples include work permits, streamlining visa processes, stock options, opportunities for career advancement, and much more. Improving this area of the AI Industry would have a
significant impact on the longevity and sustainability of AI startups, as they can instead choose to spend more resources and time on building a solid team, and target long-term growth, instead of chasing after talent in the labour market.
Another factor that significantly hinders the potential economic benefit that AI startups hold, is the lack of international collaboration. Xuli Tang, Xin Li, and Feicheng Ma (2022) found that international collaboration in AI, is much less prevalent than in other areas, with only 15.7% of businesses and research using international collaboration. International collaboration improves the quality and speed of research, as shown by Wagner (2017) who found that a higher number of countries represented in paper, positively correlated with higher citations. This, and other similar trends highlighting the correlations between the degree of international collaboration and the quality of research, highlight the importance of collaboration across borders. The technological revolution in ICT is often looked at as a force that ‘eradicated’ the issue of geographical distance. However, the ability of ICT to remove the need for close geographical distance is overestimated, with multiple studies being done proving that geographical distance in Europe is still a major hindering factor for collaboration. It is therefore important for both private companies and governments to contribute to the implementation of programs that support international partnerships. Initiatives like the Horizon Europe program, the world’s largest research collaboration program, are a fitting
example of how this can be implemented. Improving the framework that is available for AI startups to engage in these international partnerships is essential for the economic growth of both AI startups and the economy, allowing for much more efficient R&D, hastening the process of progress for these companies, and as a result, increasing the pace at which the positive effects of AI startups, such as contribution to the economy, increased job opportunities and technological advancement, occur.
In conclusion, the trajectory of Artificial intelligence startups holds immense promise for economic prosperity and technological advancement. However, this potential can only be realized through the concentrated efforts of governments and large companies to address the challenges that face these companies in the sector. Effective investment and funding strategies, Improvements in education and reduction in the digital skills gap, and supporting international collaboration are all key factors in supporting the growth of AI startups and ensuring long-term sustainability. Improvements in the research behind AI and increases in international partnerships will hold potential benefits for not just the individual companies, but on a global scale as well, by allowing countries and companies alike, to take advantage of the opportunity for improved productivity, effective methods, and technological advancements. By prioritizing these aims and taking note of the potential limitations and risks, policymakers and people in power can pave the way for AI startups to play an influential role in the economy and further societal progress.
In the realm of healthcare economics, the fusion of artificial intelligence (AI) is catalysing a paradigm shift, reshaping traditional methodologies, and unlocking unprecedented opportunities for efficiency and innovation. From optimizing resource allocation to enhancing patient care, AI is revolutionizing the way healthcare economics is understood and practiced.
One of the most pressing challenges in healthcare economics is the efficient allocation of resources to maximize patient outcomes while minimizing costs. AI plays a pivotal role in this endeavour by analysing vast amounts of data to identify patterns and trends. By leveraging machine learning algorithms, healthcare providers can optimize resource allocation, ensuring that critical resources such as medical equipment, staff, and facilities are deployed where they are most needed. This not only enhances operational efficiency but also improves patient access to quality care.
Predictive analytics powered by AI has emerged as a game-changer in healthcare economics. By analysing patient data, including medical history, diagnostic tests, and treatment outcomes, AI algorithms can forecast disease progression, identify at-risk patients, and anticipate healthcare needs with remarkable accuracy. This proactive approach enables healthcare providers to intervene early, potentially preventing costly
complications and reducing the overall burden on the healthcare system.
AI-driven technologies are revolutionizing patient care by enabling personalized treatment plans tailored to individual needs. By analysing vast datasets of patient demographics, genetic information, and clinical data, AI algorithms can identify optimal treatment strategies that are tailored to each patient's unique characteristics. This personalized approach not only improves patient outcomes but also reduces healthcare costs by minimizing unnecessary treatments and hospitalizations.
In addition to clinical applications, AI is transforming healthcare economics by streamlining administrative processes and reducing operational costs. AI-powered solutions automate routine tasks such as appointment scheduling, medical coding, and billing, freeing up valuable time for healthcare professionals to focus on patient care. Furthermore, AI algorithms can identify inefficiencies in healthcare delivery systems and suggest improvements to optimize resource utilization and reduce waste.
AI has the potential to address healthcare disparities by improving access to care and reducing barriers to
healthcare delivery. By analysing demographic data and healthcare utilization patterns, AI algorithms can identify underserved populations and target interventions to improve access to healthcare services. Furthermore, AIdriven telemedicine platforms enable remote consultations and monitoring, overcoming geographical barriers and increasing access to specialist care in rural and underserved areas.
While the integration of AI in healthcare economics offers immense potential, it also raises important ethical considerations. Issues such as data privacy, algorithmic bias, and accountability must be carefully addressed
to ensure that AI technologies are deployed in a fair and equitable manner. Moreover, healthcare providers must ensure that AI-driven decisionmaking processes are transparent and explainable, allowing for meaningful human oversight and intervention when necessary.
In conclusion, the integration of AI in healthcare economics holds tremendous promise for improving patient outcomes, enhancing operational efficiency, and reducing healthcare costs. By leveraging the power of AI, healthcare providers can unlock new insights, optimize resource allocation, and deliver personalized care that is tailored to the needs of individual patients. However, to fully realize the potential of AI in healthcare economics, it is essential to address ethical considerations and ensure that these technologies are deployed in a responsible and equitable manner.
Gates Cambridge Annual Lecture 2024: A global turning point: how to escape the permacrisis
Monday 22nd April, 5-6:45pm, online
Nobel Laureate Michael Spence and Dr Mohamed ElErian, President, Queens’ College will be joined by Gates Cambridge Scholar Reid Lidow to discuss their new book co-authored with former Prime Minister Gordon Brown. At the heart of today’s permacrisis are broken approaches to growth, economic management, and governance. While these approaches are broken, they are not beyond repair. "Permacrisis: A Plan to Fix a Fractured World" is an explanation of where we’ve gone wrong, and a provocative, inspiring plan to do nothing less than change the world. The authors set out how we can prevent crises and better manage the future for the benefit of the many and not the few. This is their answer to what change should look like.
More info: Click here or search on
Public Health Systems in the Age of Financialization
Tuesday 21st May, 5:30-7pm, online
In Public Health Systems in the Age of Financialization, Ana Carolina Cordilha unpacks policy shifts that have transformed public health systems into vehicles for financial speculation and capital accumulation. While it is commonly thought that these systems are being cut back in the period of financialization, the author shows that current changes in public health financing go far beyond budget cuts and privatization measures. She examines how public health systems are adopting financial instruments and participating in financial accumulation strategies, with harmful impacts on solidarity and democratic participation. With an in-depth study of both the French and Brazilian systems, Cordilha explores the different ways in which this process unfolds in central and peripheral countries.
More info: Click here or search on
The core mission of the Shackle Economics Society is to provide you with an opportunity to share your interest in Economics. Delivering a talk will also help you to hone your public speaking skills, grow in confidence, and enhance your university applications. We also invite external speakers to come and share their expertise. Recent external speakers include Maxwell Marlow (Adam Smith Institute), Christopher Snowdon (IEA) and Jeremy Featherstone.
If you are interested in presenting at the Shackle Economics Society, please contact Mr Featherstone or the new Shackle Society Representatives Myles O, Jack S and Enis A.
Four Perse teams took part in the Wharton Global High School Investment Competition this year and the Perse finals were held earlier this term. The teams presented their investment strategies to an audience including former Invesco fund manager Jeff Taylor, our outside judge. All teams put in an impressive performance, but Team NewittAll Investment Management managed to take the crown with their investment strategy for the client. The Perse will take part in next year's Wharton Global Competition again and teams will be decided by the end of the summer term. Up until then, PIC members are taking part in the Investopedia portfolio challenge and honing their knowledge of stock selection and investment strategies.
The Undercover Economist is a great Economics book explaining the hidden forces that shape our lives. Through engaging storytelling, Tim Harford presents difficult economic concepts in simple terms, giving day-to-day examples of how economic forces are at work in our quotidian actions. Ranging from why coffee shops are located where they are to delving into globalisation, the Undercover Economist helps people gain a well-rounded knowledge of Economics in a fun and informative manner. I would recommend the book for anyone who is interested in everyday applications of Economics as well as topics which are no longer taught at A Level such as game theory and auction theory.
Octavian M
From match fixing sumo wrestling to exposing the infamous Ku Klux Klan’s deepest secrets, Freakonomics is a thought-provoking and engaging book that challenges readers to look at the world through an economics lens. By considering the hidden forces that shape human behaviour and society, Steven Levitt and Stephen Dubner touch on incentives and motivation, conventional wisdom and unconventional thinking, causality vs correlation, and the general application of economics to form an interdisciplinary approach to introducing various economics concepts. I would recommend the book to anyone who is unsure where to start their super-curricular reading, but it is also great for those keen on behavioural economics, psychology and/or sociology.
Varun VBorrow a book and submit a review for the next edition.
Lords of Finance by Liaquat Ahamed provides a captivating narrative that delves into the world of banking and politics during the interwar period. We meet four central bankers who held the reigns for most of the global economy, where each decision has widespread lasting effects: Montagu Norman of the Bank of England, Benjamin strong of the Federal Reserve Bank, Hjalmar Schacht of the Reichsbank, and Emile Moreau of the Banque de France. Ahamed explores the political and economic events and decisions that led to the Great Depression, providing context for the consequences of monetary policy and the interplay between financial markets and geopolitics. I highly recommend this book to anyone who holds an interest in economics or politics and wants to develop an understanding of the events that shaped the modern economy.
Ivan KEconomic Persepectives needs articles and book reviews. It would be great to have more pupils contributing from all year groups. Why not pick one of the new books in the Economics Library and write a short review? Please get in touch with the editors for more information.
The Shackle Economics Society are always open to new contributions from pupils, even if you don’t study economics. Pick a topic of your choice and get in touch with Mr Featherstone or the student representatives.
Do you have an idea for a new society or event that you would like to lead? Pitch your idea to Mr Larner.