6 minute read
Abramoff’s Diagnostic Artificial Intelligence Tackles Diabetic Retinopathy
by uiowaeye
Next Article
When Michael Abramoff, MD, PhD , was an ophthalmology resident, he found himself spending a lot of his time with patients doing annual diabetic retinopathy exams, only to confirm they didn’t have the disease and telling them to come back in a year. Conversely, he’d also see patients who were already experiencing symptoms of diabetic retinopathy, but to effectively treat this disease and prevent blindness, it needs to be diagnosed before the patient begins experiencing symptoms. There needed to be a way to test for diabetic retinopathy that increased productivity for providers and increased access for patients.
“In my view, the central problems in healthcare—ever increasing cost, and persistent health inequity—is because of a productivity gap,” Abramoff said. In other fields outside of healthcare, Abramoff explained, productivity has been relentlessly increasing due to “innovations such as mechanization and automation.” Even with increases in technology, like digital health records, healthcare is still heavily dependent on physicians doing most of the same manual, diagnostic tasks that they have been doing for the better part of a century.
With a background in ophthalmology and computer science, Abramoff wasn’t satisfied with the status quo. “I theorized that we could automate healthcare tasks using technology, especially in the case of chronic diseases, such as image-based diagnosis, that would improve productivity,” Abramoff said. But he also saw the benefits of this type of automation for underserved populations. “Bringing care to where the patient already visits, such as primary care and retail or laboratory clinics rather than the patient coming to the care, such as a specialist’s office,” Abramoff explained, “would increase access for patients that may never have been seen otherwise.”
The idea of using machine learning to diagnose diseases wasn’t something that just occurred to Abramoff—he had been thinking of ways to create and use this technology for decades. In the 1980s he was designing machine learning algorithms using neural networks. Through his residency he began to see specific uses for, what is now called, “autonomous diagnostic artificial intelligence.” In his PhD work, Abramoff began laying the groundwork for diagnostic AI in healthcare and eventually he began a decade’s long process of working with the FDA and other regulators to “measure how ethical principles are being met by an AI, ensure safety, efficacy and equity, and mitigate racial and ethnic bias by designing AI the right way—clarifying what the best clinical trials are, and what AI should be compared to in order to prove its safety and efficacy.” The goal was the make sure this autonomous machine learning AI, which after all makes medical decisions by itself, has high safety and minimal racial and other undesired bias.
This work led to Abramoff inventing a general machine learning approach that uses so-called “disease biomarkers” to build biomarker specific detectors that in combination now have higher accuracy, avoid the introduction of racial bias, and can determine when any of the detectors are incorrect. This “ethical framework for the creation of AI in healthcare” was the foundation on which Abramoff would begin to build AI diagnostic devices that could autonomously diagnose diabetic retinopathy.
To meet his goals, Abramoff formed a company called Digital Diagnostics. Today the automated diabetic retinopathy diagnostic solution he had envisioned years ago is a fully developed device called IDx-DR. This new technology was authorized in 2018 by the FDA for use in clinical care. As a testament to the efficacy of the IDx-DR, it’s become part of the American Diabetes Association’s “Standard of Diabetes Care” to detect diabetic retinopathy and help prevent blindness. Just this year, Abramoff was awarded a U.S. patent for this new technology, which covers the “diagnosis of a disease condition using an automated diagnostic model.” This applies to any diagnostic device that uses AI to learn from example images, which applies well beyond eye care. Currently Abramoff’s diagnostic devices are being used in over 20 health care systems around the country. It feels safe to say that Abramoff has proven that intelligent diagnostic platforms can be deployed safely and responsibly to improve patient outcomes, while increasing healthcare productivity and patient access.
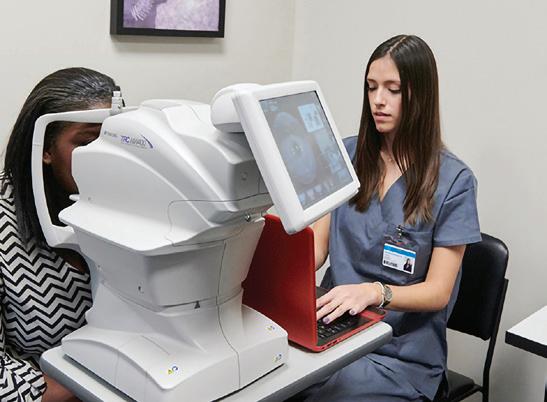
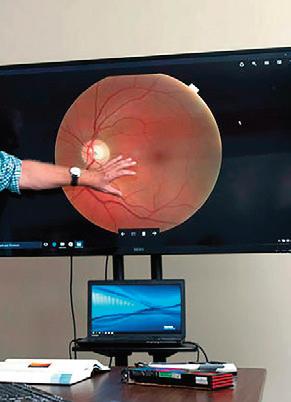
Most highly cited with University of Iowa first author:
M. D. Abràmoff, B. Cunningham, B. Patel, M. B. Eydelman, T. Leng, T. Sakamoto, B. Blodi, S. M. Grenon, R. M. Wolf, A. K. Manrai, J. M. Ko, M. F. Chiang, D. Char, M. Blumenkranz, E. Chew, M. Chiang, M. Eydelman, D. Myung, J. S. Schuman, C. Shields, K. A. Goldman, R. Wolf, J. L. Gassee, D. Roman, S. Satel, D. Fong, D. Rhew, H. Wei and M. Willingham. Foundational Considerations for Artificial Intelligence Using Ophthalmic Images. Ophthalmology 2022;129(2): e14-e32. doi: 10.1016/j.ophtha.2021.08.023
S. R. Russell, A. V. Drack, A. V. Cideciyan, S. G. Jacobson, B. P. Leroy, C. Van Cauwenbergh, A. C. Ho, A. V. Dumitrescu, I. C. Han, M. Martin, W. L. Pfeifer, E. H. Sohn, J. Walshire, A. V. Garafalo, A. K. Krishnan, C. A. Powers, A. Sumaroka, A. J. Roman, E. Vanhonsebrouck, E. Jones, F. Nerinckx, J. De Zaeytijd, R. W. J. Collin, C. Hoyng, P. Adamson, M. E. Cheetham, M. R. Schwartz, W. den Hollander, F. Asmus, G. Platenburg, D. Rodman and A. Girach. Intravitreal antisense oligonucleotide sepofarsen in Leber congenital amaurosis type 10: a phase 1b/2 trial. Nat Med 2022;28(5): 1014-1021. doi: 10.1038/s41591-022-01755-w
A. P. Voigt, N. K. Mullin, K. Mulfaul, L. P. Lozano, L. A. Wiley,
M. J. Flamme-Wiese, E. A. Boese, I. C. Han, T. E. Scheetz, E. M. Stone, B. A. Tucker and R. F. Mullins. Choroidal Endothelial and Macrophage Gene Expression in Atrophic and Neovascular Macular Degeneration. Hum Mol Genet 2022;31(14): 2406-2423. doi: 10.1093/hmg/ddac043
R. G. Coussa, E. M. Binkley, M. E. Wilkinson, J. L. Andorf, B. A. Tucker, R. F. Mullins, E. H. Sohn, L. A. Yannuzzi, E. M. Stone and I. C. Han. Predominance of hyperopia in autosomal dominant Best vitelliform macular dystrophy. Br J Ophthalmol 2022;106(4): 522527. doi: 10.1136/bjophthalmol-2020-317763
E. M. Binkley, M. R. Tamplin, A. H. Vitale, H. C. Boldt, R. H. Kardon and I. M. Grumbach. Longitudinal optical coherence tomography angiography (OCT-A) in a patient with radiation retinopathy following plaque brachytherapy for uveal melanoma. Am J Ophthalmol Case Rep 2022;26(101508). doi: 10.1016/j. ajoc.2022.101508
Most highly cited with any University of Iowa author:
M. D. Abràmoff, B. Cunningham, B. Patel, M. B. Eydelman, T. Leng, T. Sakamoto, B. Blodi, S. M. Grenon, R. M. Wolf, A. K. Manrai, J. M. Ko, M. F. Chiang, D. Char, M. Blumenkranz, E. Chew, M. Chiang, M. Eydelman, D. Myung, J. S. Schuman, C. Shields, K. A. Goldman, R. Wolf, J. L. Gassee, D. Roman, S. Satel, D. Fong, D. Rhew, H. Wei and M. Willingham. Foundational Considerations for Artificial Intelligence Using Ophthalmic Images. Ophthalmology 2022;129(2): e14-e32. doi: 10.1016/j.ophtha.2021.08.023
C. M. McDowell, K. Kizhatil, M. H. Elliott, D. R. Overby, J. van Batenburg-Sherwood, J. C. Millar, M. H. Kuehn, G. Zode, T. S. Acott, M. G. Anderson, S. K. Bhattacharya, J. A. Bertrand, T. Borras, D. E. Bovenkamp, L. Cheng, J. Danias, M. L. De Ieso, Y. Du, J. A. Faralli, R. Fuchshofer, P. S. Ganapathy, H. Gong, S. Herberg, H. Hernandez, P. Humphries, S. W. M. John, P. L. Kaufman, K. E. Keller, M. J. Kelley, R. A. Kelly, D. Krizaj, A. Kumar, B. C. Leonard, R. L. Lieberman, P. Liton, Y. Liu, K. C. Liu, N. N. Lopez, W. Mao, T. Mavlyutov, F. McDonnell, G. J. McLellan, P. Mzyk, A. Nartey, L. R. Pasquale, G. C. Patel, P. P. Pattabiraman, D. M. Peters, V. Raghunathan, P. V. Rao, N. Rayana, U. Raychaudhuri, E. Reina-Torres, R. Ren, D. Rhee, U. R. Chowdhury, J. R. Samples, E. G. Samples, N. Sharif, J. S. Schuman, V. C. Sheffield, C. H. Stevenson, A. Soundararajan, P. Subramanian, C. K. Sugali, Y. Sun, C. B. Toris, K. Y. Torrejon, A. Vahabikashi, J. A. Vranka, T. Wang, C. E. Willoughby, C. Xin, H. Yun, H. F. Zhang, M. P. Fautsch, E. R. Tamm, A. F. Clark, C. R. Ethier and W. D. Stamer. Consensus Recommendation for Mouse Models of Ocular Hypertension to Study Aqueous Humor Outflow and Its Mechanisms. Invest Ophthalmol Vis Sci 2022;63(2): 12. doi: 10.1167/iovs.63.2.12
K. M. Miller, T. A. Oetting, J. P. Tweeten, K. Carter, B. S. Lee, S. Lin, A. A. Nanji, N. H. Shorstein and D. C. Musch. Cataract in the Adult Eye Preferred Practice Pattern. Ophthalmology 2022;129(1): p1p126. doi: 10.1016/j.ophtha.2021.10.006
H. K. Gill, R. L. Niederer, E. M. Shriver, L. K. Gordon, A. L. Coleman and H. V. Danesh-Meyer. An Eye on Gender Equality: A Review of the Evolving Role and Representation of Women in Ophthalmology. Am J Ophthalmol 2022;236(232-240). doi: 10.1016/j.ajo.2021.07.006
B. Chandra, M. L. Tung, Y. Hsu, T. Scheetz and V. C. Sheffield Retinal ciliopathies through the lens of Bardet-Biedl Syndrome: Past, present and future. Prog Retin Eye Res 2022;89(101035). doi: 10.1016/j.preteyeres.2021.101035